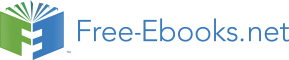

1
2
3
4
5
6
0
1
2
3
4
5
6
Time [ s ]
Time [ s ]
ror
10
ror 0.2
]
ation er
0
ation er b ]
0
tim ad/s[ r
W
tim [
-10
es -0.2
0
1
2
3
4
5
6
peed es
lux
0
1
2
3
4
5
6
S
Time [ s ]
F
Time [ s ]
( a )
( b )
b ]
b ] 0.2
[ W 0.2
[ W
0
0
ψ rβ
-0.2
ated ψ rβ -0.2
ctualA
tim
-0.4
-0.4
-0.4
-0.3
-0.2
-0.1
0
0.1
0.2
0.3
0.4
Es
-0.4
-0.3
-0.2
-0.1
0
0.1
0.2
0.3
0.4
Actual ψ [ Wb ]
Estimated ψ [ Wb ]
rα
rα
( c )
( d )
Fig. 18. Drive response on application and removal of load
4. Conclusion and future works
In this chapter we have presented some methods of sensorless vector control of induction
motor drive using machine model-based estimation. Sensorless vector control is an active
research area and the treatment of the whole model based sensorless vector control will
demand a book by itself.
First, a speed estimation algorithm in vector controlled induction motor drive has been
presented. The proposed method is based on observing a newly defined quantity which is a
function of rotor flux and speed. The algorithm uses command flux for speed computation.
The problem of decrease in estimation accuracy with the decrease in speed was overcome
using a flux observer based on voltage model of the machine along with the observer of the
newly defined quantity, and satisfactory results were obtained.
Then, a joint rotor flux and speed estimation algorithm has been presented. The proposed
method is based on a modified Blaschke equation and on observing the newly defined
quantity mentioned above. Good estimation accuracy was obtained and the response of the
sensorless vector controlled drive was found to be satisfactory.
The mathematical model of the motor used for implementing the estimation algorithm was
derived with the assumption that the rotor speed dynamics is much slower than that of
electrical states. Therefore, increase in estimation accuracy of the proposed algorithms will
be observed with the increase in the size of the machine used.
The machine model developed in this chapter may be used in future for machine parameter
estimation. The newly defined quantity presented in this chapter contains rotor resistance
information as well, in addition to that of rotor flux and speed. Therefore, future research
efforts may be made towards developing rotor resistance estimation algorithm using the
94
Electric Machines and Drives
new machine model. Further, in the proposed algorithms rotor flux was necessary for speed
estimation. Future research efforts may also be made towards developing a speed
estimation algorithm for which the knowledge of rotor flux is not necessary.
5. References
Abbondante, A. & Brennen, M. B. (1975). Variable speed induction motor drives use
electronic slip calculator based on motor voltages and currents. IEEE Trans. Ind.
Appl, Vol. 1A-11, No. 5, Sept/Oct, pp. 483-488.
Ben-Brahim, L. & Kudor, T. (1995). Implementation of an induction motor speed estimator
using neural networks. Proceedings of International Power Electronics Conference, IPEC
1995, Yokohama, April, pp. 52-58.
Bodson, M.; Chiasson, J. & Novotnak, R. T. (1995). Nonlinear Speed Observer for High
Performance Induction Motor Control. IEEE Trans. Ind. Elec, Vol. 42, No. 4, Aug.
pp. 337-343.
Choy, I.; Kwon, S. H.; Lim J. & Hong, S. W. (1996). Robust Speed Estimation for Tacholess
Induction Motor Drives. IEEE Electronics Letters, Vol. 32, No. 19, pp. 1836-1838.
Comnac V.; Cernat M.; Cotorogea, M. & Draghici, I. (2001). Sensorless Direct Torque and
Stator Flux Control of Induction Machines Using an Extended Kalman Filter",
Proceedings of IEEE Int. Conf. on Control Appl, Mexico, Sept. 5-7, pp. 674-679.
Du T.; Vas, P. & Stronach, F. (1995). Design and Application of Extended Observers for Joint
State and Parameter Estimation in High Performance AC Drives. IEE Proc. Elec.
Power Appl., Vol. 142, No. 2, pp. 71-78.
