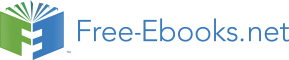

Big Data to Knowledge at NIH
As biomedical tools and technologies rapidly improve, researchers are producing and analyzing an ever-expanding amount of complex biological data called “big data.” The Big Data to Knowledge (BD2K) program is a trans-NIH initiative that was launched in 2013 to support the research and development of innovative and transformative approaches and tools to maximize and accelerate the integration of big data and data science into biomedical research. The BD2K Program also supported initial efforts toward making data sets “FAIR” Findable, Accessible, Interoperable, and Reusable. Learn more about the FAIR principles.
Big Data to Knowledge Phase I & II
In its first phase (FY2014-FY2017), BD2K invested $200 million in grant awards to address some major data science challenges and to stimulate data-driven discovery. It focused on facilitating broad use of biomedical big data, developing and disseminating analysis methods and software, enhancing training relevant for large-scale data analysis,and establishing centers of excellence for biomedical big data. These awards will continue through award end dates, and lessons from this initial investment will help inform the second phase of the program (FY2018-FY2021).
BD2K has now entered a second phase that will focus on making the products of research developed in Phase I usable, discoverable, and disseminated to their intended end-users. In addition, the program will continue to pursue approaches to making biomedical big data Findable, Accessible, Interoperable, and Reusable or “FAIR.” It will support the NIH Data Commons Pilot Phase, a trans-NIH initiative to test the feasibility of, and develop best practices for, making NIH-funded data sets and computational tools available through communal, collaborative platforms on public clouds.
BD2K Centers
BD2K funded 13 Centers of Excellence, which are large-scale projects developing new approaches, methods, software tools, and related resources, and are also providing training to advance Big Data science in the context of their biomedical area of focus. The Centers are located all across the United States and function with the other BD2K grantees as a consortium and collaborate with one another for the purpose of furthering every aspect of the field of biomedical data science research.
Big Data for Discovery Science (BDDS)
Researchers at the BDDS focus on proteomics, genomics, and images of cells and brains collected from patients and subjects across the globe. They enable detection of patterns, trends, and relationships among these data for the efficient large-scale analysis of biomedical data.
BD2K-LINCS Data Coordination and Integration Center (BD2K-LINCS DCIC)
The BD2K-LINCS DCIC conducts data science research focused on perturbation-response data obtained from experiments with human cells and tissues, and provides access to and analysis of this data by the broader biomedical research community.
Center for Big Data in Translational Genomics (BDTG )
The BDTG creates data models and analysis tools to analyze massive datasets of genomic information to uncover the contribution of gene variants to disease with an initial focus on cancer.
Center for Causal Modeling and Discovery of Biomedical Knowledge from Big Data (CCD )
Center for Causal Modeling & Discovery of Biomedical Knowledge from Big Data (CCD) develops computational methods known as causal discovery algorithms that can be used to discover causal relationships from a combination of observational data, experimental data, and prior knowledge.
Center for Expanded Data Annotation and Retrieval (CEDAR )
Center for Expanded Data Annotation and Retrieval (CEDAR) is building new web-based technology to make it easier for biomedical scientists to author detailed metadata that describe their experiments completely, adhere to appropriate community-based standards, and incorporate controlled terms that facilitate interoperability with other online data sets.
Center for Mobility Data Integration to Insight (The Mobilize Center )
The Mobilize Center is analyzing movement data from over 6 million individuals using a smartphone app, revealing new insights about physical activity levels around the world and the factors predictive of these activity levels.
BD2K Centers Resources
The BD2K Centers have developed a wide array of tools and resources. A list of these resources is maintained by the BD2K Centers Coordination Center (BD2KCCC) . The BD2KCCC helps to promote collaboration among the Centers and across the BD2K program, and coordinates BD2K Centers Consortium activities.
Resources from each BD2K Center were highlighted in a special issue of the Summer 2017 Biomedical Computational review . Learn more about some of the BD2K Centers exciting accomplishments.
