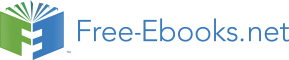

In this final installment of the paper we consider the case where the signals or the messages or both are
continuously variable, in contrast with the discrete nature assumed heretofore. To a considerable extent the
continuous case can be obtained through a limiting process from the discrete case by dividing the continuum
of messages and signals into a large but finite number of small regions and calculating the various parameters involved on a discrete basis. As the size of the regions is decreased these parameters in general approach as
limits the proper values for the continuous case. There are, however, a few new effects that appear and also
a general change of emphasis in the direction of specialization of the general results to particular cases.
We will not attempt, in the continuous case, to obtain our results with the greatest generality, or with
the extreme rigor of pure mathematics, since this would involve a great deal of abstract measure theory
and would obscure the main thread of the analysis. A preliminary study, however, indicates that the theory
can be formulated in a completely axiomatic and rigorous manner which includes both the continuous and
discrete cases and many others. The occasional liberties taken with limiting processes in the present analysis can be justified in all cases of practical interest.
18. SETS AND ENSEMBLES OF FUNCTIONS
We shall have to deal in the continuous case with sets of functions and ensembles of functions. A set of
functions, as the name implies, is merely a class or collection of functions, generally of one variable, time.
It can be specified by giving an explicit representation of the various functions in the set, or implicitly by giving a property which functions in the set possess and others do not. Some examples are:
1. The set of functions:
f t
sin t
=
+
:
Each particular value of
determines a particular function in the set.
2. The set of all functions of time containing no frequencies over W cycles per second.
3. The set of all functions limited in band to W and in amplitude to A.
4. The set of all English speech signals as functions of time.
An ensemble of functions is a set of functions together with a probability measure whereby we may
determine the probability of a function in the set having certain properties.1 For example with the set,
f t
sin t
=
+
;
we may give a probability distribution for , P
. The set then becomes an ensemble.
Some further examples of ensembles of functions are:
1. A finite set of functions fk t ( k
1 2
n) with the probability of fk being pk.
=
;
;
:
:
:
;
2. A finite dimensional family of functions
f
1
2
n; t
;
;
:
:
:
;
with a probability distribution on the parameters i:
p
1
n
;
:
:
:
;
:
For example we could consider the ensemble defined by
n
f a 1
an 1
n; t
∑ ai sin i t i
;
:
:
:
;
;
;
:
:
:
;
=
!
+
i 1
=
with the amplitudes ai distributed normally and independently, and the phases i distributed uniformly (from 0 to 2 ) and independently.
1In mathematical terminology the functions belong to a measure space whose total measure is unity.
32
3. The ensemble
∞
+
sin
2 W t
n
f a
,
i t
∑ an
;
=
2 W t
n
n
∞
,
=,
p
with the ai normal and independent all with the same standard deviation
N. This is a representation
of “white” noise, band limited to the band from 0 to W cycles per second and with average power N.2
4. Let points be distributed on the t axis according to a Poisson distribution. At each selected point the function f t is placed and the different functions added, giving the ensemble
∞
∑ f t tk
+
k
∞
=,
where the tk are the points of the Poisson distribution. This ensemble can be considered as a type of impulse or shot noise where all the impulses are identical.
5. The set of English speech functions with the probability measure given by the frequency of occurrence
in ordinary use.
An ensemble of functions f
t is stationary if the same ensemble results when all functions are shifted
any fixed amount in time. The ensemble
f t
sin t
=
+
is stationary if
is distributed uniformly from 0 to 2 . If we shift each function by t 1 we obtain
f t
t 1
sin t
t 1
+
=
+
+
sin t
=
+
'
with
distributed uniformly from 0 to 2 . Each function has changed but the ensemble as a whole is
'
invariant under the translation. The other examples given above are also stationary.
An ensemble is ergodic if it is stationary, and there is no subset of the functions in the set with a probability different from 0 and 1 which is stationary. The ensemble
sin t
+
is ergodic. No subset of these functions of probability
0 1 is transformed into itself under all time trans-
6=
;
lations. On the other hand the ensemble
a sin t
+
with a distributed normally and
uniform is stationary but not ergodic. The subset of these functions with
a between 0 and 1 for example is stationary.
Of the examples given, 3 and 4 are ergodic, and 5 may perhaps be considered so. If an ensemble is
ergodic we may say roughly that each function in the set is typical of the ensemble. More precisely it is
known that with an ergodic ensemble an average of any statistic over the ensemble is equal (with probability
1) to an average over the time translations of a particular function of the set.3 Roughly speaking, each
function can be expected, as time progresses, to go through, with the proper frequency, all the convolutions
of any of the functions in the set.
2This representation can be used as a definition of band limited white noise. It has certain advantages in that it involves fewer limiting operations than do definitions that have been used in the past. The name “white noise,” already firmly entrenched in the literature, is perhaps somewhat unfortunate. In optics white light means either any continuous spectrum as contrasted with a point spectrum, or a spectrum which is flat with wavelength (which is not the same as a spectrum flat with frequency).
3This is the famous ergodic theorem or rather one aspect of this theorem which was proved in somewhat different formulations by Birkoff, von Neumann, and Koopman, and subsequently generalized by Wiener, Hopf, Hurewicz and others. The literature on ergodic theory is quite extensive and the reader is referred to the papers of these writers for precise and general formulations; e.g., E. Hopf, “Ergodentheorie,” Ergebnisse der Mathematik und ihrer Grenzgebiete, v. 5; “On Causality Statistics and Probability,” Journal of Mathematics and Physics, v. XIII, No. 1, 1934; N. Wiener, “The Ergodic Theorem,” Duke Mathematical Journal, v. 5, 1939.