Fodor, D. ; Ionescu, F. ; Floricau, D. ; Six, J.P. ; Delarue, P. ; Diana, D. & Griva, G. (1995).
Neural Networks Applied for Induction Motor Speed Sensorless Estimation.
Proceedings of the IEEE International Symposium on Industrial Electronics, ISIE’ 95, July
10-14, Athens, pp. 181-186.
Gopinath, B. (1971). On the Control of Linear Multiple Input-Output Systems. Bell System
Technical Journal, Vol. 50, No. 3, March, pp. 1063-1081.
Haghgoeian, F.; Ouhrouche, M. & Thongam, J. S. (2005). MRAS-based speed estimation for
an induction motor sensorless drive using neural networks. WSEAS Transactions on
Systems, Vol. 4, No. 12, December, pp. 2346-2352.
Jansen, P. L. & Lorenz, R. D. (1994). A physically insightful approach to the design and
accuracy assessment of flux observers for field oriented induction machine drives.
IEEE Trans. Ind. App., Vol. 30, No. l, Jan. /Feb., pp. 101-110.
Kim, S. H.; Park, T. S.; Yoo, J. Y. & Park, G. T. (2001). Speed-sensorless vector control of an
induction motor using neural network speed estimation. IEEE Trans. Ind. Elec, Vol.
48, No. 3, June, pp. 609-614.
Kim, Y. R.; Sul S. K. & and Park, M. H. (1994). Speed sensorless vector control of induction
motor using extended Kalman filter. IEEE Trans. Ind. Appl., Vol. 30, No. 5,
Sept/Oct, pp. 1225-1233.
Kubota, H.; Matsuse K. & Nakano, T. (1993). DSP-based speed adaptative flux observer of
induction motor. IEEE Trans. Ind. Appl, Vol. 29, No. 2, March/April, pp. 344-348.
Liu, J. J.; Kung, I. C. & Chao, H. C. (2001). Speed estimation of induction motor using a non-
linear identification technique. Proc. Natl. Sci. Counc. ROC (A), Vol. 25, No. 2, pp.
107-114.
Sensorless Vector Control of Induction Motor Drive - A Model Based Approach
95
Ma, X. & Gui, Y. (2002). Extended Kalman filter for speed sensor-less DTC based on DSP.
Proc. of the 4th World Cong. on Intelligent Control and Automation, Shanghai, China,
June 10-14, pp. 119-122.
Minami, K.; Veley-Reyez, M.; Elten, D.; Verghese, G. C. & Filbert, D. (1991). Multi-stage
speed and parameter estimation for induction machines. Proceedings of the IEEE
Power Electronics Specialists Conf., Boston, USA, pp. 596-604.
Ohtani, T.; Takada, N. & and Tanaka, K. (1992). Vector control of induction motor without
shaft encoder. IEEE Trans. Ind. Appl, Vol. 28, No. 1, Jan/Feb, pp. 157-164.
Pappano, V.; Lyshevski, S. E. & Friedland, B. (1998). Identification of induction motor
parameters. Proceedings of the 37th IEEE Conf. on Decision and Control, Tampa,
Florida, USA, December 16-18, pp. 989-994.
Peng, F. Z. & Fukao, T. (1994). Robust speed identification for speed sensorless vector
control of induction motors. IEEE Trans. Ind. Appl, Vol. 30, No. 5, Sept/Oct., pp.
1234-1240.
Rowan, T. M. & Kerkman, R. J. (1986). A new synchronous current regulator and an analysis
of current-regulated PWM inverters. IEEE Trans. Ind. Appl, Vol. IA-22, No. 4,
July/Aug., pp. 678-690.
Schauder, C. (1992). Adaptive speed identification for vector control of induction motors
without rotational transducers. IEEE Trans. Ind. Appl, Vol. 28, No. 5, Sept./Oct., pp.
1054-1061.
Sathiakumar, S. (2000). Dynamic flux observer for induction motor speed control.
Proceedings of Australian Universities Power Engineering Conf. AUPEC 2000, Brisbane,
Australia, 24-27 Sept., pp. 108-113.
Simoes, M. G. & Bose, B. K. (1995). Neural network based estimation of feedback signals for
a vector controlled induction motor drive. IEEE Trans. Ind. Appl., Vol. 31,
May/June, pp. 620-629.
