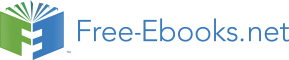

The Role of Big Data
It is important to note that all of these remarkable advancements in machine learning are made possible by, and otherwise depend on, the emergence of big data. The ability of a computer algorithm to generate useful solutions from the data relies on the existence of a lot of data. More data means more opportunity for a computer algorithm to find associations. And as more associations are found, the greater the accuracy of predictions. Just like with humans, the more experience a computer has, the better the results will be.
This trial-and-error approach to computer learning requires an immense amount of computer processing power. It also requires specialized processing power, designed specifically to enhance the performance of machine learning algorithms. The SEC staff is currently using these computing environments and is also planning to scale them up to accommodate future applications that will be on a massive scale. For instance, market exchanges will begin reporting all of their transactions through the Consolidated Audit Trail system, also known as CAT, starting in November of this year.[10] Broker-dealers will follow with their orders and transactions over the subsequent 2 years. This will result in data about market transactions on an unprecedented scale. And, making use of this data will require the analytic methods we are currently developing to reduce the enormous datasets into usable patterns of results, all aimed to help regulators improve market monitoring and surveillance.
We already have some experience with processing big transaction data. Using, again, our big data technologies, such as Hadoop computational clusters that are both on premises and available through cloud services, we currently process massive datasets. One example is the Option Pricing Reporting Authority data, or OPRA data. To help you grasp the size of the OPRA dataset, one day’s worth of OPRA data is roughly two terabytes. To illustrate the size of just one terabyte, think of 250 million, double-sided, single-spaced, printed pages. Hence, in this one dataset, we currently process the equivalent of 500 million documents each and every day. And we reduce this information into more usable pieces of information, including market quality and pricing statistics.
However, with respect to big data, it is important to note that good data is better than more data. There are limits to what a clever machine learning algorithm can do with unstructured or poor-quality data. And there is no substitute for collecting information correctly at the outset. This is on the minds of many of our quant staff. And it marks a fundamental shift in the way the Commission has historically thought about the information it collects. For example, when I started at the Commission almost a decade ago, physical paper documents and filings dominated our securities reporting systems. Much of it came in by mail, and some [documents] still come to us in paper or unstructured format. But this is changing quickly, as we are continuing to modernize the collection and dissemination of timely, machine-readable, structured data to investors.[11]
The staff is also cognizant of the need to continually improve how we collect information from registrants and other market participants, whether it is information on security-based swaps, equity market transactions, corporate issuer financial disclosures, or investment company holdings. We consider many factors, such as the optimal reporting format, frequency of reporting, the most important data elements to include, and whether metadata should be collected by applying a taxonomy of definitions to the data. We consider these factors each and every time the staff makes a recommendation to the Commission for new rules, or amendments to existing rules, that require market participant or SEC-registrant reporting and disclosures.
The Future of Artificial Intelligence at the Commission
So, where does this leave the Commission with respect to all of the buzz about artificial intelligence?
At this point in our risk assessment programs, the power of machine learning is clearly evident. We have utilized both machine learning and big data technologies to extract actionable insights from our massive datasets. But computers are not yet conducting compliance examinations on their own. Not even close. Machine learning algorithms may help our examiners by pointing them in the right direction in their identification of possible fraud or misconduct, but machine learning algorithms can’t then prepare a referral to enforcement. And algorithms certainly cannot bring an enforcement action. The likelihood of possible fraud or misconduct identified based on a machine learning predication cannot – and should not – be the sole basis of an enforcement action. Corroborative evidence in the form of witness testimony or documentary evidence, for example, is still needed. Put more simply, human interaction is required at all stages of our risk assessment programs.
So while the major advances in machine learning have and will continue to improve our ability to monitor markets for possible misconduct, it is premature to think of AI as our next market regulator. The science is not yet there. The most advanced machine learning technologies used today can mimic human behavior in unprecedented ways, but higher-level reasoning by machines remains an elusive hope.
I don’t mean for these remarks to be in any way disparaging of the significant advancements computer science has brought to market assessment activities, which have historically been the domain of the social sciences. And this does not mean that the staff won’t continue to follow the groundbreaking efforts that are moving us closer to AI. To the contrary, I can see the evolving science of AI enabling us to develop systems capable of aggregating data, assessing whether certain Federal securities laws or regulations may have been violated, creating detailed reports with justifications supporting the identified market risk, and forwarding the report outlining that possible risk or possible violation to Enforcement or OCIE staff for further evaluation and corroboration.
It is not clear how long such a program will take to develop. But it will be sooner than I would have imagined 2 years ago. And regardless of when, I expect that human expertise and evaluations always will be required to make use of the information in the regulation of our capital markets. For it does not matter whether the technology detects possible fraud, or misconduct, or whether we train the machine to assess the effectiveness of our regulations – it is SEC staff who uses the results of the technologies to inform our enforcement, compliance, and regulatory framework.
Thank you for your time today.
References
[1] The Securities and Exchange Commission, as a matter of policy, disclaims responsibility for any private publication or statement by any of its employees. The views expressed herein are those of the author and do not necessarily reflect the views of the Commission or of the author’s colleagues on the staff of the Commission. I would like to thank Vanessa Countryman, Marco Enriquez, Christina McGlosson-Wilson, and James Reese for their extraordinary help and comments.
[2] SEC Speech, Has Big Data Made us Lazy?, Midwest Region Meeting of the American Accounting Association, October 2016. https://www.sec.gov/news/speech/bauguess-american-accounting-association-102116.html.
[3] http://cfe.columbia.edu/files/seasieor/center-financial-engineering/presentations/MachineLearningSECRiskAssessment030615public.pdf .
[4] Arthur Samuel, 1959, Some Studies in Machine Learning Using the Game of Checkers. IBM Journal 3, (3): 210-229.
[5] https://en.wikipedia.org/wiki/AlphaGo_versus_Lee Sedol .
[6] For an excellent layperson discussion on how machine learning is enabling all of this, see, e.g., Gideon Lewis- Kraus, The New York Times, December 14, 2016, The Great A.I. Awakening.
[7] See http://www.jmlr.org/papers/volume3/blei03a/blei03a.pdf .
[8] See, G. Hoberg and C. Lewis, 2017, Do Fraudulent Firms Produce Abnormal Disclosure? Journal of Corporate Finance, Vol. 43, pp. 58-85.
[9] Loughran, Tim, and McDonald, Bill, 2011. When is a Liability Not a Liability? Textual Analysis, Dictionaries, and 10-Ks. Journal of Finance 66: 35–65.
[10] See, e.g., https://www.sec.gov/divisions/marketreg/rule613-info.htm.
[11] Securities and Exchange Commission Strategic Plan Fiscal years 2014-2018, https://www.sec.gov/about/sec-strategic-plan-2014-2018.pdf.
Source: https://www.sec.gov/news/speech/bauguess-big-data-ai