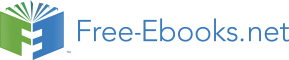

National Artificial Intelligence Research
and Development Strategic Plan
On May 3, 2016, the Obama Administration announced the formation of a new NSTC Subcommittee on Machine Learning and Artificial intelligence, to help coordinate Federal activity in AI. This Subcommittee, on June 15, 2016, directed the Subcommittee on Networking and Information Technology Research and Development (NITRD) to create a National Artificial Intelligence Research and Development Strategic Plan. A NITRD Task Force on Artificial Intelligence was then formed to define the Federal strategic priorities for AI R&D, with particular attention on areas that industry is unlikely to address.
This National Artificial Intelligence R&D Strategic Plan establishes a set of objectives for Federally-funded AI research, both research occurring within the government as well as Federally-funded research occurring outside of government, such as in academia. The ultimate goal of this research is to produce new AI knowledge and technologies that provide a range of positive benefits to society, while minimizing the negative impacts. To achieve this goal, this AI R&D Strategic Plan identifies the following priorities for Federally-funded AI research:
Strategy 1: Make long-term investments in AI research. Prioritize investments in the next generation of AI that will drive discovery and insight and enable the United States to remain a world leader in AI.
Strategy 2: Develop effective methods for human-AI collaboration. Rather than replace humans, most AI systems will collaborate with humans to achieve optimal performance. Research is needed to create effective interactions between humans and AI systems.
Strategy 3: Understand and address the ethical, legal, and societal implications of AI. We expect AI technologies to behave according to the formal and informal norms to which we hold our fellow humans. Research is needed to understand the ethical, legal, and social implications of AI, and to develop methods for designing AI systems that align with ethical, legal, and societal goals.
Strategy 4: Ensure the safety and security of AI systems. Before AI systems are in widespread use, assurance is needed that the systems will operate safely and securely, in a controlled, well-defined, and well-understood manner. Further progress in research is needed to address this challenge of creating AI systems that are reliable, dependable, and trustworthy.
Strategy 5: Develop shared public datasets and environments for AI training and testing. The depth, quality, and accuracy of training datasets and resources significantly affect AI performance. Researchers need to develop high quality datasets and environments and enable responsible access to high-quality datasets as well as to testing and training resources.
Strategy 6: Measure and evaluate AI technologies through standards and benchmarks. Essential to advancements in AI are standards, benchmarks, testbeds, and community engagement that guide and National Artificial Intelligence Research and Development Strategic Plan evaluate progress in AI. Additional research is needed to develop a broad spectrum of evaluative techniques to evaluate progress in AI. Additional research is needed to develop a broad spectrum of evaluative techniques.
Strategy 7: Better understand the national AI R&D workforce needs. Advances in AI will require a strong community of AI researchers. An improved understanding of current and future R&D workforce demands in AI is needed to help ensure that sufficient AI experts are available to address the strategic R&D areas outlined in this plan.
The AI R&D Strategic Plan closes with two recommendations:
Recommendation 1: Develop an AI R&D implementation framework to identify S&T opportunities and support effective coordination of AI R&D investments, consistent with Strategies 1-6 of this plan.
Recommendation 2: Study the national landscape for creating and sustaining a healthy AI R&D workforce, consistent with Strategy 7 of this plan.
This plan makes several assumptions about the future of AI. First, it assumes that AI technologies will continue to grow in sophistication and ubiquity, thanks to AI R&D investments by government and industry. Second, this plan assumes that the impact of AI on society will continue to increase, including on employment, education, public safety, and national security, as well as the impact on U.S. economic growth. Third, it assumes that industry investment in AI will continue to grow, as recent commercial successes have increased the perceived returns on investment in R&D. At the same time, this plan assumes that some important areas of research are unlikely to receive sufficient investment by industry, as they are subject to the typical underinvestment problem surrounding public goods. Lastly, this plan assumes that the demand for AI expertise will continue to grow within industry, academia, and government, leading to public and private workforce pressures.