Tajima, H. & Hori, Y. (1993). Speed sensorless field-orientation control of the induction
machine. IEEE Trans. Ind. Appl., Vol. 29, No. 1, pp. 175-180.
Thongam, J. S. & Thoudam, V. P. S. (2004). Stator flux based speed estimation of induction
motor drive using EKF. IETE Journal of Research, India, Vol. 50, No. 3. May-June, pp
191-197.
Thongam, J. S. & Ouhrouche, M. (2006). Flux estimation for speed sensorless rotor flux
oriented controlled induction motor drive. WSEAS Transactions on Systems, Vol. 5,
No. 1, Jan., pp. 63-69.
Thongam, J. S. & Ouhrouche, M. (2007). A novel speed estimation algorithm in indirect
vector controlled induction motor drive. International Journal of Power and Energy
Systems, Vol. 27, No. 3, 2007, pp. 294-298.
Toqeer, R. S. & Bayindir, N. S. (2003). Speed estimation of an induction motor using Elman
neural network. Neuro Computing, Volume 55, Issues 3-4, October, pp. 727- 730.
Velez-Reyes, M.; Minami, K. & Verghese, G. C. (1989). Recursive speed and parameter
estimation for induction machines", IEEE/IAS Ann. Meet. Conf. Rec., San Diego, pp.
607-611.
Veleyez-Reyes, M. & Verghese, G. C. (1992). Decomposed algorithms for speed and
parameter estimation in induction machines. IFAC Symposium on Nonlinear Control
System Design, Bordeaux, France, pp. 77-82.
96
Electric Machines and Drives
Verghese, G. C. & Sanders, S. R. (1988). Observers for flux estimation in induction machines.
IEEE Trans. Ind. Elec, Vol. 35, No. 1, Feb., pp. 85-94.
Yan, Z.; Jin C. & Utkin, V. I. (2000). Sensorless sliding-mode control of induction motors.
IEEE Trans. Ind. Elec, Vol. 47, No. 6, Dec., pp. 1286-1297.
6
Feedback Linearization of Speed-Sensorless
Induction Motor Control with Torque
Compensation
Cristiane Cauduro Gastaldini1, Rodrigo Zelir Azzolin2,
Rodrigo Padilha Vieira3 and Hilton Abílio Gründling4
1,2,3,4 Federal University of Santa Maria
2 Federal University of Rio Grande
3 Federal University of Pampa
Brazil
1. Introduction
This chapter addresses the problem of controlling a three-phase Induction Motor (IM) without
mechanical sensor (i.e. speed, position or torque measurements). The elimination of the
mechanical sensor is an important advent in the field of low and medium IM servomechanism;
such as belt conveyors, cranes, electric vehicles, pumps, fans, etc. The absence of this sensor
(speed, position or torque) reduces cost and size, and increases reliability of the overall
system. Furthermore, these sensors are often difficult to install in certain applications and
are susceptible to electromagnetic interference. In fact, sensorless servomechanism may
substitute a measure value by an estimated one without deteriorating the drive dynamic
performance especially under uncertain load torque.
Many approaches for IM sensorless servomechanism have been proposed in the literature
is related to vector-controlled methodologies.
One of the proposed nonlinear control
methodologies is based on Feedback Linearization Control (FLC), as first introduced by
(Marino et al., 1990). FLC provides rotor speed regulation, rotor flux amplitude decoupling
and torque compensation. Although the strategy presented by (Marino et al., 1990) was not
a sensorless control strategy, fundamental principles of FLC follow servomechanism design
of sensorless control strategies, such as (Gastaldini & Grundling, 2009; Marino et al., 2004;
Montanari et al., 2007; 2006).
The purpose of this chapter is to present the development of two FLC control strategies in the
presence of torque disturbance or load variation, especially under low rotor speed conditions.
Both control strategies are easily implemented in fixed point DSP, such as TMS320F2812 used
on real time experiments and can be easily reproduced in the industry. Furthermore, an
analysis comparing the implementation and the limitation of both strategies is presented. In
order to implement the control law, these algorithms made use of only two-phase IM stator
currents measurement. The values of rotor speed and load torque states used in the control
algorithm are estimated using a Model Reference Adaptive System (MRAS) (Peng & Fukao,
1994) and a Kalman filter (Cardoso & Gründling, 2009), respectively.
