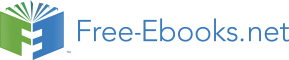

This session has explored the importance of a deep analytics based mechanism for cancer prevention in the context of human biological system. It presents a new framework of human biological system in terms of computing, data, networking, application and security schema of an information system based on analogical reasoning. DACPM promotes a hybrid approach which recognizes the role of both proactive and reactive approaches in making decisions on healthcare investment for cancer prevention. The reactive approach may outperform proactive one against the threats that never occur actually. Sometimes, reactive approach may be cost effective as compared to proactive approach. The basic building blocks of the proposed mechanism are threat analytics and adaptive secure multiparty computation. The threat analytics monitor the system performance of human biological system based on time series data, detects and analyzes different types of vulnerabilities on the biological system.
This work finds a set of interesting research agenda for future work: (a) explore new risk factors and causes of cancer, classifying cancers, opportunities for early detection and prevention and cost reduction of cancer care; (b) how to design an intelligent threat analytics; (c) how to design intelligent verification mechanisms; (d) how to rationalize DACPM, (e) how to quantify and code miscellaneous security intelligence parameters, (e) check the performance of kernel based learning algorithms with CNN, (g) how to apply integrated medicine for critical case (e.g. multiple organ failure syndrome) and exercise allopathic, homeopathy, herbal, yoga and naturopathy effectively for various purposes such as pain management, combating side effects of radiation and chemotherapy (e.g. hair fall, nausea, vomiting), every cancer patient requires specific treatment considering complexity of disease and (g) explore new approaches of cancer prevention such as vaccination for auto-immunity, laser therapy, integrated and regenerative medicine, precision medicine, gene therapy and stem cell therapy and (h) is it possible to imagine the security schema of human biological system based on antivirus, firewalls and various cryptographic tools (e.g. encryption, decryption, digital signature and signcryption) apart from secure multi-party computation? The next session explores the strategic option of bio-medical instrumentation and organ transplantation for various types of cancers such as pancreatic and liver cancer.
The expert panel are summarizing the outcome of deep analytic on the evaluation of today’s biotechnology technology. The diffusion of the technology is controlled by four factors: machine intelligence, security intelligence, collaborative intelligence and collective intelligence [Figure 5.5]. The machine intelligence considers a set of important criteria such as dominant design features, construction materials, system performance, biocompatibility and ease of use and deployment. It is essential to understand the fundamental principles, functions and mechanisms of the aforesaid biological organs through innovative experimental set up. The security intelligence considers safety, reliability, consistency, efficient surgical operation and reduced risk of infection. The design of biomedical devices must consider biological, chemical, mechanical, electrical and human factors of safety rationally. The collaborative intelligence demands proper integration and coordination among patient care chain, design chain and supply chain of biomedical engineering. The collective intelligence is determined by efficient demand, supply and capacity management of critical resources. Another critical success factor of technology diffusion is correctness and rationality of scope analytics. For example, oral insulin has more strength and opportunities of growth as compared to artificial pancreas. The technology of artificial kidney and liver should explore the hidden potential of tissue engineering. On the other side, the technology of artificial cardiovascular devices and limbs should explore the strength of mechanical and electrical engineering and mechatronics. It is also an interesting research agenda to explore the scope of living biological organ transplantation through organ donation, organ banks and alternative medicines (e.g. integrated medicine, regenerative medicine, precision medicine) as a part of proactive and reactive healthcare approaches. Finally, deep analytics can streamline the diffusion of biomedical technology through efficient coordination and integration among 7-S elements.
Figure 5.5: Technology diffusion of biomedical technology
This session presents artificial immune system mechanism (AIM). It is a new approach of epidemic and pandemic outbreak control. The basic building block of the mechanism is an analytics having multidimensional view of intelligent reasoning. The mechanism evaluates innate and adaptive system immunity in terms of collective, machine, security, collaborative and business intelligence. It is possible to redefine an efficient global healthcare policy for improved immunity and sustainable physical, mental and social health through reduction of artificial physical and mental stress. A biological system ensures optimal level of immunity by balancing natural and artificial intelligence (AI) based on intelligent reasoning. Effective control of epidemic and pandemic outbreak demands the innovation of smart biomedical devices such as non-contact infrared forehead thermometer. The digital thermal scanner is expected to have a set of important features such as capability to measure body temperature range with accuracy (e.g.0.30 C), 0C / 0F options, LCD backlight display, high or low body temperature indicator, high temperature alarm, auto power off after 15 seconds and warranty of one year.