Desired Outcome
This AI R&D Strategic Plan looks beyond near-term AI capabilities toward longer-term transformational impacts of AI on society and the world. Recent advances in AI have led to significant optimism about the potential for AI, resulting in strong industry growth and commercialization of AI approaches. However, while the Federal government can leverage industrial investments in AI, many application areas and long-term research challenges will not have clear near-term profit drivers, and thus may not be significantly addressed by industry. The Federal government is the primary source of funding for long-term, high-risk research initiatives, as well as near-term developmental work to achieve department- or agency-specific requirements or to address important societal issues that private industry does not pursue. The Federal government should therefore emphasize AI investments in areas of strong societal importance that are not aimed at consumer markets—areas such as AI for public health, urban systems and smart communities, social welfare, criminal justice, environmental sustainability, and national security, as well as long-term research that accelerates the production of AI knowledge and technologies.
A coordinated R&D effort in AI across the Federal government will increase the positive impact of these technologies, and provide policymakers with the knowledge needed to address complex policy challenges related to the use of AI. A coordinated approach, moreover, will help the United States capitalize on the full potential of AI technologies for the betterment of society.
This AI R&D Strategic Plan defines a high-level framework that can be used to identify scientific and technological gaps in AI and track the Federal R&D investments that are designed to fill those gaps. The AI R&D Strategic Plan identifies strategic priorities for both near-term and long-term support of AI that address important technical and societal challenges. The AI R&D Strategic Plan, however, does not define specific research agendas for individual Federal agencies. Instead, it sets objectives for the Executive Branch, within which agencies may pursue priorities consistent with their missions, capabilities, authorities, and budgets, so that the overall research portfolio is consistent with the AI R&D Strategic Plan.
AI can increase economic prosperity by the introduction of new products and services which can create new markets, and improve the quality and efficiency of existing goods and services across multiple industries including: Manufacturing, Logistics, Finance, Transportation, Agriculture, Marketing, Communications, and Science and Technology. AI can also improve educational opportunities and social wellbeing and enhanced national and homeland security.
Since its beginnings, AI research has advanced in three technology waves. The first wave focused on handcrafted knowledge, with a strong focus in the 1980s on rule-based expert systems in well-defined domains, in which knowledge was collected from a human expert, expressed in “if-then” rules, and then implemented in hardware. Such systems-enabled reasoning was applied successfully to narrowly defined problems, but it had no ability to learn or to deal with uncertainty. Nevertheless, they still led to important solutions, and the development of techniques that are still actively used.
The second wave of AI research from the 2000s to the present is characterized by the ascent of machine learning. The availability of significantly larger amounts of digital data, relatively inexpensive massively parallel computational capabilities, and improved learning techniques have brought significant advances in AI when applied to tasks such as image and writing recognition, speech understanding, and human language translation. The fruits of these advances are everywhere: smartphones perform speech recognition, ATMs perform handwriting recognition on written checks, email applications perform spam filtering, and free online services perform machine translation. Key to some of these successes was the development of deep learning.
AI systems now regularly outperform humans on specialized tasks. Major milestones when AI first surpassed human performance include: chess (1997), trivia (2011), Atari games (2013), image recognition (2015), speech recognition (2015), and Go (2016). The pace of such milestones appears to be increasing, as is the degree to which the best-performing systems are based on machine learning methods, rather than sets of hand-coded rules.
Such achievements in AI have been fueled by a strong base of fundamental research. This research is expanding and is likely to spur future advances. As one indicator, from 2013 to 2015 the number of Web of Science-indexed journal articles mentioning "deep learning" increased six-fold. The trends also reveal the increasingly global nature of research, with the United States no longer leading the world in publication numbers, or even publications receiving at least one citation.
The AI field is now in the beginning stages of a possible third wave, which focuses on explanatory and general AI technologies. The goals of these approaches are to enhance learned models with an explanation and correction interface, to clarify the basis for and reliability of outputs, to operate with a high degree of transparency, and to move beyond narrow AI to capabilities that can generalize across broader task domains. If successful, engineers could create systems that construct explanatory models for classes of real world phenomena, engage in natural communication with people, learn and reason as they encounter new tasks and situations, and solve novel problems by generalizing from past experience. Explanatory models for these AI systems might be constructed automatically through advanced methods. These models could enable rapid learning in AI systems. They may supply “meaning” or “understanding” to the AI system, which could then enable the AI systems to achieve more general capabilities.
The research priorities outlined in this AI R&D Strategic Plan focus on areas that industry is unlikely to address and thus areas that are most likely to benefit from Federal investment. These priorities cut across all of AI to include needs common to the AI sub-fields of perception, automated reasoning/planning, cognitive systems, machine learning, natural language processing, robotics, and related fields. Because of the breadth of AI, these priorities span the entire field, rather than only focusing on individual research challenges specific to each sub-domain. To implement the plan, detailed roadmaps should be developed that address the capability gaps consistent with the plan.