Tools and resources are also available on the individual Centers resource pages:
BDDS
BD2K-LINCS DCIC
CCD
CEDAR
The Mobilize Center
CPCP
MD2K
ENIGMA
HeartBD2K
KnowEnG
PIC-SURE
The Big Data to Knowledge Cloud Credits Model
What is the Cloud Credits Model?
On-demand, cloud-based storage and computing resources are becoming more commonplace in biomedical research. However, direct acquisition of these services by investigators can be complicated and inefficient. As one step to help overcome these barriers, the NIH BD2K program has developed a Cloud Credits Model. This pilot project provides pre-paid credits that allow NIH-funded investigators to access modern, cloud-based technology to support their research objectives. Through the credits, NIH can test a business model for making data storage and analysis in the cloud more efficient.
The Cloud Credits Model is a component of the first phase of the Big Data to Knowledge Program (BD2K). This project supports B2DK’s aim to facilitate access and use of biomedical big data. NIH expects that the lessons learned from the Cloud Credits Model will apply to second-phase BD2K initiatives, including the efforts of the NIH Data Commons Pilot Phase, which will make use of cloud technologies to advance biomedical research.
The Cloud Credits Model involves:
Using existing, centralized agreements with cloud vendors that conform to NIH requirements for capacity, security, accessibility, and other key criteria
Creating a marketplace of cloud resource providers and cloud resource consumers (investigators), to test the application of private sector operating approaches to a government sponsored effort
Assessing the model for effectiveness and efficiency with respect to ease to of use and cost
Participating investigators can select services from multiple cloud service providers that conform to NIH specifications. Cloud credits are requested through and issued by the Centers for Medicare & Medicaid Services (CMS) Alliance to Modernize Healthcare (CAMH) Federally Funded Research and Development Center (FFRDC), operated by The MITRE Corporation. The Cloud Credits Model is not connected to the NIH grants mechanism and does not impact investigators’ grants.
What does NIH hope to gain from the Cloud Credits Model?
NIH hopes to learn whether this model could be used in the future to acquire services and to compute on, store, and share biomedical big data at scale in the cloud. The primary goals are to create efficiencies for investigators, facilitate cost savings for NIH, and promote and share data generated by the community.
To date, the Cloud Credits Model has issued an “alpha” round of credits to 8 investigators to test the process of issuing credits, gaining access to cloud resources, and tracking usage. Over $100,000 in credits have been consumed, and reporting systems and dashboards have been created that inform participants as well as the NIH of spending by account and category.
Additionally, in preparation for issuance of additional credits to investigators, the Cloud Credits Model project team has built capacity for providing basic technical assistance across conformant cloud resources. Lastly, tools for capturing final outcomes, including a survey and post implementation report, have been developed.
In summary, the Cloud Credits Model offers the following:
An “on-ramp” to the cloud for participating investigators
Training opportunities for the community and for NIH
Processing efficiencies (accounts, invoices, and other administration efforts)
Incentives for investigators to create and share the data / tools / workflows that they created using credits
Insight into resource use to inform decisions about cost-sustainability
Experience working with cloud resource providers, including the ability to extend business relationships for future initiatives
Resource Indexing
To harness the full potential of Big Data scientists must be able to readily find, cite, and access existing data and other digital objects, such as software. There is no existing infrastructure or incentive that enables this. These basic goals maximize data use, enable sharing, limit duplication of effort, and allow areas of sparse research coverage to be more readily identified. To advance the infrastructure and policies needed to meet these goals, awards in this area address the challenges of resource discovery, citation, and access.
The Data Discovery Index concept furthers BD2K’s goal of improving the sharing of biomedical data. It will enable researchers to make better use of what already exists. It will also allow them to produce datasets that complement existing data for greater analytic potential.
In 2014, BD2K awarded a Data Discovery Index Coordination Consortium grant to the BioCADDIE project. BD2K also made a series of Data Discovery Index Supplement awards. These grants permit existing NIH-funded projects to join the consortium activities.