This chapter is organized as follows: Section 2 presents the fifth-order IM mathematical
model. Section 3 introduces the feedback linearization modelling of IM control. A simplified
98
Electric Machines and Drives
FLC control strategy is described in Section 4. The proposed methods for speed and torque
estimation, MRAS and Kalman filter algorithms, respectively, are developed in Sections 5 and
6. State variable filter is used to obtain derivative signals necessary for implementation of the
control algorithm, and this is presented in section 7. Digital implementation in fixed point
DSP TMS320F2812 and real time experimental results are given in Section 8. Finally, Section 9
presents the conclusions.
2. Induction motor mathematical model
A three-phase N pole pair induction motor is expressed in an equivalent two-phase model in
an arbitrary rotating reference frame (q-d), according to (Krause, 1986) and (Leonhard, 1996)
according to the fifth-order model, as
d I
dt qs = −a 12 Iqs − ωs Ids + a 13 a 11 λqr − a 13 Nωλdr + a 14 Vqs (1)
d I
dt ds = −a 12 Ids + ωs Iqs + a 13 a 11 λdr + a 13 Nωλqr + a 14 Vds (2)
d λ
dt qr = −a 11 λqr − ( ωs − Nω) λdr + a 11 Lm Iqs
(3)
d λ
dt dr = −a 11 λdr + ( ωs − Nω) λqr + a 11 Lm Ids
(4)
d ω = μ · λ
ω − TL
(5)
dt
dr Iqs − λqr Ids − B
J
J
Te = μ · J · λdr Iqs − λqr Ids
(6)
In equations (1)-(6): Is = Iqs, Ids , λr = λqr, λdr and Vs = Vqs, Vds denote stator current, rotor flux and stator voltage vectors, where subscripts d and q stand for vector components in
(q-d) reference frame; ω is the rotor speed, the load torque TL, electric torque Te and ωs is the stationary speed, θ 0 is the angular position of the (q-d) reference frame with respect to a fixed stator reference frame ( α- β) , where physical variables are defined. Transformed variables related to three-phase (RST) system are given by
x αβ = K · xRST
(7)
Let
xqd = e jθ 0x αβ
(8)
⎡
⎤
⎢ 1
− 1
− 1 ⎥
with e jθ 0 =
cos θ 0
− sin θ 0
and K =
2 ⎣
2
√
2
√ ⎦.
sin θ 0
cos θ 0
3
3
3
0
−
−
2
2
xqd and x αβ stand for two-dimensional voltage flux and stator current vector, respectively on (q-d) and ( α- β)reference frame.
The relations between mechanical and electrical parameters in the above equations are
Δ
Δ
Δ
R
Δ
Δ
a
s
0 = Ls Lr − L 2 m, a 11 = Rr , a
= LsLr
+ L 2 m a
= Lm , a = Lr and μ Δ= NLm ;
L
12
11
, a 13
14
r
a 0 Ls
a 0
a 0
a 0
JLr
Feedback Linearization of Speed-Sensorless Induction Motor Control with Torque Compensation
99
where Rs, Rr, Ls and Lr are the stator/rotor resistances and inductances, Lm is the magnetizing inductance, J is the rotor inertia, B is the viscous coefficient and N is the number of pole pairs.
In the control design, the viscous coefficient of (5) is considered to be approximately zero, i.e.
B ≈ 0.
3. Feedback Linearization Control
The feedback Linearization Control (FLC) general specifications are two outputs - rotor speed
and rotor flux modulus, as
T Δ
y1 =
ω
λ 2 qr + λ 2
= ω |λ
dr
r |
T
(9)
which is controlled by two-dimensional stator voltage vector Vs , on the basis of measured
variables vector y2 = Is. The development concept of this control strategy is completely
described in (Marino et al., 1990) and it will be omitted here. Following the concept of indirect
field orientation developed by Blaschke, (Krause, 1986) and (Leonhard, 1996), the purpose of
FLC control is to align rotor flux vector with the d-axis reference frame, i.e.
λdr = |λr