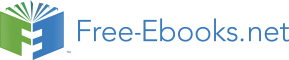

Artificial intelligence (AI)
The Comptroller General convened a Forum on Artificial intelligence (AI) in early 2018 to consider the policy and research implications of AI’s use in 4 areas with the potential to significantly affect daily life—cybersecurity, automated vehicles, criminal justice, and financial services. The forum highlighted the fact that AI will have far-reaching effects on society and could improve human life and economic competitiveness—but it also poses new risks.
Regarding opportunities, investment in automation through AI technologies could lead to improvements in productivity and economic outcomes, similar to that experienced during previous periods of automation, according to a forum participant. In cybersecurity, AI automated systems and algorithms can help identify and patch vulnerabilities and defend against attacks. Automotive and technology firms use AI tools in the pursuit of automated cars, trucks, and aerial drones. In criminal justice, algorithms are automating portions of analytical work to provide input to human decision makers in the areas of predictive policing, face recognition, and risk assessments. Many financial services firms use AI tools in areas like customer service operations, wealth management, consumer risk profiling, and internal controls.
Forum participants also highlighted a number of challenges related to AI. For example, if the data used by AI are biased or become corrupted by hackers, the results could be biased or cause harm. The collection and sharing of data needed to train AI systems, a lack of access to computing resources, and adequate human capital are also challenges facing the development of AI. Furthermore, the widespread adoption of AI raises questions about the adequacy of current laws and regulations. Finally, participants noted the need to develop and adopt an appropriate ethical framework to govern the use of AI in research, as well as explore factors that govern how quickly society will accept AI systems in their daily lives.
After considering the benefits and challenges of AI, forum participants highlighted several policy issues they believe require further attention. In particular, forum participants emphasized the need for policymakers to explore ways to (1) incentivize data sharing, such as providing mechanisms for sharing sensitive information while protecting the public and manufacturers; (2) improve safety and security (e.g., by creating a framework that ensures that the costs and liabilities of providing safety and security are appropriately shared between manufacturers and users); (3) update the regulatory approach that will affect AI (e.g., by leveraging technology to improve and reduce the burden of regulation, while assessing whether desired outcomes are being achieved); and (4) assess acceptable levels of risk and ethical considerations (e.g., by providing mechanisms for assessing tradeoffs and benchmarking the performance of AI systems).
As policymakers explore these and other implications, they will be confronted with fundamental tradeoffs, according to forum participants. As such, participants highlighted several areas related to AI they believe warrant further research, including (1) establishing regulatory sandboxes (i.e., experimental safe havens where AI products can be tested); (2) developing high-quality labeled data (i.e., data organized, or labeled, in a manner to facilitate their use with AI to produce more accurate outcomes); (3) understanding the implications of AI on training and education for jobs of the future; and (4) exploring computational ethics and explainable AI, whereby systems can reason without being told explicitly what to do and inspect why they did something, making adjustments for the future.
The Congressional Artificial Intelligence Caucus exists to inform policymakers of the technological, economic and social impacts of advances in AI and to ensure that rapid innovation in AI and related fields benefits Americans as fully as possible. The AI Caucus brings together experts from academia, government and the private sector to discuss the latest technologies and the implications and opportunities created by these new changes.
“It’s time to get proactive on artificial intelligence,” said Congressman Delaney, co-chair of the House AI Caucus. “AI is going to reshape our economy the way the steam engine, the transistor or the personal computer did and as a former entrepreneur, I believe the impact will be positive overall. Big disruptions also create new policy needs and we should start working now so that AI is harnessed in a way that society benefits, that businesses benefit and that workers benefit. Rep. Pete Olson said, “Artificial Intelligence has the power to truly transform our society, and as policymakers, we must be forward thinking about its applications. An AI Advisory committee will help ensure that the federal government enables growth and advancement in this exciting field, while empowering Congress to address potential AI issues going forward. Action on this initiative has been slow.