Data Discovery Index Coordination Consortium (DDICC) Award
Biological and HealthCare Data Discovery and Indexing Ecosystem (bioCADDIE)
bioCADDIE seeks to develop a prototype DDI that will enable finding, accessing and citing biomedical big data. bioCADDIE has a Community Engagement mandate that seeks to work with the broader biomedical community to better identify data, and other digital objects, so that they may find shared data in ways that allow for extracting maximal knowledge.
www.biocaddie.orgExit Link Disclaimer
Data Discovery Index (DDI) Supplement Awards
The Cardiovascular Research Grid
Johns Hopkins University
PI: Raimond Lester Winslow
Grant Number: 3R24HL085343-08S1
The Cardiovascular Research Grid (CVRG) Project is a national resource providing the capability to store, manage, and analyze data on the structure and function of the cardiovascular system in health and disease. The CVRG will develop new tools that will enhance the ability of researchers to explore and analyze their data to understand the cause and treatment of heart disease.
Computational tools for the analysis of high-throughput immunoglobulin sequencing
Yale University
PI: Steven H. Kleinstein
Grant Number: 1R01AI104739-01A1
This project will develop and validate computational methods to analyze large-scale immunology sequencing data sets. These methods will provide insights into the mechanisms underlying autoimmune disease, as well as biomarkers for susceptibility to infection or vaccination response.
Discovering and Applying Knowledge in Clinical Databases
Columbia University Health Sciences
PI: George M. Hripcsak
Grant Number: 3R01LM006910-15S1
This project uses data mining and knowledge engineering studies the electronic health record in order to better understand how health care processes cause systematic bias and other problems in the data which complicate incorporation into scientific studies. By avoiding or correcting those problems, we hope to improve reuse of the data for purposes such as clinical research and quality improvement.
fMRI-based Biomarkers for Multiple Components of Pain
University of Colorado
PI: Tor Dessart Wager
Grant Number: 3R01DA035484-02S1
Current treatments for pain are only modestly effective, in large part because pain is created through a complex set of brain processes and can be measured only by patients' self-reports, which presents a serious barrier to effective research and treatment. This project capitalizes on recent breakthroughs in measuring human brain activity and using it to objectively assess the brain processes that underlie pain experience, which could transform the way pain is measured and new treatments are developed.
Generation of a centralized and integrated resource for exposure data
North Carolina State University – Raleigh
PI: Carolyn J. Mattingly
Grant Number: 3R01ES019604-04S1
Most human diseases involve interactions between genetic and environmental factors; however, the basis of these complex interactions is not well understood. This project will enhance the capacity for prediction, analysis and interpretation of environment-disease networks by developing novel analysis and visualization tools that include exposure data. These tools will leverage the public Comparative Toxicogenomics Database (CTD), which aims to promote understanding about environment-disease relationships.
A Hub for the Nuclear Receptor Signaling Atlas
Baylor College of Medicine
PIs: Bert W. O’Malley, Ronald Evans, and Neil McKenna
Grant Number: 3U24DK097748-03S1
Nuclear receptors (NRs) and their coregulators are important therapeutic targets in many different disease states including cancer, obesity, diabetes, inflammation, neurological disorders and senescent diseases. This project will produce a NR research community resource hub for information and data analysis tools and will provide community research grants to generate datasets to populate the hub. These initiatives will have tangible benefits for the progress of research in the field towards developing novel NR- and coregulator-based therapeutics.
Natural language processing for clinical and translational research
The Mayo Clinic – Rochester
PIs: Hongfang Liu, Serguei Pakhomov, and Hua Xu
Grant Number: 3R01GM102282-02S1
Rapid growth in the clinical implementation of large electronic medical records (EMRs) has led to an unprecedented expansion of datasets for clinical and translational research. This project will develop a novel natural language processing framework to enable the use of information embedded in clinical narratives for research.
Using Biomedical Knowledge to Identify Plausible Signals for Pharmacovigilance
Drexel University
PI: Andrew Robert Cohen
Grant Number: 5R01NS076709-04
This project will develop and evaluate methods to identify automatically biologically plausible adverse drug events found within clinical patient records, using knowledge extracted from the biomedical literature. If successful, these methods will provide the means for earlier detection of harmful drug effects, limiting consequent morbidity and mortality.
