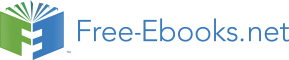

Has Big Data Made Us Lazy?
Scott W. Bauguess Deputy Director and Deputy Chief Economist, DERA
Midwest Region Meeting - American Accounting Association (AAA), Chicago Illinois
Oct. 21, 2016
Thank you, Carol [“Shaokun” Yu] for the introduction.
Thanks also to John Hepp, Jason Stanfield, Jean Thompson, and all of the other conference organizers for the invitation to speak here today, at this year’s Midwest Region meeting of the American Accounting Association (AAA), and for all of the preparation required to make an event like this happen. On a personal note, I grew up in the Chicago area and attended college down south at the U of I, so it’s always a pleasure to have an opportunity to revisit my geographical roots. And finally, the views that I express today are my own and do not necessarily reflect the views of the Commission or its staff.[i]
My prepared remarks this morning center on a topic that, while not new, has received an increasing amount of attention in recent years: “big data.” This is not the first time that I have spoken on this topic, or on how the Commission has harnessed the power of big data in its analytical programs. But this is the first time that I have focused on how the growth of big data may be shaping our behavior. In particular, I want to spend some time expressing my view on the role of human interaction with analytical processes that have developed as a consequence of the proliferation of big data.
The title of my talk is: “Has Big Data Made Us Lazy?” Of course, I wouldn’t have asked the question if I didn’t think there was a component of “yes” in the answer. There is no question that most, if not all, of us have benefited from our new information environment: the data is better; there is more of it. But there are consequences from the proliferation of analytical methods enabled by big data, some of which may not be obvious. What I hope to do, over the course of my talk, is to illustrate how the rise of big data has influenced the way we think about our research and analytical programs in the SEC’s Division of Economic and Risk Analysis, also known as DERA. I think that some of what I have to say has strong parallels with what you may be experiencing in your own academic research and education.
What Is Big Data?
A good place to begin is by asking the question: “What is big data?” The answer frequently depends on whom you ask. Some of the best definitions I’ve heard are based on rules of thumb. And I mean that literally: the amount of data you can fit on a thumb drive. Anything larger is big data. I’ve also heard someone say that big data is anything that you can’t process in a standard desktop application like Excel. But if you talk to academic and other quantitatively oriented researchers working with high-velocity data, such as that generated through equity market trading, they may tell you that big data is anything that takes more than a day to process. Or, perhaps more fittingly, data that takes more time to process than it took to create it – for example, if it takes longer than a day to process a day’s worth of trading data.
A common theme of these and other definitions is the nature of the computing power and software available to process the data. In particular, “big” cannot be defined by quantity alone. What was big last year is not big today. Advancements in computing power, expanded data storage capacity, and faster interconnection speeds all contribute to making data smaller. This has been true for decades. I worked with big data back in the 1990s when I was an engineer. I analyzed millions of data observations that described the underlying physics of the transistor devices that went into microcontrollers and microprocessors. The only difference is that back then I didn’t know to call it big data. So, for the purposes of this talk, assume that when I speak of big data, I am referring to any data that approaches our computational limitations on analyzing it.
How Big Data Is Shaping Analytical Methods
While the rise of big data is a relatively recent innovation, statistical modeling that makes use of data – data of any size – is not. For example, Ordinary Least Squares (OLS) regressions, a widely applied statistical method used in the academic literature today, was first documented and put to use more than 200 years ago.[ii] But until perhaps a little over a decade ago, most applications of OLS, and similar statistical methods, centered on small samples of data. In fact, it was not uncommon for journals to publish papers whose results were drawn from carefully collected samples of less than 100 observations. This was the era when t-statics mattered when interpreting statistical significance.[iii]
Today, studies of this (small) magnitude are few and far between. Research with hundreds of thousands or millions of observations is now commonplace, and researchers are able to conduct many of these large-scale statistical analyses with an ordinary laptop, operated from the comfort of the corner coffee shop. And for more intensive and complicated computing needs, the institutions we work for are no longer required to invest in expensive computing environments that run on premises. Access to high-performance Cloud computing environments is ubiquitous. You can rent CPU time by the cycle, or by the hour, and you can ramp up or down your storage space with seemingly infinite flexibility.
As a result, researchers with access to these computing resources have fewer limitations in the scope of potential empirical studies. And off-the-shelf statistical software packages make good use of these newfound freedoms. We’ve all become experts in applying the most sophisticated of econometric techniques. Gone are the days when we had to program them ourselves in FORTRAN, C, or SAS. You can now rely on user communities to package them into scripts, which can be downloaded and executed in our software of choice with just a few clicks of the mouse.