Select Committee on Artificial Intelligence
In order to improve the coordination of Federal efforts related to AI and ensure continued U.S. leadership in AI, The White House chartered a Select Committee on Artificial Intelligence (“Select Committee”) under the National Science and Technology Council. The membership of the Select Committee is comprised of the most senior R&D officials in the Federal Government. The Select Committee will:
The Select Committee will also provide guidance and direction to the existing ML/AI subcommittee, which will continue to serve as community of practice for Federal AI researchers. The Select Committee will be chaired by The White House Office of Science and Technology Policy (OSTP), the National Science Foundation (NSF), and the Defense Advanced Research Projects
Select Committee membership will include the most senior R&D officials of the Federal Government, including the Undersecretary of Commerce for Standards and Technology, Undersecretary of Defense for Research and Engineering, the Undersecretary of Energy for Science, the Director of NSF, and the Directors of DARPA and IARPA. The Select Committee will also include representatives from the National Security Council, the Office of the Federal Chief Information Officer, the Office of Management and Budget, and OSTP.
The advance of technology has evolved the roles of humans and machines in conflict from direct confrontations between humans to engagements mediated by machines. Originally, humans engaged in primitive forms of combat. With the advent of the industrial era, however, humans recognized that machines could greatly enhance their warfighting capabilities. Networks then enabled teleoperation, which eventually proved vulnerable to electronic attack and subject to constraint due to long signal propagation distances and times. The next stage in warfare will involve more capable autonomous systems, but before we can allow such machines to supplement human warfighters, they must achieve far greater levels of intelligence.
Traditionally, we have designed machines to handle well-defined, high-volume or high-speed tasks, freeing humans to focus on problems of ever-increasing complexity. In the 1950s and 1960s, early computers were automating tedious or laborious tasks. It was during this era that scientists realized it was possible to simulate human intelligence and the field of artificial intelligence (AI) was born. AI would be the means for enabling computers to solve problems and perform functions that would ordinarily require a human intellect.
Early work in AI emphasized handcrafted knowledge, and computer scientists constructed so-called expert systems that captured the specialized knowledge of experts in rules that the system could then apply to situations of interest. Such “first wave” AI technologies were quite successful – tax preparation software is a good example of an expert system – but the need to handcraft rules is costly and time-consuming and therefore limits the applicability of rules-based AI.
The past few years have seen an explosion of interest in a sub-field of AI dubbed machine learning that applies statistical and probabilistic methods to large data sets to create generalized representations that can be applied to future samples. Foremost among these approaches are deep learning (artificial) neural networks that can be trained to perform a variety of classification and prediction tasks when adequate historical data is available. Therein lies the rub, however, as the task of collecting, labelling, and vetting data on which to train such “second wave” AI techniques is prohibitively costly and time-consuming.
DARPA envisions a future in which machines are more than just tools that execute human-programmed rules or generalize from human-curated data sets. Rather, the machines DARPA envisions will function more as colleagues than as tools. Towards this end, DARPA research and development in human-machine symbiosis sets a goal to partner with machines. Enabling computing systems in this manner is of critical importance because sensor, information, and communication systems generate data at rates beyond which humans can assimilate, understand, and act. Incorporating these technologies in military systems that collaborate with warfighters will facilitate better decisions in complex, time-critical, battlefield environments; enable a shared understanding of massive, incomplete, and contradictory information; and empower unmanned systems to perform critical missions safely and with high degrees of autonomy. DARPA is focusing its investments on a third wave of AI that brings forth machines that understand and reason in context.
For more than five decades, DARPA has been a leader in generating groundbreaking research and development (R&D) that facilitated the advancement and application of rule-based and statistical-learning based AI technologies. DARPA continues to lead innovation in AI research as it funds a broad portfolio of R&D programs, ranging from basic research to advanced technology development. DARPA believes this future, where systems are capable of acquiring new knowledge through generative contextual and explanatory models, will be realized upon the development and application of “Third Wave” AI technologies.