33
Just as we may perform various operations on numbers or functions to obtain new numbers or functions,
we can perform operations on ensembles to obtain new ensembles. Suppose, for example, we have an
ensemble of functions f
t and an operator T which gives for each function f
t a resulting function
g
t :
g
t
T f
t
=
:
Probability measure is defined for the set g
t by means of that for the set f
t . The probability of a certain
subset of the g
t functions is equal to that of the subset of the f
t functions which produce members of
the given subset of g functions under the operation T . Physically this corresponds to passing the ensemble through some device, for example, a filter, a rectifier or a modulator. The output functions of the device
form the ensemble g
t .
A device or operator T will be called invariant if shifting the input merely shifts the output, i.e., if g
t
T f
t
=
implies
g
t
t 1
T f
t
t 1
+
=
+
for all f
t and all t 1. It is easily shown (see Appendix 5 that if T is invariant and the input ensemble is stationary then the output ensemble is stationary. Likewise if the input is ergodic the output will also be
ergodic.
A filter or a rectifier is invariant under all time translations. The operation of modulation is not since the carrier phase gives a certain time structure. However, modulation is invariant under all translations which
are multiples of the period of the carrier.
Wiener has pointed out the intimate relation between the invariance of physical devices under time
translations and Fourier theory.4 He has shown, in fact, that if a device is linear as well as invariant Fourier analysis is then the appropriate mathematical tool for dealing with the problem.
An ensemble of functions is the appropriate mathematical representation of the messages produced by
a continuous source (for example, speech), of the signals produced by a transmitter, and of the perturbing
noise. Communication theory is properly concerned, as has been emphasized by Wiener, not with operations
on particular functions, but with operations on ensembles of functions. A communication system is designed
not for a particular speech function and still less for a sine wave, but for the ensemble of speech functions.
19. BAND LIMITED ENSEMBLES OF FUNCTIONS
If a function of time f t is limited to the band from 0 to W cycles per second it is completely determined by giving its ordinates at a series of discrete points spaced 1 seconds apart in the manner indicated by the
2 W
following result.5
Theorem 13: Let f t contain no frequencies over W . Then
∞
sin
2 W t
n
f t
∑ X ,
n
=
∞
2 W t
n
,
,
where
n
Xn
f
=
:
2 W
4Communication theory is heavily indebted to Wiener for much of its basic philosophy and theory. His classic NDRC report, The Interpolation, Extrapolation and Smoothing of Stationary Time Series (Wiley, 1949), contains the first clear-cut formulation of communication theory as a statistical problem, the study of operations on time series. This work, although chiefly concerned with the linear prediction and filtering problem, is an important collateral reference in connection with the present paper. We may also refer here to Wiener’s Cybernetics (Wiley, 1948), dealing with the general problems of communication and control.
5For a proof of this theorem and further discussion see the author’s paper “Communication in the Presence of Noise” published in the Proceedings of the Institute of Radio Engineers, v. 37, No. 1, Jan., 1949, pp. 10–21.
34
In this expansion f t is represented as a sum of orthogonal functions. The coefficients Xn of the various terms can be considered as coordinates in an infinite dimensional “function space.” In this space each
function corresponds to precisely one point and each point to one function.
A function can be considered to be substantially limited to a time T if all the ordinates Xn outside this interval of time are zero. In this case all but 2 TW of the coordinates will be zero. Thus functions limited to a band W and duration T correspond to points in a space of 2 TW dimensions.
A subset of the functions of band W and duration T corresponds to a region in this space. For example, the functions whose total energy is less than or equal to E correspond to points in a 2 TW dimensional sphere p
with radius r
2 W E.
=
An ensemble of functions of limited duration and band will be represented by a probability distribution p x 1
xn in the corresponding n dimensional space. If the ensemble is not limited in time we can consider
;
:
:
:
;
the 2 TW coordinates in a given interval T to represent substantially the part of the function in the interval T
and the probability distribution p x 1
xn to give the statistical structure of the ensemble for intervals of
;
:
:
:
;
that duration.
20. ENTROPY OF A CONTINUOUS DISTRIBUTION
The entropy of a discrete set of probabilities p 1
pn has been defined as:
;
:
:
:
;
H
∑ pi log pi
=
,
:
In an analogous manner we define the entropy of a continuous distribution with the density distribution
function p x by:
Z
∞
H
p x log p x dx
=
,
:
∞
,
With an n dimensional distribution p x 1
xn we have
;
:
:
:
;
Z
Z
H
p x 1
xn log p x 1
xn dx 1
dxn
=
,
;
:
:
:
;
;
:
:
:
;
:
If we have two arguments x and y (which may themselves be multidimensional) the joint and conditional entropies of p x y are given by
;
Z Z
H x y
p x y log p x y dx dy
;
=
,
;
;
and
Z Z
p x y
;
Hx y
p x y log
dx dy
=
,
;
p x
Z Z
p x y
H
;
y x
p x y log
dx dy
=
,
;
p y
where
Z
p x
p x y dy
=
;
Z
p y
p x y dx
=
;
:
The entropies of continuous distributions have most (but not all) of the properties of the discrete case.
In particular we have the following:
1. If x is limited to a certain volume v in its space, then H x is a maximum and equal to log v when p x is constant (1 v) in the volume.
=
35
2. With any two variables x, y we have
H x y
H x
H y
;
+
with equality if (and only if) x and y are independent, i.e., p x y
p x p y (apart possibly from a
;
=
set of points of probability zero).
3. Consider a generalized averaging operation of the following type:
Z
p 0 y
a x y p x dx
=
;