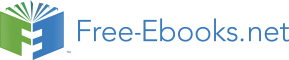

Setting β = 0, (4.3.78), (4.3.79) become
˙ θ = P φ
˙
P φφ P
P
= −
,
P (0) = P
m 2
0
(4.3.80)
In terms of the P − 1 we have
d
φφ
P − 1 =
dt
m 2
which implies that d( P − 1) ≥ 0, and, therefore, P − 1 may grow without bound.
dt
In the matrix case, this means that P may become arbitrarily small and slow
down adaptation in some directions. This is the so-called covariance wind-up
problem that constitutes one of the main drawbacks of the pure least-squares
algorithm.
Despite its deficiency, the pure least-squares algorithm has the unique
property of guaranteeing parameter convergence to constant values as de-
scribed by the following theorem:
Theorem 4.3.4 The pure least-squares algorithm (4.3.80) guarantees that
(i)
, ns, θ, ˙ θ, P ∈ L∞.
(ii)
, ns, ˙ θ ∈ L 2 .
(iii) lim t→∞ θ( t) = ¯
θ, where ¯
θ is a constant vector.
(iv) If ns, φ ∈ L∞ and φ is PE, then θ( t) converges to θ∗ as t → ∞.
Proof From (4.3.80) we have that ˙
P ≤ 0, i.e., P ( t) ≤ P 0. Because P ( t) is nonin-
creasing and bounded from below (i.e., P ( t) = P ( t) ≥ 0 , ∀t ≥ 0) it has a limit, i.e.,
lim P ( t) = ¯
P
t→∞
where ¯
P = ¯
P
≥ 0 is a constant matrix. Let us now consider
d
φφ ˜
θ
( P − 1 ˜
θ) = −P − 1 ˙
P P − 1 ˜
θ + P − 1 ˙˜
θ =
+ φ = 0
dt
m 2
where the last two equalities are obtained by using ˙ θ = ˙˜
θ, d P − 1 = −P − 1 ˙
P P − 1
dt
˜
and
= − θ φ = − φ ˜ θ. Hence, P − 1( t)˜
θ( t) = P − 1 ˜
θ(0), and, therefore, ˜
θ( t) =
m 2
m 2
0
P ( t) P − 1 ˜
˜
˜
0
θ(0) and lim t→∞ θ( t) = ¯
P P − 1
0
θ(0), which implies that lim t→∞ θ( t) = θ∗ +
¯
P P − 1 ˜
0
θ(0) = ¯
θ.
196
CHAPTER 4. ON-LINE PARAMETER ESTIMATION
Because P ( t) ≤ P
˜
0 and ˜
θ( t) = P ( t) P − 1
0
θ(0) we have θ, ˜
θ ∈ L∞, which, together
˜
with φ ∈ L
θ φ and , n
m
∞, implies that
m = − m
s ∈ L∞. Let us now consider the
function
˜
θ P − 1( t)˜
θ
V (˜
θ, t) =
2
The time derivative ˙
V of V along the solution of (4.3.80) is given by
˜
2
2
˙
θ φφ ˜
θ
m 2
m 2
V = ˜
θ φ +
= − 2 m 2 +
= −
≤ 0
2 m 2
2
2
which implies that V ∈ L∞, m ∈ L 2; therefore, , ns ∈ L 2. From (4.3.80) we have
|φ|
| ˙ θ| ≤ P
| m|
m
Because P, φ , m ∈ L
m
∞ and
m ∈ L 2, we have ˙ θ ∈ L∞
L 2, which completes the
proof for (i), (ii), and (iii). The proof of (iv) is given in Section 4.8.
✷
Remark 4.3.16
(i) We should note that the convergence rate of θ( t) to θ∗ in Theorem 4.3.4
is not guaranteed to be exponential even when φ is PE. As shown in the
proof of Theorem 4.3.4 (iv) in Section 4.8, P ( t), ˜
θ( t) satisfy
¯
m
P − 1
P ( t) ≤
I, |˜
θ( t) | ≤
0
¯
m |˜ θ(0) |, ∀t > T
( t − T
0
0) α 0
( t − T 0) α 0
where ¯
m = sup t m 2( t), i.e., |˜ θ( t) | is guaranteed to converge to zero with
a speed of 1 .
t
(ii) The convergence of θ( t) to ¯
θ as t → ∞ does not imply that ˙ θ( t) → 0 as
t → ∞ (see examples in Chapter 3).