The contribution of the present work is as follows. This novel concept of artificial immune mechanism has been applied to resolve the conflict between security intelligence and business intelligence for mitigating the risk of bio-terrorism today. The review of existing literature could not find out efficient mechanisms to counter bio-terrorism from the perspectives of security intelligence and business intelligence. The security intelligence has been defined weakly, incompletely and imprecisely. The system lacks intelligent model checking or system verification mechanisms based on rational threat analytics. The research methodology adopted in the present work includes case based reasoning, threat analytics and review of relevant literature on cryptography, secure multi-party computation and bio-terrorism. The logic of the bio-terrorism mechanism is explored through hypothetical case based reasoning on healthcare service chain, life-science supply chain, bio-technology and medical practice. The security intelligence is explored through threats analytics on malicious business intelligence.
A human agent must have common sense healthcare knowledge base for proper biological system control through intelligent self-assessment, self-confidence, life- style, diet control and right decision making at right time. It demands the necessity of learning the basic concept of AI reasoning, immunology and common sense healthcare from the childhood through an effective education and knowledge management system. It is not bio-inspired AI but the envision of AI inspired biological system which has a great potential to resolve the global healthcare problems significantly. AI has imagined the product concepts of highly complicated and costly artificial human organs such as artificial brain, heart, lungs, kidney, liver, pancreas, intestine, limbs, neural system, blood and cells though there are technological limitations and financial constraints of biomedical electronics, instrumentation, mechatronics, robotics, computational intelligence and materials science and also critical immunity issues. It is hard to develop and simulate artificial organs due to the inherent complexities of structure, material and mechanisms of the biological system. There are various risks of adaptive immunity and transmission of deadly diseases in artificial organ transplantation, artificial reproduction, common immunization programmes and breast milk feeding to the infants. Relaxation music, yoga and meditation is expected to be a good solution for the treatment of mental health though the modern entertainment world is getting flooded with ‘boom boom digital dhamaka’ and panic buttons through digital media and horror movies. AIM may be able to overcome some of those practically feasible constraints of the aforesaid imaginative reasoning through intelligent analytics and reasoning. AI community needs a new broad outlook, imagination and dreams to solve a complex problem through a set of simple mechanisms and solutions. Life is beautiful, let us apply AI for rational social choice to save the world.
B. W. Stewart, C. P. Wild, Eds., World Cancer Report 2014, IARC, France.
C.Tomasetti and B.Vogelstein. 2015. Cancer etiology. Variation in cancer risk among tissues can be explained by the number of stem cell divisions. Science, 347(6217):78–81.
A.Albini, F. Tosetti and VW Li. 2012. Cancer prevention by targeting angiogenesis. Nat Rev Clin Oncol. 9(9):498–509.
G. Anthes. 2013. Deep learning comes of age. Communications of the Association for Computing Machinery (ACM), 56(6):13–15.
I. Arel, C. Rose, and T. Karnowski. 2010. Deep machine learning — a new frontier in artificial intelligence. IEEE Computational Intelligence Magazine,5:13–18.
Y. Bengio. 2013. Deep learning of representations: Looking forward. In
Statistical Language and Speech Processing, pages 1–37. Springer.
L. Deng. 2011. An overview of deep-structured learning for information processing. In Proceedings of Asian-Pacific Signal & Information Processing Annual Summit and Conference (APSIPA-ASC). October 2011.
N. Nisan and A.Ronen. 1999. Algorithmic mechanism design. In 31st Annual ACM symposium on Theory of Computing, pp 129 -140.
S. Chakraborty. 2007. A study of several privacy preserving multi-party negotiation problems with applications to supply chain management. Indian Institute of Management Calcutta, India.
A.Barth, B.Rubinstein, M.Sundararajan, J.Mitchell, D.Song and P.L. Bartlett. 2010. A learning-based approach to reactive security. In: Radu, S. (ed.) Financial Cryptography’ 2010. LNCS, vol. 6052, pp. 192–206. Springer.
R.Bohme and T.W.Moore. 2009. The iterated weakest link: A model of adaptive security investment. In: Workshop on the Economics of Information Security (WEIS), University College, London, UK.
Y. Lindell. 2003. Composition of secure multi-party protocols a comprehensive study. Springer.
R.Canetti, U.Feige, O.Goldreich and M.Naor. 1996. Adaptively secure multi- party computation.
M.Kearns and M. Li. 1993. Learning in the presence of malicious errors. SIAM Journal on Computing 22(4), 807–837.
M.Barreno, B.Nelson, R. Sears, A.D. Joseph and J.D.Tygar. 2006. Can machine learning be secure? In Proceedings of the ACM symposium on Information, computer, and communications security.