This has allowed researchers to increase their focus on assessing the robustness of the empirical methods that underlie the conclusions of their studies. It has also raised the expectations of editorial staff at journals that they do so. For example, I would be surprised if there is a researcher in this room who hasn’t faced the econometric challenge of determining causal inference. The issue is pervasive in almost all empirical work: how can we prove the direction of causality among the correlations we observe in our studies?
Most social sciences research doesn’t have the benefit of controlled experiments and must rely on natural observations. The academic literature increasingly emphasizes the need to identify instrumental variables in regressions, or natural experiment settings, to control for potential reverse causality between the correlations we observe and are trying to explain in our studies.
This pursuit of econometric perfection, and use of advanced statistical methods, has had some potentially unintended consequences. The first, in my subjective assessment, is that many journal articles devote fewer pages to descriptive statistics and stylized facts than they once did. In their place, we find batteries of econometric robustness checks. But when publication is dependent on “econometric proof,” it can limit the discovery and examination of basic market and agent behaviors. In particular, there are benefits to documenting correlations, trends, and styled facts even if their nature is not yet well understood. Doing so can provide the basis for interesting hypotheses and new theoretical models, which could in turn generate more empirical research.
The second effect of this pursuit, and an equally subjective assessment on my part, is that this has encouraged the rapid emergence of the new field of data science. You may not yet be familiar with what this encompasses, but I’m sure everyone here is well aware of terms like “machine learning,” “neural networks,” “data mining,” and “natural language processing.” These are among the computational solutions that computer science and applied mathematics have brought to big data analytics. Importantly, underlying this new field is a philosophy that analytical approaches should start from the data, with important insights generated by creating algorithms designed to recognize trends and patterns therein.
For those of you like me, whose training is grounded in the social sciences, learning about the application of these analytical methods represents a paradigm shift. Formal deductive processes, and parsimonious regression models, are replaced by a bottom-up, data-driven approach that lets “data speak for itself.” The social sciences teach us that data mining is forbidden; it generates spurious correlations that lead to incorrect conclusions about the way the world works. Data science is premised on the very opposite: for instance, it’s up to the observer to decide what to do when informed by an online retailer that other shoppers also bought band-aids after purchasing razor blades.
If you search blog posts and internet forums on the subject, you will find an active debate on the tradeoffs of each approach. The core difference I see is that social scientists seek to understand why relations exist—that is, they develop and test hypotheses that help us understand human nature. Data scientists seek to understand trends and are more focused on predicting behavior than understanding it. They build models that fit the data. And they privilege accuracy over intuition. A social scientist may view this as lazy. A data scientist views this as practical.
Analytical Programs at the SEC
Why are these different perspectives important for how we approach data analytics at the SEC? I’ve been at the Commission for nearly a decade. During that time, I have worked on a large number of policy issues. The economic analyses that have supported these policy decisions are predominantly grounded in the theory-driven research of social scientists. They rely on carefully constructed analyses that seek to address causal inference, which is crucial to understanding the potential impact of a new regulation.
But in the last few years, I’ve witnessed the arrival of increasingly complex data and new analytical methods used to analyze them. And some of these analytical methods are allowing analyses of previously impenetrable information sets – for example, those without structure, such as freeform text. This has been of particular interest to the SEC, where registrant filings are often in the form of a narrative disclosure. So, as a result, we have begun a host of new initiatives that leverage the machine learning approach to behavioral predictions, particularly in the area of market risk assessment, which includes the identification of potential fraud and misconduct.
Today, the SEC, like many other organizations, is adopting these new methodologies at a very rapid pace. Of course, this is not to say that we are letting go of classical statistical modeling. And, as I would like to focus on now, none of our analytical programs, whether grounded in classical statistical modeling, or machine learning, can replace human judgment, which remains essential in making the output of our analytical models and methods actionable. To understand why, let me give you some examples.