DARPA announced in September 2018 a multi-year investment of more than $2 billion in new and existing programs called the “AI Next” campaign. Key areas of the campaign include automating critical DoD business processes, such as security clearance vetting or accrediting software systems for operational deployment; improving the robustness and reliability of AI systems; enhancing the security and resiliency of machine learning and AI technologies; reducing power, data, and performance inefficiencies; and pioneering the next generation of AI algorithms and applications, such as “explainability” and common sense reasoning.
AI Next builds on DARPA‘s five decades of AI technology creation to define and to shape the future, always with the Department’s hardest problems in mind. Accordingly, DARPA will create powerful capabilities for the DoD by attending specifically to the following areas:
New Capabilities: AI technologies are applied routinely to enable DARPA R&D projects, including more than 60 exisiting programs, such as the Electronic Resurgence Initiative, and other programs related to real-time analysis of sophisticated cyber attacks, detection of fraudulent imagery, construction of dynamic kill-chains for all-domain warfare, human language technologies, multi-modality automatic target recognition, biomedical advances, and control of prosthetic limbs. DARPA will advance AI technologies to enable automation of critical Department business processes. One such process is the lengthy accreditation of software systems prior to operational deployment. Automating this accreditation process with known AI and other technologies now appears possible.
Robust AI: AI technologies have demonstrated great value to missions as diverse as space-based imagery analysis, cyberattack warning, supply chain logistics and analysis of microbiologic systems. At the same time, the failure modes of AI technologies are poorly understood. DARPA is working to address this shortfall, with focused R&D, both analytic and empirical. DARPA’s success is essential for the Department to deploy AI technologies, particularly to the tactical edge, where reliable performance is required.
Adversarial AI: The most powerful AI tool today is machine learning (ML). ML systems can be easily duped by changes to inputs that would never fool a human. The data used to train such systems can be corrupted. And, the software itself is vulnerable to cyber attack. These areas, and more, must be addressed at scale as more AI-enabled systems are operationally deployed.
High Performance AI: Computer performance increases over the last decade have enabled the success of machine learning, in combination with large data sets, and software libraries. More performance at lower electrical power is essential to allow both data center and tactical deployments. DARPA has demonstrated analog processing of AI algorithms with 1000x speedup and 1000x power efficiency over state-of-the-art digital processors, and is researching AI-specific hardware designs. DARPA is also attacking the current inefficiency of machine learning, by researching methods to drastically reduce requirements for labeled training data.
Next Generation AI: The machine learning algorithms that enable face recognition and self-driving vehicles were invented over 20 years ago. DARPA has taken the lead in pioneering research to develop the next generation of AI algorithms, which will transform computers from tools into problem-solving partners. DARPA research aims to enable AI systems to explain their actions, and to acquire and reason with common sense knowledge. DARPA R&D produced the first AI successes, such as expert systems and search, and more recently has advanced machine learning tools and hardware. DARPA is now creating the next wave of AI technologies that will enable the United States to maintain its technological edge in this critical area.
In addition to new and existing DARPA research, a key component of the campaign will be DARPA’s Artificial Intelligence Exploration (AIE) program, which was first announced in July 2018. AIE constitutes a series of high-risk, high payoff projects where researchers will work to establish the feasibility of new AI concepts within 18 months of award. Leveraging streamlined contracting procedures and funding mechanisms will enable these efforts to move from proposal to project kick-off within three months of an opportunity announcement. Forthcoming AIE Opportunities will be published on the FedBizOpps website under Program Announcement DARPA-PA-18-02.