S.Chakraborty. 2015. Secure multi-party computation: how to solve the conflict between security and business intelligence. Technical report.
S.K.Chaturvedi. 2012. Psychiatric oncology: cancer in mind. Indian Journal Psychiatry. Apr-Jun; 54(2): 111–118.
T.V.Borght, S. Asenbaum, P.Bartenstein, C.Halldin, Ö. Kapucu, K.V. Laere,
A. Varrone and K.Tatsch. 2010. Brain Tumor Imaging: European Association of Nuclear Medicine Procedure Guidelines.
M. Havaeia, A. Davyb, D. Warde-Farley, A. Biard, A. Courvillec, Y. Bengioc,
C. Pal, P.Jodoina and H. Larochelle. 2016. Brain Tumor Segmentation with Deep Neural Networks.
R.L.Siegel, K.D.Miller and A. Jemal. 2015. Cancer statistics. CA Cancer J Clin. 65(1):5–29.
American Cancer Society. 2015. Breast Cancer Facts and Figures 2015–2016: Atlanta: American Cancer Society, Inc.
D. Wang, A. Khosla, R. Gargeya, H.Irshad and A.B. Beck. 2016. Deep Learning for Identifying Metastatic Breast Cancer.
American Cancer Society. 2014.American Cancer Society: Cancer Facts and Figures 2014. Altanta, GA: American Cancer Society..
R.Siegel, D.Naishadham and A. Jemal. Cancer statistics, 2012. CA Cancer J Clin. 62(1):10-29.
S.Deuffic, et al. 1998. Trends in primary liver cancer. Lancet.
1998;351(9097):214-215.
R.Govindan, N. Page and D. Morgensztern. 2006. Changing epidemiology of small-cell lung cancer in the United States over the last 30 years: analysis of the surveillance, epidemiologic, and end results database. J Clin Oncol. 24(28):4539-4544. PMID: 17008692.
LC Caprario, DM Kent and GM Strauss. 2013. Effects of chemotherapyon survival of elderly patients with small-cell lung cancer: analysis of the SEER- medicare database. J Thorac Oncol. 8(10):1272-1281. PMID: 24457238. PMCID: 3901951.
F.Barbone, M. Bovenzi, F Cavallieri and G. Stanta. 1997. Cigarette smoking and histologic type of lung cancer in men. Chest. 112(6):1474-1479. PMID: 9404741.
S. Faderl S, S. O’Brien S and C-H Pui 2010. Adult acute lymphoblastic leukemia: concepts and strategies. Cancer. 116(5):1165-1176.
T.J. Lightfoot and E. Roman. 2004. Causes of childhood leukemia and lymphoma. Toxicol Appl Pharmacol. 199(2):104-117.
M. Murai and M. Oya. 2004. Renal cell carcinoma: etiology, incidence and epidemiology. Curr Opin Urol ;14: 229–33.
JD Mulder, HE Schütte, HM Kroon and W.K., Taconis. Radiologic atlas of bone tumors. 2nd edn. Amsterdam: Elsevier; 1993.
A.G. Huvos 1991. Bone tumors. Diagnosis, treatment, and prognosis. 2nd edn. Philadelphia: W.B. Saunders Company.
J.Ferlay, I.I.Soerjomataram and R. Dikshit 2015. Cancer incidence and mortality worldwide: sources, methods and major patterns in GLOBOCAN. Int J Cancer. 2015;136:E359–E386.doi:10.1002/ijc.29210.
BK Edwards, A-M Noone, AB Mariotto et al. 2014. Annual Report to the Nation on the status of cancer, 1975-2010, featuring prevalence of comorbidity and impact on survival among persons with lung, colorectal, breast, or prostate cancer. Cancer.120(9):1290–1314.
D. Hanahan , R.A. Weinberg, Hallmarks of cancer: the next generation, Cell 144 (5) (2011) 646–674 .
K. Kourou , T.P. Exarchos , K.P. Exarchos , M.V. Karamouzis , D.I. Fotiadis, Ma- chine learning applications in cancer prognosis and prediction, Comput. Struct. Biotechnol. J. 13 (2015) 8–17 .
E. Sayed , A. Wahed , I.A . Emam , A . Badr, Feature selection for cancer classifica- tion: an SVM based approach, Int. J. Comput. Appl. 46 (8) (2012) 20–26.
A. Statnikov , L. Wang , C.F. Aliferis , A comprehensive comparison of random forests and support vector machines for microarray-based cancer classification., BMC Bioinform. 9 (1) (2008) 1–10 .
S.B. Cho , H.H. Won , Machine learning in DNA microarray analysis for cancer classification, in: Asia-Pacific Bioinformatics Conference, 2003, pp. 189–198 .