Let me begin with the Corporate Issuer Risk Assessment Program, also known as CIRA, which relies on classical statistical modeling developed by DERA economists and accountants in collaboration with expert staff in the SEC’s Division of Enforcement. This program grew out of an initiative originally referred to as the “accounting quality model,” or, AQM, which was itself rooted in academic research. In particular, AQM focused on estimates of earnings quality and indications of inappropriate managerial discretion in the use of accruals. As former DERA Division Director and SEC Chief Economist Craig Lewis noted, “[a]cademics in finance and accounting have long studied the information contained in financial statements to better understand the discretionary accounting choices that are made when presenting financial information to shareholders.”[iv]
Today, the CIRA program includes these modeling measures of earnings quality as part of more than two hundred thirty (230) custom metrics provided to SEC staff. These include measures of earnings smoothing, auditor activity, tax treatments, key financial ratios, and indicators of managerial actions. Importantly, they are readily accessible by SEC staff through an intuitive dashboard customized for their use. Referencing DERA’s collaboration with the Division of Enforcement’s FRAud Group, Enforcement Division Director Andrew Ceresney noted earlier this year, “CIRA provides us with a comprehensive overview of the financial reporting environment of Commission registrants and assists our staff in detecting anomalous patterns in financial statements that may warrant additional inquiry.”[v]
However, this was not how the press first reported on the original initiative when it coined the term “Robocop” to describe it — as if a machine makes the important decisions in identifying potential market risks. As our current DERA Director and Chief Economist Mark Flannery recently noted, “this implied perspective is at best inaccurate and at worst misleading. While these activities use quantitative analytics designed to help prioritize limited agency resources, the tools we in DERA are developing do not — indeed cannot — work on their own.”[vi]
But at the same time, some of the most exciting developments at the Commission have centered on machine learning and text analytics. While machine learning methods have been around since the 1950s,[vii] it is the arrival of big data and high performance computing environments that has advanced their uses. At the Commission, this has taken on several forms. At the most basic level, and consistent with methods that are now commonplace in academic research, we have extracted words and phrases from narrative disclosures in forms and filings. For example, by applying a programming technique that uses human-written rules to define patterns in documents, referred to as “regular expressions,” [viii] we are able to systematically measure and assess how emerging growth companies are availing themselves of JOBS Act provisions through what they disclose in their registration statements.
More recently, we have adopted topic modeling[ix] methods to analyze tens of thousands of narrative disclosures contained in registrant filings. For those of you not familiar with topic modeling, when applied to a corpus of documents, it can identify groups of words and phrases across all documents that pertain to distinct concepts (“topics”) and simultaneously generate the distribution of topics found within each specific document. We are also performing sentiment analysis using natural language processing techniques to assess the tonality[x] of each filing – for example, identify those with a negative tone, or a tone of obfuscation. We then map these topic and tonality “signals” into known measures of risk – such as examination results or past enforcement actions – using machine learning algorithms. Once trained, the final model can be applied to new documents as they are filed by registrants, with levels of risk assigned on the basis of historical findings across all filers. This process can be applied to different types of disclosures, or to unique categories of registrants, and the results then used to help inform us on how to prioritize where investigative and examination staff should look.
While this machine-learning approach to text analytics has provided a new and exciting way to detect potential market misconduct, just as with classical modeling methods, it does not work on its own. In particular, while a model may classify a filing as high risk, the classification does not provide a clear indicator of potential wrongdoing. To the contrary, many machine learning methods do not generally point to a particular action or conduct indicative of fraud or other violation. The human element remains a necessary part of the equation.
We Still Need Humans in the Big Data World
More generally, what both of these risk assessment programs illustrate is that our analytic initiatives must rely on SEC staff to operate them and assess their results. This is not a flaw in their design. Rather, initiatives that reflect a more classical modeling approach need thoughtful design on the front end that only a human with significant experience and insights into the market can provide. And on the flip side, the machine-learning initiatives require care and thought on the back end, from the human user, who needs to bring that same experience to interpreting the results and deriving meaning beyond simple (or not so simple) correlations. In all cases, the human role is essential when using the results to inform on critical decisions related to policy issues or risk assessment.
The SEC undertakes these and many other initiatives as part of its overarching commitment to protecting investors. To that end, the results from these risk assessment models and tools – whether based on a classical modeling or a machine-learning approach – can tell us only where to focus our attention in the market. The methods may provide indicia of potential wrongdoing but cannot identify misconduct without an investigator or examiner engaging in further, expert inquiry. That can involve assessing results of analytics; deciding to conduct additional, empirical research; or using staff expertise to determine whether the results of the analytic model require additional evidence to meet the elements of a securities law violation.
Importantly, no matter what analytical method is used to identify, for example, possible securities fraud, we still need experts at the SEC to be able to identify: (1) manipulation or deception through misrepresentation and/or omission; (2) materiality; (3) that the possible violative conduct was "in connection with" the purchase or sale of securities, and (4) Scienter—intent or knowledge of wrongdoing. This is needed in order to meet the threshold requirements to file a securities fraud action in Federal District Court. Hence, analytics are only the first step.
An illustration of how human intervention can make the analytic results of these programs more powerful is when they are combined with the results from other information sources, such as a tip, complaint, or referral, referred to as a TCR, from a market participant or another regulator. In particular, we often receive information of alleged wrongdoing by an entity that is covered by one of our risk assessment programs or tools. In these cases, an investigator can use the output to seek instant corroboration or evidence consistent with the allegation. This can make the TCR more immediately actionable and result in more effective disposition, increasing the overall effectiveness of our market monitoring programs.
More generally, the success of an analytical program designed to detect fraud or misconduct depends on developing methods and models that accurately capture what our expert investigative and examination staff often know through their considerable experience. That is, the analytical methods must identify risk factors and outcomes that match what the investigators and examiners know about market misconduct. This requires translating expert knowledge into observations that can be described numerically so that algorithms can derive metrics collected from common data sources that proxy for these risk factors.