(iii) We can establish that , ˙ θ → 0 as t → ∞ if we assume that ˙ φ/m, ˙
m/m ∈
L∞ as in the case of the gradient algorithm based on the instantaneous
cost.
Pure Least-Squares with Covariance Resetting
The so called wind-up problem of the pure least-squares algorithm is avoided
by using various modifications that prevent P ( t) from becoming singular.
4.3. ADAPTIVE LAWS WITH NORMALIZATION
197
One such modification is the so-called covariance resetting described by
˙ θ = P φ
˙
P φφ P
P
= −
,
P ( t+
m 2
r ) = P 0 = ρ 0 I
(4.3.81)
where tr is the time for which λmin( P ( t)) ≤ ρ 1 and ρ 0 > ρ 1 > 0 are some design scalars. Because of (4.3.81), P ( t) ≥ ρ 1 I ∀t ≥ 0; therefore, P is
guaranteed to be positive definite for all t ≥ 0.
Strictly speaking, (4.3.81) is no longer the least-squares algorithm that
we developed by setting ∇J( θ) = 0 and β = 0. It does, however, behave as
a pure least-squares algorithm between resetting points. The properties of
(4.3.81) are similar to those of the gradient algorithm based on the instan-
taneous cost. In fact, (4.3.81) may be viewed as a gradient algorithm with
time-varying adaptive gain P .
Theorem 4.3.5 The pure least-squares with covariance resetting algorithm
(4.3.81) has the following properties:
(i)
, ns, θ, ˙ θ ∈ L∞.
(ii)
, ns, ˙ θ ∈ L 2 .
(iii) If ns, φ ∈ L∞ and φ is PE then θ( t) converges exponentially to θ∗.
Proof The covariance matrix P ( t) has elements that are discontinuous functions
of time whose values between discontinuities are defined by the differential equation
(4.3.81). At the discontinuity or resetting point tr, P ( t+
r ) = P 0 = ρ 0 I ; therefore,
P − 1( t+
r ) = ρ− 1
0 I . Between discontinuities d P − 1( t) ≥ 0 , i.e., P − 1( t
dt
2) −P − 1( t 1) ≥ 0
∀t 2 ≥ t 1 ≥ 0 such that tr ∈ [ t 1 , t 2], which implies that P − 1( t) ≥ ρ− 1
0 I , ∀t ≥ 0.
Because of the resetting, P ( t) ≥ ρ 1 I, ∀t ≥ 0. Therefore, (4.3.81) guarantees that
ρ 0 I ≥ P ( t) ≥ ρ 1 I, ρ− 1
1 I ≥ P − 1( t) ≥ ρ− 1
0 I ,
∀t ≥ 0
Let us now consider the function
˜
θ P − 1 ˜
θ
V (˜
θ) =
(4.3.82)
2
where P is given by (4.3.81). Because P − 1 is a bounded positive definite symmetric
matrix, it follows that V is decrescent and radially unbounded in the space of ˜
θ.
Along the solution of (4.3.81) we have
˙
1
d( P − 1)
1
d( P − 1)
V =
˜
θ
˜
θ + ˜
θ P − 1 ˙˜
θ = − 2 m 2 + ˜
θ
˜
θ
2
dt
2
dt
198
CHAPTER 4. ON-LINE PARAMETER ESTIMATION
Between resetting points we have from (4.3.81) that d( P − 1) = φφ ; therefore,
dt
m 2
2
˙
1 (˜
θ φ)2
m 2
V = − 2 m 2 +
= −
≤ 0
(4.3.83)
2
m 2
2
∀t ∈ [ t 1 , t 2] where [ t 1 , t 2] is any interval in [0 , ∞) for which tr ∈ [ t 1 , t 2].
At the points of discontinuity of P , we have
1
V ( t+
˜
r ) − V ( tr) =
θ ( P − 1( t+
2
r ) − P − 1( tr)) ˜
θ
Because P − 1( t+
r ) = 1 I , P − 1( t
I, it follows that V ( t+
ρ
r) ≥ 1
r ) − V ( tr) ≤ 0, which
0
ρ 0
implies that V ≥ 0 is a nonincreasing function of time for all t ≥ 0. Hence, V ∈ L∞
and lim t→∞ V ( t) = V∞ < ∞. Because the points of discontinuities tr form a set
of measure zero, it follows from (4.3.83) that m,
∈ L 2. From V ∈ L∞ and
ρ− 1
1 I ≥ P − 1( t) ≥ ρ− 1
0 I we have ˜
θ ∈ L∞, which implies that , m ∈ L∞. Using
m ∈ L∞
L 2 and ρ 0 I ≥ P ≥ ρ 1 I we have ˙ θ ∈ L∞
L 2 and the proof of (i) and
(ii) is, therefore, complete.