The U.S. Army Research Laboratory, together with the Algorithmic Warfare Cross-Functional Team (AWCFT) and the Joint Artificial Intelligence Center (JAIC), hosted the 2nd Annual DoD AI Industry Day to discuss AI software prototyping activities and future industry participation. All technical businesses engaged in AI/ML were invited to submit their capabilities briefing to the DOD technical panel for consideration. This all-day event allowed businesses to engage their counterparts, create synergy and ask questions of the AI team. In support of AI prototyping, the AI Industry Day focused on the following five areas:
As the R&D arm of DHS, S&T focuses on providing the tools, technologies, and knowledge products for DHS operational components, state and local first responders, and the Homeland Security mission ensuring R&D coordination across the Department. S&T’s R&D focus areas cover DHS’s core mission areas and use our network of industry, national laboratories, international, academic and other partners to seek solutions for capability gaps and define topics for future research.
AI’s promise can be seen in the rapid proliferation of many applications across government and the private sector. From a government perspective, it holds the potential for enhanced insight into public service operations and improved delivery of services, including through anticipatory responsiveness to inquiries, discovery of new trends, and automation of internal processes. Examples of AI applications span the gamut from helping people navigate immigration systems, to predicting and pre-empting threats, to making critical infrastructure more resilient against increasing attacks. From the DHS S&T perspective, the future AI trajectory will proceed in the following three ways:
First, AI technology is increasingly providing us with new knowledge and informing our actions. Fueled by sensors, data digitization, and ever-increasing connectedness, AI filters, associates, prioritizes, classifies, measures, and predicts outcomes, allowing the Federal government to make more informed, data-driven decisions.
Second, algorithms are ingesting and processing ever higher volumes of data. Their complexity, especially in the case of deep learning algorithms, will continue to increase, and there is a need to better understand how outputs are produced from the set of inputs, which may not be able to be understood or analyzed in isolation.
Finally, private industry is leading the way in AI development, as many see the implementation of AI as a key competitive advantage. The private sector’s significant investments and the ability to adopt new AI models and processes faster than the public sector present the government with a key decision point on how to best participate in this growing, but still nascent field. Government should move forward with adoption of emerging technologies such as AI to improve citizen services. Government also plays an important role in promoting research and development. Government should ensure it is informed of developments in the private sector, while continuing to support AI research and development, and promote the use of AI technology to create government efficiencies and enhance the public good.
AI is an integral part of several S&T Cyber Security Division (CSD) research projects funded within current resources, which are using AI and machine learning techniques for a variety of purposes, including but not limited to predictive analysis for malware evolution; enabling defensive techniques to be established ahead of a future malware variant; detecting anomalous network traffic and behaviors to inform cyber defensive decision making; and helping identify, categorize and score various adversarial Telephony Denial of Service (TDoS) techniques.
A good example of S&T’s work involves demonstration of TDoS protection for a major US bank with a significant impact on its contact center that processes close to 11 million calls per week. The machine learning-based policy engine blocks more than 120,000 calls per month based on voice firewall policies including harassing callers, robocalls and potential fraudulent calls. It also blocks two to three phone-based attacks each month (computer-generation of calls into 1-800 toll free destinations in an attempt to collect a portion of the connection or per-minute charges associated with the call). This same technology can be used by 911 call centers to defend against denial of service attacks.
Another S&T research example capitalizes on the convergence of technologies such as machine learning, software defined networking, and global internet routing to help build more robust defenses against Distributed Denial of Service (DDoS) attacks. This specific application uses machine learning to create fine-grained, temporal traffic models that allow anomaly detection without preset thresholds and with low false positive rates. It then uses Software Defined Networking technology to deploy thousands of rules to instantly defend against complex DDoS attacks at very high speeds.
DHS S&T launched its Silicon Valley Innovation Program (SVIP) to keep pace with the innovation community and engage that community to tackle significant problems faced by the Department’s operational missions. SVIP expands DHS S&T’s reach to find new technologies that strengthen national security.
Through a streamlined application and pitch process leveraging Other Transaction Authority, SVIP is seeking solutions to challenges that range across the entire spectrum of the homeland security mission space, including cybersecurity and technology solutions for Customs and Border Protection (CBP) and first responders.
