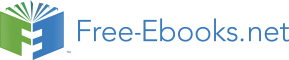

6.2.2
Quasiconvex Functionals
A functional on is quasiconvex if for each
, the func-
De
nition
6.5:
H
2
R
tional inequality specication
( )
is convex.
fH
j
H
g
An equivalent de nition of quasiconvexity, that has a form similar to the de -
nition of convexity, is: whenever , ~
and
0 1],
H
H
2
H
2
( + (1 ) ~) max ( ) ( ~)
H
;
H
f
H
H
g:
From de nition 6.4 we can see that every convex functional is quasiconvex.
The values of a quasiconvex functional along a line in is plotted in gure 6.4
H
note that this functional is not convex. A quasiconvex function of one variable is
called unimodal, since, roughly speaking, it cannot have two separate regions where
it is small.
~
(H
)
~ )
;
)H
;
;
(1;+
(H
)
(
;
H
;
=
0
=
1
@
;
@
R
;
A functional is quasiconvex if for every pair of transfer ma-
Figure
6.4
trices and ~ the graph of along the line
+(1 ) ~ lies on or below
H
H
H
;
H
the larger of ( ) and ( ~), i.e., in the shaded region.
H
H
A positive weighted maximum of quasiconvex functionals is quasiconvex, but a
positive-weighted sum of quasiconvex functionals need not be quasiconvex.
There is a natural correspondence between quasiconvex functionals and nested
families of convex speci cations, i.e., linearly ordered parametrized sets of speci ca-
tions. Given a quasiconvex functional , we have the family of functional inequality
speci cations given by
=
( )
. This family is linearly ordered:
H
fH
j
H
g
H
is stronger than
if
.
H
6.2 AFFINE AND CONVEX SETS AND FUNCTIONALS
133
Conversely, suppose we are given a family of convex speci cations , indexed
H
by a parameter
, such that
is stronger than
if
(so the family of
2
R
H
H
speci cations is linearly ordered). The functional
family( ) = inf
(6.1)
H
f
j
H
2
H
g
( family( ) = if
for all ) simply assigns to a transfer matrix the index
H
1
H
62
H
H
corresponding to the tightest speci cation that it satis es, as shown in gure 6.5.
This functional family is easily shown to be quasiconvex, and its sub-level sets are
essentially the original speci cations:
family( )
+
H
fH
j
H
g
H
for any positive .
(5)
(3)
(4) H
H
H
(2)
H
(1)
H
q
@
I
@
H
Five members of a nested family of convex sets are shown.
Figure
6.5
Such nested families dene a quasiconvex function by (6.1) for the given
transfer matrix , we have family( ) = 2.
H
H
6.2.3
Linear Transformations
Convex subsets of and convex functionals on are often de ned via linear trans-
H
H
formations. Suppose that is a vector space and :
is a linear function.
V
L
H
!
V
If is a convex (or a ne) subset of , then the subset of de ned by
V
V
H
1 =
( )
H
f
H
j
L
H
2
V
g
is convex (or a ne). Similarly if is a convex (or quasiconvex or a ne) functional
on , then the functional on de ned by
V
H
( ) = ( ( ))
H
L
H
is convex (or quasiconvex or a ne, respectively).
These facts are easily established from the de nitions above we mention a few
important examples.
134