H. Hijazi , C. Chan , A classification framework applied to cancer gene expression profiles, J. Healthc. Eng. 4 (4) (2012) 255–284.
N. C. F. Codella, Q. B. Nguyen, S. Pankanti, D. A. Gutman, B. Helba, A. C. Halpern, J. R. Smith, Deep learning ensembles for melanoma recognition in dermoscopy images, IBM Journal of Research and Development 61 (4) (2017) 5:1 – 5:15.
G. Karakoulas, J. Shawe-Taylor, Optimizing classifiers for imbalanced training sets, in: The Conference on Advances in Neural Information Processing Systems II, MIT Press, Cambridge, MA, USA, 1999, pp. 253– 259.
[42] R. Rasti, M. Teshnehlab, S. L. Phung, Breast cancer diagnosis in dce- mri using mixture ensemble of convolutional neural networks, Pattern Recognition 72 (2017) 381–390.
Nussinov, R. Advancements and Challenges in Computational Biology. PLoS Comput. Biol. 2015, 11 (1).
LeCun, Y.; Bengio, Y.; Hinton, G. Deep Learning. Nature 2015, 521 (7553), 436−444.
Schmidhuber, J. Deep Learning in Neural Networks: An Overview. Neural Networks 2015, 61, 85−117.
Fakoor, R.; Huber, M. Using Deep Learning to Enhance Cancer Diagnosis and Classification. In Proceeding 30th Int. Conf. Mach. Learn. Atlanta, GA, 2013, Vol. 28.
https://www.cancer.gov/about-cancer/treatment/types/surgery/lasers-fact- sheet
Lu YF, Goldstein DB, Angrist M, Cavalleri G (July 2014). "Personalized medicine and human genetic diversity". Cold Spring Harbor Perspectives in Medicine. 4 (9):
a008581. doi:10.1101/cshperspect.a008581. PMC 4143101. PMID 25059740.
"N-of-One: Tailored Clinical Molecular Test Interpretation". n-of-one.com.
Blau CA, Liakopoulou E (January 2013). "Can we deconstruct cancer, one patient at a time?". Trends in Genetics. 29 (1): 6–10. doi:10.1016/j.tig.2012.09.004. PMC 4221262. PMID 23102584.
Garraway LA, Verweij J, Ballman KV (May 2013). "Precision oncology: an overview". Journal of Clinical Oncology. 31 (15): 1803–5. doi:10.1200/jco.2013.49.4799. PMID 23589545.
Shrager J, Tenenbaum JM (February 2014). "Rapid learning for precision oncology". Nature Reviews. Clinical Oncology. 11 (2): 109–18. doi:10.1038/nrclinonc.2013.244. PMID 24445514.
Ashley EA (June 2015). "The precision medicine initiative: a new national effort". JAMA. 313 (21): 2119–20. doi:10.1001/jama.2015.3595. PMID 25928209.
Ashley EA (August 2016). "Towards precision medicine". Nature Reviews. Genetics. 17 (9): 507–22. doi:10.1038/nrg.2016.86. PMID 27528417.
Mesko B (2017). "Expert Review of Precision Medicine and Drug Development". Journal Expert Review of Precision Medicine and Drug Development. 2 (5): 239–241. doi:10.1080/23808993.2017.1380516.
Ray A. "Artificial Intelligence and Blockchain for Precision Medicine". Inner Light Publishers. Retrieved 21 May 2018.
Krittanawong C, Zhang H, Wang Z, Aydar M, Kitai T (May 2017). "Artificial Intelligence in Precision Cardiovascular Medicine". Journal of the American College of Cardiology. 69(21): 2657–2664. doi:10.1016/j.jacc.2017.03.571. PMID 28545640.
Mason, Chris; Dunnill, Peter (2008). "A brief definition of regenerative medicine". Regenerative Medicine. 3 (1): 1–5. doi:10.2217/17460751.3.1.1. ISSN 1746-0751. PMID 18154457.
Mahla RS (2016). "Stem cells application in regenerative medicine and disease threpeutics". International Journal of Cell Biology. 2016 (7): 1–24. doi:10.1155/2016/6940283. PMC 4969512. PMID 27516776.
Mason C; Dunnill P (January 2008). "A brief definition of regenerative medicine". Regenerative Medicine. 3 (1): 1–5. doi:10.2217/17460751.3.1.1. PMID 18154457.
Regenerative medicine glossary". Regenerative Medicine. 4 (4Suppl): S1–88. July 2009. doi:10.2217/rme.09.s1. PMID