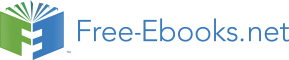

, ns ∈ L∞, θk ∈ ∞
618
CHAPTER 8. ROBUST ADAPTIVE LAWS
(ii) , m ∈ S( η 2 ) , ∆ θ
) where ∆ θ
m 2
k ∈ D( η 2
m 2
k = θk+1 − θk and
k 0+ N
t
k + N T
0
s
D( y) =
{x
x
y( τ ) dτ + c
k}
k xk ≤ c 0
1
k= k
tk
0
0
for some c 0 , c 1 ∈ R+ and any k 0 , N ∈ N + .
(iii) If ns, φ ∈ L∞ and φ is PE with a level of excitation α 0 > 0 that is
independent of η, then
(a) ˜
θk = θk − θ∗ converges exponentially to the residual set
D 0 = ˜
θ |˜
θ| ≤ c( σ 0 + ¯ η)
where c ≥ 0 , ¯
η = sup t | η |.
m
(b) There exists a constant ¯
η∗ > 0 such that if ¯
η < ¯
η∗, then ˜
θk
converges exponentially fast to the residual set
Dθ = ˜
θ |˜
θ| ≤ c¯
η
Proof Consider the function
V ( k) = ˜
θk Γ − 1 ˜ θk
(8.5.109)
Using ˜
θk+1 = ˜
θk + ∆ θk in (8.5.109), where ∆ θk = Γ tk+1( ( τ ) φ( τ) − w( τ) θ
t
k) dτ , we
k
can write
V ( k + 1) = V ( k) + 2˜
θk Γ − 1∆ θk + ∆ θk Γ − 1∆ θk
tk+1
= V ( k) + 2˜
θk
( ( τ ) φ( τ ) − w( τ ) θk) dτ
(8.5.110)
tk
tk+1
tk+1
+
( ( τ ) φ( τ ) −w( τ ) θk) dτΓ
( ( τ ) φ( τ ) −w( τ ) θk) dτ
tk
tk
Because ˜
θ φ = − m 2 + η, we have
k
tk+1
tk+1
tk+1 2
tk+1
˜
m 2
η 2
θk
( τ ) φ( τ ) dτ =
( − 2 m 2 + η) dτ ≤ −
dτ +
dτ
t
2
2 m 2
k
tk
tk
tk
(8.5.111)
8.5. ROBUST ADAPTIVE LAWS
619
where the last inequality is obtained by using the inequality −a 2 + ab ≤ − a 2 + b 2 .
2
2
Now consider the last term in (8.5.110), since
tk+1
tk+1
( ( τ ) φ( τ ) − w( τ ) θk) dτΓ
( ( τ ) φ( τ ) − w( τ ) θk) dτ
tk
tk
t
2
k+1
≤ λm
( ( τ ) φ( τ ) − w( τ ) θk) dτ
tk
where λm = λmax(Γ − 1), it follows from the inequality ( a + b)2 ≤ 2 a 2 + 2 b 2 that tk+1
tk+1
( ( τ ) φ( τ ) − w( τ ) θk) dτΓ
( ( τ ) φ( τ ) − w( τ ) θk) dτ
tk
tk
t
2
2
k+1
φ( τ )
tk+1
≤ 2 λm
( τ ) m( τ )
dτ
+ 2 λm
w( τ ) θkdτ
t
m( τ )
k
tk
tk+1
tk+1 |φ| 2
≤ 2 λ
2
m
( τ ) m 2( τ ) dτ
dτ + 2 λmσ 2 sT 2 s|θk| 2
t
m 2
k
tk
tk+1
≤ 2 λ
2
mTs
( τ ) m 2( τ ) dτ + 2 λmσ 2 sT 2 s|θk| 2
(8.5.112)
tk
In obtaining (8.5.112), we have used the Schwartz inequality and the assumption
|φ| ≤ 1. Using (8.5.111), (8.5.112) in (8.5.110), we have
m
tk+1
tk+1 |η| 2
V ( k + 1) ≤ V ( k) − (1 − 2 λ
2
mTs)
( τ ) m 2( τ ) dτ +
dτ
t
m 2
k
tk
− 2 σ
˜
sTsθk θk + 2 λmσ 2
s T 2
s |θk| 2
tk+1
≤ V ( k) − (1 − 2 λ
2
mTs)
( τ ) m 2( τ ) dτ + ¯
η 2 Ts
tk
− 2 σsTs(˜
θk θk − λmσ 0 Ts|θk| 2)
tk+1
≤ V ( k) − (1 − 2 λ
2
mTs)
( τ ) m 2( τ ) dτ + ¯
η 2 Ts
tk
1
|θ∗| 2
− 2 σsTs ( − λ
(8.5.113)
2
mσ 0 Ts) |θk| 2 −
2
where the last inequality is obtained by using ˜
θ θ
− |θ∗| 2 . Therefore, for
k
k ≥ |θk| 2
2
2
1 − 2 σ 0 λmTs > 0 and 1 − 2 λmTs > 0, it follows from (8.5.113) that V ( k + 1) ≤ V ( k) whenever
¯
η 2 + σ
|θ
0 |θ∗| 2
k| 2 ≥ max
M 20 , σ 0(1 − 2 λmσ 0 Ts)
Thus, we can conclude that V ( k) and θk ∈ ∞. The boundedness of , m follows
immediately from the definition of
and the normalizing properties of m, i.e.,
φ , η ∈ L
m m
∞.