One of the most important Federal research priorities, outlined in Strategy 1, is for sustained long-term research in AI to drive discovery and insight. Many of the investments by the U.S. Federal government in high-risk, high-reward fundamental research have led to revolutionary technological advances we depend on today, including the Internet, GPS, smartphone speech recognition, heart monitors, solar panels, advanced batteries, cancer therapies, and much, much more. The promise of AI touches nearly every aspect of society and has the potential for significant positive societal and economic benefits. Thus, to maintain a world leadership position in this area, the United States must focus its investments on high-priority fundamental and long-term AI research.
Many AI technologies will work with and alongside humans, thus leading to important challenges in how to best create AI systems that work with people in intuitive and helpful ways. The walls between humans and AI systems are slowly beginning to erode, with AI systems augmenting and enhancing human capabilities. Fundamental research is needed to develop effective methods for human-AI interaction and collaboration, as outlined in Strategy 2.
AI advancements are providing many positive benefits to society and are increasing U.S. national competitiveness.8 However, as with most transformative technologies, AI presents some risks in several areas, from jobs and the economy to safety, ethical, and legal questions. Thus, as AI science and technology develop, the Federal government must also invest in research to better understand what the implications are for AI for all these realms, and to address these implications by developing AI systems that align with ethical, legal, and societal goals, as outlined in Strategy 3.
A critical gap in current AI technology is a lack of methodologies to ensure the safety and predictable performance of AI systems. Ensuring the safety of AI systems is a challenge because of the unusual complexity and evolving nature of these systems. Several research priorities address this safety challenge. First, Strategy 4 emphasizes the need for explainable and transparent systems that are trusted by their users, perform in a manner that is acceptable to the users, and can be guaranteed to act as the user intended.
The potential capabilities and complexity of AI systems, combined with the wealth of possible interactions with human users and the environment, makes it critically important to invest in research that increases the security and control of AI technologies. Strategy 5 calls on the Federal government to invest in shared public datasets for AI training and testing in order to advance the progress of AI research and to enable a more effective comparison of alternative solutions. Strategy 6 discusses how standards and benchmarks can focus R&D to define progress, close gaps, and drive innovative solutions for specific problems and challenges. Standards and benchmarks are essential for measuring and evaluating AI systems and ensuring that AI technologies meet critical objectives for functionality and interoperability.
Finally, the growing prevalence of AI technologies across all sectors of society creates new pressures for AI R&D experts. Opportunities abound for core AI scientists and engineers with a deep understanding of the technology who can generate new ideas for advancing the boundaries of knowledge in the field. The Nation should take action to ensure a sufficient pipeline of AI-capable talent. Strategy 7 addresses this challenge.
While the ultimate goal for many AI algorithms is to address open challenges with human-like solutions, we do not have a good understanding of what the theoretical capabilities and limitations are for AI and the extent to which such human-like solutions are even possible with AI algorithms. Theoretical work is needed to better understand why AI techniques—especially machine learning—often work well in practice.
While different disciplines (including mathematics, control sciences, and computer science) are studying this issue, the field currently lacks unified theoretical models or frameworks to understand AI system performance. Additional research is needed on computational solvability, which is an understanding of the classes of problems that AI algorithms are theoretically capable of solving, and likewise, those that they are not capable of solving. This understanding must be developed in the context of existing hardware, in order to see how the hardware affects the performance of these algorithms. Understanding which problems are theoretically unsolvable can lead researchers to develop approximate solutions to these problems, or even open up new lines of research on new hardware for AI systems. For example, when invented in the 1960s, Artificial Neural Networks (ANNs) could only be used to solve very simple problems. It only became feasible to use ANNs to solve complex problems after hardware improvements such as parallelization were made, and algorithms were adjusted to make use of the new hardware. Such developments were key factors in enabling today’s significant advances in deep learning.
General AI has been an ambition of researchers since the advent of AI, but current systems are still far from achieving this goal. The relationship between narrow and general AI is currently being explored; it is possible that lessons from one can be applied to improve the other and vice versa. While there is no general consensus, most AI researchers believe that general AI is still decades away, requiring a long-term, sustained research effort to achieve it.