DHS SVIP and CBP are working together to evaluate and implement innovative methods -- to include the use of AI and machine learning -- to exchange information and intelligence, build capacity, and increase worldwide security and compliance standards in support of CBP and its international partners. These efforts widen border security capabilities and support a “defense in depth” approach to combat the global threat environment, and strengthen our combined enforcement efforts.
CBP offers advanced passenger data-screening and targeting technology as an open source software project, known as the Global Travel Assessment System or GTAS. It is a turn-key application that provides to CBP’s foreign counterpart agencies the necessary decision support system features to receive and store air traveler data, both Advanced Passenger Information (API) and Passenger Name Record (PNR), provide real-time risk assessment against this data based on a country’s own specific risk criteria and/or watch lists, and view high-risk travelers as well as their associated flight and reservation information. The purpose of GTAS is to provide border security entities the basic capacity to ingest, process, query, and construct risk criteria against the industry-derived standardized air traveler information. The system provides border security organizations with the necessary tools to prescreen travelers entering into and leaving their countries.
Last year, DHS SVIP and CBP partnered to enhance the GTAS project with solutions from the global innovation community, namely new capabilities using AI and machine learning, and identifying the following three capabilities for consideration:
VISUALIZATION: This would extend the basic flight and passenger tabular list screens with geospatial, link analysis, seat map visualization, or any other concepts that improve the software by presenting data graphically
PREDICTIVE MODELS: These would complement GTAS rules engine with statistical and machine learning models and a “predictive model engine” that performs real-time risk assessment and
ENTITY RESOLUTION: This capability would enhance the basic name/date of birth and document matching algorithms to support more advanced entity identification and matching algorithms.
AI and machine learning are rapidly moving from scientific understanding to engineering application in most domain areas. This reality means DHS must aggressively work with its research, development, test and evaluation partners throughout government and industry to develop effective, trusted homeland security applications of AI and machine learning.
One real value of AI is in replacing federal workers. Agencies face new choices about whether some work should be fully automated, divided among people and machines, or performed by people but enhanced by machines. AI-augmented government could free up 96.7 million federal government working hours annually, potentially saving $3.3 billion. At the high end estimates indicate that AI technology could free up as many as 1.2 billion working hours every year, saving $41.1 billion. The type of federal jobs that could be replaced by AI depends on five factors:
Based on these factors, the most susceptible types of jobs to be replaced by AI involve predictable physical work, data processing, and data collection. Jobs not currently ripe for AI automation (but may be soon) are unpredictable physical work or stakeholder interactions. The least susceptible job types are the ones where federal employees are applying expertise and/or managing others.
Therefore, it is not a binary question of the job being replaced by AI tools or not. Rather, the AI replacement/augmentation is a continuum. Jobs that are completely predictable and follow a well-defined process are most likely to be replaced by AI tools. Jobs that rely on judgment in an unpredictable environment while managing people could benefit from AI augmentation but will not be replaced by AI tools. You can see an analysis of 800+ occupations by the potential for automation. This analysis relies on the five factors to determine the probability for AI replacement. According to this analysis, administrative and government occupations face only a 31% chance for AI replacement which is the lowest of the service-providing industries.
An interesting trend in government that argues for the augmentation of federal workers rather than significant replacement is the need for agile government decision-making. According to a recent study, government decision-makers must operate in an increasingly volatile, uncertain, complex, and ambiguous (VUCA) environment. AI tools can help with the need for more sophisticated prediction abilities that help decision makers navigate the VUCA world. However, there is still the need for imagination, creativity, and innovation. Traits that AI tools have not yet demonstrated and may not develop for many years from now.
As Artificial Intelligence and Machine Learning technologies improve, the need for highly trained data scientists will only increase. Very soon, machines will have the ability to conduct more accurate analysis with even less data, but this will only be possible with expert statistical modeling and perfected algorithms created by data scientists. There will be an increase in need for individuals who can understand, design, and leverage these tools. They have broad applications in everything from business intelligence to consumer electronics to medical devices, but there is a lot of nuance to how they’re built, used, and validated.