PI Name |
Institution Name |
Title |
AHALT, STANLEY CARLTON |
UNIV OF NORTH CAROLINA CHAPEL HILL |
A Collaboration for the NIH Data Commons |
BROWN, C TITUS |
UNIVERSITY OF CALIFORNIA AT DAVIS |
Tools and Workflows for Mining Genomic Data on Many Clouds |
CROSAS, MERCE |
HARVARD UNIVERSITY |
Towards a FAIR Digital Ecosystem in the Cloud |
DAVIS-DUSENBERY |
SEVEN BRIDGES GENOMICS, INC. |
FAIR Data to Drive CURES |
FOSTER, IAN |
UNIVERSITY OF CHICAGO |
A Commons Platform for Promoting Continuous FAIRness |
KOHANE, ISAAC S |
HARVARD MEDICAL SCHOOL |
Patient-Centric Information Commons under FAIR Principles (PIC-FAIR) |
MA'AYAN, AVI |
ICAHN SCHOOL OF MEDICINE AT MOUNT SINAI |
Development and Implementation Plan for Community Supported FAIR Guidelines and Metrics |
OHNO-MACHADO, LUCILA (contact) |
UNIVERSITY OF CALIFORNIA SAN DIEGO |
CALIFORNIA: Cloud-agnostic Architecture to Locate Indexed FAIR Objects and safely Reuse them in New Integrated Analyses |
PATEN, BENEDICT (contact) |
UNIVERSITY OF CALIFORNIA SANTA CRUZ |
The Commons Alliance: A Partnership to Catalyze the Creation of an NIH Data Commons |
WHITE, OWEN R |
UNIVERSITY OF MARYLAND BALTIMORE |
University of Maryland NIH Data Commons Facilitation Center |
Administrative Supplements to Existing NIH Grants and Cooperative Agreements (Admin Supp) PA16-287 |
||
PI Name |
Institution Name |
Title |
ABECASIS, GONCALO |
UNIVERSITY OF MICHIGAN |
Studies of Rare Genetic Variation in the Isolated Population of Sardinia |
ARDLIE, KRISTIN (contact) |
BROAD INSTITUTE, INC. |
A portal and integrative collaborative analysis platform for GTEx |
CHERRY, JOE MICHAEL |
STANFORD UNIVERSITY |
|
PSATY, BRUCE M (contact) |
UNIVERSITY OF WASHINGTON |
NIH Data Commons Pilot Support Services |
|
|
|
Contractor Name |
Title |
MITRE Corporation |
NIH Data Commons Pilot Support Services |
Big Data to Knowledge (BD2K) Community-Based Data and Metadata Standards Efforts (R24) RFA-ES-16-010 |
||
PI Name |
Institution Name |
Title |
DEUTSCH, ERIC |
INSTITUTE FOR SYSTEMS BIOLOGY |
Advancing data and metadata standards for proteomics mass spectra |
PETERS, BJOERN (contact) |
LA JOLLA INST FOR ALLERGY & IMMUNOLGY |
|
SIM, IDA |
UNIVERSITY OF CALIFORNIA, SAN FRANCISCO |
Open mHealth: Community-Based Data and Metadata Standards for Mobile Health |
BD2K Support for Meetings of Data Science Related Organizations (U13) RFA-CA-16-020 |
||
PI Name |
Institution Name |
Title |
HAENDEL, MELISSA A (contact) |
OREGON HEALTH & SCIENCE UNIVERSITY |
PI Name |
Institution Name |
Title |
SARKAR, INDRA NEIL (contact) |
BROWN UNIVERSITY |
Training and Teaching for Transforming Big Data to Knowledge |
BD2K Enhancing Diversity in Biomedical Data Science (R25) RFA-MD-16-002 |
||
PI Name |
Institution Name |
Title |
BAI, YONGSHENG (contact) |
INDIANA STATE UNIVERSITY |
|
GIANNOPOULOU, EVGENIA (contact) |
NEW YORK CITY COLLEGE OF TECHNOLOGY |
City Tech-WCM Big Data Training Program in Biomedical Informatics |
MARQUEZ-MAGANA, LETICIA MARIA (contact) |
SAN FRANCISCO STATE UNIVERSITY |
HEART & SOUL: Enabling full representation in biomedical Big Data science |