However, groups and networks of AI systems may be coordinated or autonomously collaborate to perform tasks not possible with a single AI system, and may also include humans working alongside or leading the team. The development and use of such multi-AI systems creates significant research challenges in planning, coordination, control, and scalability of such systems. Planning techniques for multi-AI systems must be fast enough to operate and adapt in real time to changes in the environment. They should adapt in a fluid manner to changes in available communications bandwidth or system degradation and faults. Many prior efforts have focused on centralized planning and coordination techniques; however, these approaches are subject to single points of failure, such as the loss of the planner, or loss of the communications link to the planner. Distributed planning and control techniques are harder to achieve algorithmically, and are often less efficient and incomplete, but potentially offer greater robustness to single points of failure. Future research must discover more efficient, robust, and scalable techniques for planning, control, and collaboration of teams of multiple AI systems and humans.
Attaining human-like AI requires systems to explain themselves in ways that people can understand. This will result in a new generation of intelligent systems, such as intelligent tutoring systems and intelligent assistants that are effective in assisting people when performing their tasks. There is a significant gap, however, between the way current AI algorithms work and how people learn and perform tasks. People are capable of learning from just a few examples, or by receiving formal instruction and/or “hints” to performing tasks, or by observing other people performing those tasks. Medical schools take this approach, for example, when medical students learn by observing an established doctor performing a complex medical procedure. Even in high-performance tasks such as world-championship Go games, a master-level player would have played only a few thousand games to train him/herself. In contrast, it would take hundreds of years for a human to play the number of games needed to train AlphaGo. More foundational research on new approaches for achieving human-like AI would bring these systems closer to this goal.
Significant advances in robotic technologies over the last decade are leading to potential impacts in a multiplicity of applications, including manufacturing, logistics, medicine, healthcare, defense and national security, agriculture, and consumer products. While robots were historically envision static industrial environments, recent advances involve close collaborations between robots and humans. Robotics technologies are now showing promise in their ability to complement, augment, enhance, or emulate human physical capabilities or human intelligence. However, scientists need to make these robotic systems more capable, reliable, and easy-to-use.
Researchers need to better understand robotic perception to extract information from a variety of sensors to provide robots with real-time situational awareness. Progress is needed in cognition and reasoning to allow robots to better understand and interact with the physical world. An improved ability to adapt and learn will allow robots to generalize their skills, perform self-assessment of their current performance, and learn a repertoire of physical movements from human teachers. Mobility and manipulation are areas for further investigation so that robots can move across rugged and uncertain terrain and handle a variety of objects dexterously. Robots need to learn to team together in a seamless fashion and collaborate with humans in a way that is trustworthy and predictable.
Human-AI system interactions have a wide range of objectives. AI systems need the ability to represent a multitude of goals, actions that they can take to reach those goals, constraints on those actions, and other factors, as well as easily adapt to modifications in the goals. In addition, humans and AI system must share common goals and have a mutual understanding of them and relevant aspects of their current states. Further investigation is needed to generalize these facets of human-AI systems to develop systems that require less human engineering.
While much of the prior focus of AI research has been on algorithms that match or outperform people performing narrow tasks, additional work is needed to develop systems that augment human capabilities across many domains. Human augmentation research includes algorithms that work on a stationary device (such as a computer); wearable devices (such as smart glasses); implanted devices (such as brain interfaces); and in specific user environments (such as specially tailored operating rooms). For example, augmented human awareness could enable a medical assistant to point out a mistake in a medical procedure, based on data readings combined from multiple devices. Other systems could augment human cognition by helping the user recall past experiences applicable to the user’s current situation.
Another type of collaboration between humans and AI systems involves active learning for intelligent data understanding. In active learning, input is sought from a domain expert and learning is only performed on data when the learning algorithm is uncertain. This is an important technique to reduce the amount of training data that needs to be generated in the first place, or the amount that needs to be learned. Active learning is also a key way to obtain domain expert input and increase trust in the learning algorithm.
Better visualization and user interfaces are additional areas that need much greater development to help humans understand large-volume modern datasets and information coming from a variety of sources. Visualization and user interfaces must clearly present increasingly complex data and information derived from them in a human-understandable way. Providing real-time results is important in safety-critical operations and may be achieved with increasing computational power and connected systems. In these types of situations, users need visualization and user interfaces that can quickly convey the correct information for real-time response.