The proof of (iii) is very similar to the proof of Theorem 4.3.2 (iii) and is
omitted.
✷
Modified Least-Squares with Forgetting Factor
When β > 0, the problem of P ( t) becoming arbitrarily small in some direc-
tions no longer exists. In this case, however, P ( t) may grow without bound
since ˙
P may satisfy ˙
P > 0 because βP > 0 and the fact that P φφ P is only
m 2
positive semidefinite.
One way to avoid this complication is to modify the least-squares algo-
rithm as follows:
˙ θ = P φ
˙
P
=
βP − P φφ P
if P ( t) ≤ R
m 2
0
(4.3.84)
0
otherwise
where P (0) = P 0 = P 0 > 0, P 0 ≤ R 0 and R 0 is a constant that serves as an upper bound for P . This modification guarantees that P ∈ L∞
and is referred to as the modified least-squares with forgetting factor. The
above algorithm guarantees the same properties as the pure least-squares
with covariance resetting given by Theorem 4.3.5. They can be established
4.3. ADAPTIVE LAWS WITH NORMALIZATION
199
by choosing the same Lyapunov-like function as in (4.3.82) and using the
identity d P − 1 = −P − 1 ˙
P P − 1 to establish
dt
dP − 1 = −βP− 1 + φφ
if
P ≤ R
m 2
0
dt
0
otherwise
where P − 1(0) = P − 1
0
, which leads to
˜
˙
− 2 m 2 − β θ P − 1 ˜
θ if
P ≤ R
V =
2
2
0
− 2 m 2
otherwise
2
Because ˙
V ≤ − 2 m 2 ≤ 0 and P ( t) is bounded and positive definite ∀t ≥ 0,
2
the rest of the analysis is exactly the same as in the proof of Theorem 4.3.5.
Least-Squares with Forgetting Factor and PE
The covariance modifications described above are not necessary when ns,
φ ∈ L∞ and φ is PE. The PE property of φ guarantees that over an interval of
time, the integral of −P φφ P is a negative definite matrix that counteracts
m 2
the effect of the positive definite term βP with β > 0 in the covariance
equation and guarantees that P ∈ L∞. This property is made precise by the
following corollary:
Corollary 4.3.2 If ns, φ ∈ L∞ and φ is PE then the recursive least-squares
algorithm with forgetting factor β > 0 given by (4.3.78) and (4.3.79) guar-
antees that P, P − 1 ∈ L∞ and that θ( t) converges exponentially to θ∗.
The proof is presented in Section 4.8.
The use of the recursive least-squares algorithm with forgetting factor
with φ ∈ L∞ and φ PE is appropriate in parameter estimation of stable
plants where parameter convergence is the main objective. We will address
such cases in Chapter 5.
Let us illustrate the design of a least-squares algorithm for the same
system considered in Example 4.3.1.
Example 4.3.4 The system
z = W ( s) A sin( ωt + ϕ)
200
CHAPTER 4. ON-LINE PARAMETER ESTIMATION
where A, ϕ are to be estimated on-line is rewritten in the form
z = θ∗ φ 0
where θ∗ = [ A 1 , A 2] , φ 0 = W ( s) φ, φ = [sin ωt, cos ωt] . The least-squares algorithm for estimating θ∗ is given by
˙ θ = P φ 0
˙
φ
P
= βP − P 0 φ 0 P,
P (0) = ρ
m 2
0 I
where = z−θ φ 0 , m 2 = 1 + φ
m 2
0 φ 0 and β ≥ 0, ρ 0 > 0 are design constants. Because
φ 0 is PE, no modifications are required. Let us simulate the above scheme when
A = 10 , ϕ = 16 ◦ = 0 . 279 rad , ω = 2 rad/sec , W ( s) = 2 . Figure 4.10 gives the s+2
time response of ˆ
A and ˆ
ϕ, the estimate of A and ϕ, respectively, for different values
of β. The simulation results indicate that the rate of convergence depends on the
choice of the forgetting factor β. Larger β leads to faster convergence of ˆ
A, ˆ
ϕ to
A = 10 , ϕ = 0 . 279, respectively.
4.3.7