620
CHAPTER 8. ROBUST ADAPTIVE LAWS
To establish (ii), we use the inequality
σ
σ
σ ˜
s
s
sθk θk
=
θ
θ
2 k θk + ( 2 k θk − σsθk θ∗)
σ
σ
≥
s |θ
s |θ
2
k| 2 + 2 k|( |θk| − 2 |θ∗|)
Because σs = 0 for |θk| ≤ M 0 and σs > 0 for |θk| ≥ M 0 ≥ 2 |θ∗|, we have σs|θk|( |θk|−
2 |θ∗|) ≥ 0 ∀k ≥ 0, therefore,
σ
σ ˜
s
sθk θk ≥
|θ
2
k| 2
which together with 2 σ 0 λmTs < 1 imply that
σs(˜
θk θk − λmσ 0 Ts|θk| 2) ≥ cσσs|θk| 2
(8.5.114)
where cσ = 1 − λ
2
mσ 0 Ts. From (8.5.114) and the second inequality of (8.5.113), we
have
tk+1
tk+1 η 2
(1 − 2 λ
2
mTs)
( τ ) m 2( τ ) dτ + cσσs|θk| 2 ≤ V ( k) − V ( k + 1) +
dτ
t
m 2
k
tk
(8.5.115)
√
which implies that m ∈ S( η 2 ) and
σ
). Because | | ≤ | m| (because
m 2
sθk ∈ D( η 2
m 2
m ≥ 1 , ∀t ≥ 0 ), we have ∈ S( η 2 ).
m 2
Note from (8.5.112) that ∆ θk satisfies
k 0+ N
k 0+ N
t
k
k+1
0+ N
(∆ θ
2
k) ∆ θk
≤ 2 λmTs
( τ ) m 2( τ ) dτ + 2 λmT 2 s
σ 2 s|θk| 2
k= k
tk
0
k= k 0
k= k 0
t
k
k
0+ N
0+ N
≤ 2 λ
2
mTs
( τ ) m 2( τ ) dτ +2 λmT 2 s
σ 2 s|θk| 2 (8.5.116)
tk 0
k= k 0
Using the properties that m ∈ S( η 2 ) , σ
), we have
m 2
sθk ∈ D( η 2
m 2
k 0+ N
tk 0+ N η 2
(∆ θk) ∆ θk ≤ c 1 + c 2
dτ
m 2
k= k
tk
0
0
for some constant c 1 , c 2 > 0. Thus, we conclude that ∆ θk ∈ D( η 2 ).
m 2
Following the same arguments we used in Sections 8.5.2 to 8.5.6 to prove pa-
rameter convergence, we can establish (iii) as follows: We have
tk+1
tk+1
˜
φ( τ ) φ ( τ )
φ( τ ) η( τ )
θk+1 = ˜
θk − Γ
dτ ˜
θk + Γ
dτ − Γ σsθkTs
t
m 2( τ )
m 2( τ )
k
tk
8.5. ROBUST ADAPTIVE LAWS
621
which we express in the form
˜
θk+1 = A( k)˜
θk + Bν( k)
(8.5.117)
where A( k) = I − Γ tk+1 φ( τ) φ ( τ) dτ , B = Γ, ν( k) =
tk+1 φ( τ) η( τ ) dτ − σ
t
sθkTs.
k
m 2( τ )
tk
m 2( τ )
We can establish parameter error convergence using the e.s. property of the homo-
geneous part of (8.5.117) when φ is PE. In Chapter 4, we have shown that φ being
PE implies that the equilibrium ˜
θe = 0 of ˜
θk+1 = A( k)˜
θk is e.s., i.e., the solution ˜
θk
of (8.5.117) satisfies
k
|˜
θk| ≤ β 0 γk + β 1
γk−i|νi|
(8.5.118)
i=0
for some 0 < γ < 1 and β 0 , β 1 > 0. Since |νi| ≤ c(¯
η + σ 0) for some constant c ≥ 0,
the proof of (iii) (a) follows from (8.5.118). From the definition of νk, we have
|νk| ≤ c 0 ¯
η + c 1 |σsθk| ≤ c 0 ¯
η + c 1 |σsθk| |˜ θk|
(8.5.119)
for some constants c 0 , c 1 , c 1, where the second inequality is obtained by using
˜
σ
θ θ
k
k
s|θk| ≤ σs
≤
1
|σ
M
sθk| | ˜
θk|. Using (8.5.119) in (8.5.118), we have
0 −|θ∗|
M 0 −|θ∗|
k− 1
|˜
θk| ≤ β 0 γk + β 1¯ η + β 2
γk−i| σsθi||˜
θi|
(8.5.120)
i=0
where β 1 = c 0 β 1 , β
1 −γ
2 = β 1 c 1.
To proceed with the parameter convergence analysis, we need the following
discrete version of the B-G Lemma.
Lemma 8.5.1 Let x( k) , f ( k) , g( k) be real valued sequences defined for k =
0 , 1 , 2 , . . . ,, and f ( k) , g( k) , x( k) ≥ 0 ∀k. If x( k) satisfies k− 1
x( k) ≤ f ( k) +
g( i) x( i) ,
k = 0 , 1 , 2 , . . .