A key research challenge is increasing the “explainability” or “transparency” of AI. Many algorithms, including those based on deep learning, are opaque to users, with few existing mechanisms for explaining their results. This is especially problematic for domains such as healthcare, where doctors need explanations to justify a particular diagnosis or a course of treatment. AI techniques such as decision-tree induction provide built-in explanations but are generally less accurate. Thus, researchers must develop systems that are transparent, and intrinsically capable of explaining the reasons for their results to users.
New methods are also needed for verification and validation of AI systems. “Verification” establishes that a system meets formal specifications, while “validation” establishes that a system meets the user’s operational needs. Safe AI systems may require new means of assessment (determining if the system is malfunctioning, perhaps when operating outside expected parameters), diagnosis (determining the causes for the malfunction), and repair (adjusting the system to address the malfunction). For systems operating autonomously over extended periods of time, system designers may not have considered every condition the system will encounter. Such systems may need to possess capabilities for self-assessment, self-diagnosis, and self-repair in order to be robust and reliable.
AI embedded in critical systems must be robust in order to handle accidents, but should also be secure to a wide range of intentional cyber attacks. Security engineering involves understanding the vulnerabilities of a system and the actions of actors who may be interested in attacking it. While cybersecurity R&D needs are addressed in greater detail in the NITRD Cybersecurity R&D Strategic Plan, some cybersecurity risks are specific to AI systems. For example, one key research area is “adversarial machine learning” that explores the degree to which AI systems can be compromised by “contaminating” training data, by modifying algorithms, or by making subtle changes to an object that prevent it from being correctly identified (e.g., prosthetics that spoof facial recognition systems). The implementation of AI in cybersecurity systems that require a high degree of autonomy is also an area for further study. One recent example of work in this area is DARPA’s Cyber Grand Challenge that involved AI agents autonomously analyzing and countering cyber attacks.
With the continuing explosion of data, data sources, and information technology worldwide, both the number and size of datasets are increasing. The techniques and technologies to analyze data are not keeping up with the high volume of raw information sources. Data capture, curation, analysis, and visualization are all key research challenges, and the science needed to extract valuable knowledge from enormous amounts of data is lagging behind. While data repositories exist, they are often unable to deal with the scaling up of datasets, have limited data provenance information, and do not support semantically rich data searches. Dynamic, agile repositories are needed.
The development of standards must be hastened to keep pace with the rapidly evolving capabilities and expanding domains of AI applications. Standards provide requirements, specifications, guidelines, or characteristics that can be used consistently to ensure that AI technologies meet critical objectives for functionality and interoperability, and that they perform reliably and safely.
Adoption of standards brings credibility to technology advancements and facilitates an expanded interoperable marketplace. One example of an AI-relevant standard that has been developed is P1872-2015 (Standard Ontologies for Robotics and Automation), developed by the Institute of Electrical and Electronics Engineers (IEEE). This standard provides a systematic way of representing knowledge and a common set of terms and definitions. These allow for unambiguous knowledge transfer among humans, robots, and other artificial systems, as well as provide a foundational basis for the application of AI technologies to robotics. Additional work in AI standards development is needed across all subdomains of AI. Standards are needed to address:
The importance of testbeds was stated in the Cyber Experimentation of the Future report: “Testbeds are essential so that researchers can use actual operational data to model and run experiments on real-world system[s] … and scenarios in good test environments.” Having adequate testbeds is a need across all areas of AI. The government has massive amounts of mission-sensitive data unique to government, but much of this data cannot be distributed to the outside research community. Appropriate programs could be established for academic and industrial researchers to conduct research within secured and curated testbed environments established by specific agencies. AI models and experimental methods could be shared and validated by the research community by having access to these test environments, affording AI scientists, engineers, and students unique research opportunities not otherwise available.
Government leadership and coordination is needed to drive standardization and encourage its widespread use in government, academia, and industry. The AI community–made up of users, industry, academia, and government—must be energized to participate in developing standards and benchmark programs. As each government agency engages the community in different ways based on their role and mission, community interactions can be leveraged through coordination in order to strengthen their impact. This coordination is needed to collectively gather user-driven requirements, anticipate developer-driven standards, and promote educational opportunities. User-driven requirements shape the objectives and design of challenge problems and enable technology evaluation. Having community benchmarks focuses R&D to define progress, close gaps, and drive innovative solutions for specific problems. These benchmarks must include methods for defining and assigning ground truth. The creation of benchmark simulation and analysis tools wi