Where appropriate, we plan to continue to borrow from the academic literature when generating new risk assessment ideas, such as we did by measuring levels of discretionary accruals as an indicator of potential earnings management. We are actively reviewing the academic literature for research that centers on potential wrongdoing, market manipulation, fraud, and other activities that may lead to investor harm. I call these “academic TCRs” – “referrals” by academic researchers of market activity that may warrant a closer look. Over the years, we’ve welcomed many of these researchers into DERA’s seminar series to discuss their findings, and the exchange of knowledge has contributed significantly to our programs and hopefully to their research.
A Final Thought on the Quality of the Underlying Data
Before I conclude, I’d like to touch on one other important aspect of any data-driven initiative. With all of these programs, we still need to be thoughtful about the sources of the data that inform them. In particular, much of the data we use to inform the models and analytical methods comes from mandated disclosures by the SEC and other regulators.
It remains important to think about what information we collect in anticipation of how we are going to analyze it. While computing power can solve the time dimension of processing data, it cannot improve the accuracy of it. Digital photography offers an excellent example of this. In the olden days, when we had to take our film to a store to get it developed, the time and pecuniary costs of doing so provided discipline in the manner in which we snapped our pictures. We were thoughtful in how we framed them. Today, we snap pictures from our phones by the bulk load, and they are instantly streamed to our home computers. The image-capture process is now costless. As a result, we have less incentive to be thoughtful on the front end of the process, and spend all of our time on the back end trying to figure out whether any of them actually worked out. One could argue that we have not made our lives easier, but simply shifted where we apply our time and effort.
Our experiences with digital photography illustrate that the usefulness of data does not necessarily increase at the same rate as its growth. Just because we have a lot of data doesn't ensure that there will be an application for it all. And big data can’t fix bad empirical methods, and bad data can’t be analyzed no matter how big it is. Data quality remains important no matter its size. So, we continue to need to think carefully about how we collect data. And just as we did in assembling small data samples in a prior era, we need to do the same when generating large samples today.
For investors and staff at the Commission who monitor markets, that often depends on the design of the forms that registrants are required to file or information that they are otherwise required to report. By thinking carefully about our disclosures, as we do with each of our rulemaking initiatives for which disclosure is required, we allow users of the data to be a little “lazy” if they choose to let the data speak for itself, because the answers are rooted in questions that were purposefully drafted.
Conclusion
So let me return to the question I posed earlier – “Has Big Data Made Us Lazy?” I think the answer is simply, “It shouldn't.” The programs that DERA helms, in close collaboration with colleagues across the SEC, are a vibrant example of how classical modeling and machine learning methods both have a vital place in approaches to understanding any complex area, such as the financial markets. But both are merely tools – albeit potentially very powerful ones – in the search for meaning that is fundamentally human.
Thank you so much for your time today.
[i] The Securities and Exchange Commission, as a matter of policy, disclaims responsibility for any private publication or statement by any of its employees. The views expressed herein are those of the author and do not necessarily reflect the views of the Commission or of the author’s colleagues on the staff of the Commission.
[ii] https://en.wikipedia.org/wiki/Least_squares
[iii] A t-test is used to test hypotheses centered on whether two samples of data are statistically different from one another (i.e., have different means) and assumes that the samples follow a normal distribution.
[iv] Craig Lewis, Chief Economist and Director, Division of Risk, Strategy, and Financial Innovation, U.S. Securities & Exchange Commission, Financial Executives International Committee on Finance and Information Technology, Dec. 13, 2012.
[v] Andrew Ceresney, Director, Division of Enforcement, U.S. Securities & Exchange Commission, Directors Forum 2016 Keynote Address.
[vi] Mark Flannery, Director, Division of Economic and Risk Analysis, U.S. Securities & Exchange Commission, Global Association of Risk Professionals Risk Assessment Keynote Address.
[vii] See Arthur L. Samuel, Some Studies in Machine Learning Using the Game of Checkers, IBM Journal, Vol. 3, No. 3, July 1959.
[viii] Thompson, K. (1968). "Programming Techniques: Regular expression search algorithm." Communications of the ACM. 11 (6): 419–422. doi:10.1145/363347.363387.
[ix] See, for example, David Blei, "Probabilistic Topic Models," Communications of the ACM. 55, April 2012.
[x] See, e.g., Tim Loughran and Bill McDonald, 2011, “When is a Liability not a Liability? Textual Analysis, Dictionaries, and 10-Ks,” Journal of Finance, 66:1, 35-65.
Source: https://www.sec.gov/news/speech/bauguess-american-accounting-association-102116.html