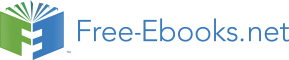

AGRICULTURAL SCIENCE
Edited by Godwin Aflakpui
Agricultural Science
Edited by Godwin Aflakpui
Published by InTech
Janeza Trdine 9, 51000 Rijeka, Croatia
Copyright © 2012 InTech
All chapters are Open Access distributed under the Creative Commons Attribution 3.0
license, which allows users to download, copy and build upon published articles even for
commercial purposes, as long as the author and publisher are properly credited, which
ensures maximum dissemination and a wider impact of our publications. After this work
has been published by InTech, authors have the right to republish it, in whole or part, in any publication of which they are the author, and to make other personal use of the
work. Any republication, referencing or personal use of the work must explicitly identify the original source.
As for readers, this license allows users to download, copy and build upon published
chapters even for commercial purposes, as long as the author and publisher are properly
credited, which ensures maximum dissemination and a wider impact of our publications.
Notice
Statements and opinions expressed in the chapters are these of the individual contributors and not necessarily those of the editors or publisher. No responsibility is accepted for the accuracy of information contained in the published chapters. The publisher assumes no
responsibility for any damage or injury to persons or property arising out of the use of any materials, instructions, methods or ideas contained in the book.
Publishing Process Manager Jana Sertic
Technical Editor Teodora Smiljanic
Cover Designer InTech Design Team
First published April, 2012
Printed in Croatia
A free online edition of this book is available at www.intechopen.com
Additional hard copies can be obtained from orders@intechopen.com
Agricultural Science, Edited by Godwin Aflakpui
p. cm.
ISBN 978-953-51-0567-1
Contents
Preface IX
Section 1
Crop Improvement 1
Chapter 1
Impact of Epistasis in
Inheritance of Quantitative Traits in Crops 3
Bnejdi Fethi and El Gazzeh Mohamed
Chapter 2
Genetic Diversity Analysis of
Heliconia psittacorum Cultivars and Interspecific
Hybrids Using Nuclear and Chloroplast DNA Regions 11
Walma Nogueira Ramos Guimarães,
Gabriela de Morais Guerra Ferraz,
Luiza Suely Semen Martins, Luciane Vilela Resende,
Helio Almeida Burity and Vivian Loges
Section 2
Crop Production 23
Chapter 3
Concepts in Crop Rotations 25
H. Arnold Bruns
Chapter 4
Texture, Color and Frequential Proxy-Detection
Image Processing for Crop Characterization in a
Context of Precision Agriculture 49
Cointault Frédéric, Journaux Ludovic, Rabatel Gilles,
Germain Christian, Ooms David, Destain Marie-France,
Gorretta Nathalie, Grenier Gilbert, Lavialle Olivier and
Marin Ambroise
Section 3
Crop Response to Water and Nutrients 71
Chapter 5
Spatial Patterns of Water and
Nitrogen Response Within Corn Production Fields 73
Jerry L. Hatfield
Chapter 6
Long-Term Mineral Fertilization and Soil Fertility 97
Margarita Nankova
VI
Contents
Chapter 7
Effect of Mixed Amino Acids on Crop Growth 119
Xing-Quan Liu and Kyu-Seung Lee
Section 4
Crop Response to Temperature 159
Chapter 8
Plant Temperature for Sterile Alteration of Rice 161
Chuan-Gen Lű
Section 5
Crop Protection 183
Chapter 9
Infrared Spectroscopy Applied to
Identification and Detection of Microorganisms and
Their Metabolites on Cereals (Corn, Wheat, and Barley) 185
Cécile Levasseur-Garcia
Chapter 10
Insect Pests of Green Gram
Vigna radiata (L.) Wilczek and Their Management 197
R. Swaminathan, Kan Singh and V. Nepalia
Section 6
Agriculture and Human Health 223
Chapter 11
The Agricultural Landscape for Recreation 225
Erik Skärbäck, John Wadbro, Jonas Björk,
Kim de Jong, Maria Albin, Jonas Ardö and Patrik Grahn
Section 7
Animal Nutrition 243
Chapter 12
Performance and Heat Index of
West African Dwarf (WAD) Rams Fed with
Adansonia digitata Bark (Baobab) as Supplement 245
Idayat Odunola Agboola
Preface
The whole world needs agriculture because agriculture does not only feed the entire
human race but also produces fibre for clothing, feed for livestock and bio-energy. In
the developing world agriculture contributes significantly to the gross domestic
product, leads the way in reducing poverty and accounts for the lion’s share of
employment opportunities especially for women. Agriculture also has one of the
highest potentials for reducing carbon emissions and helping vulnerable people adapt
to climate change.
The Food and Agriculture Organisation (FAO) of the United Nations and the World
Bank have indicated that:
100% of the global population depends on agriculture for nutrition
40% of the global population relies on agriculture for employment
70% of worldwide withdrawal of water is for the purposes of agriculture
30% proportion of greenhouse gas emissions is related to agriculture
70% increment in food production is needed to feed a global population of 9
billion by 2050.
These figures by the FAO and the World Bank indicate that without agriculture, the
world economy would not be what it has been and what it is today.
For agriculture to continuously contribute to food security, environmental
sustainability and economic opportunities by driving the rural and national economic
development with well targeted investments, it is imperative that the research and
development agenda which is based on the science behind agriculture must be
pursued vigorously. It is in this context that this book, Agricultural Science has been
written with multiple authors compiling some important state-of-the art contributions
on the subject in recent years.
The contributions of chapters in the book are divided into seven sections: Crop
Improvement, Crop Production, Crop Response to Water and Nutrients, Crop
Response to Temperature, Crop Protection, Agriculture and Human Health, and
Animal Nutrition. The sections vary in the number of chapters which was largely due
to the number of authors who contributed chapters to publish the book. The chapters
in each section and in the book in general vary in scope and the way they attempt to
X
Preface
manipulate resources and variables to improve on productivity and also to link
agricultural landscape to recreation and therefore human health, albeit remotely.
I acknowledge the authors for willingly contributing their chapters without which we
could not have published this book. I am equally grateful to Ms Jana Sertic, the
Publishing Process Manager for the able assistance she provided and to the
Information Technology Department for providing the requisite framework that
greatly enhanced the work of putting together the chapters in the book. The Technical
Editors deserve commendation for preparing the online publication and print versions
of the book.
Finally, I owe a debt of gratitude to the Scientific Board of the INTECH OPEN
ACCESS PUBLISHER for the trust reposed in me to edit this book. I am most grateful
to be of service to the scientific community.
Dr. Godwin Aflakpui
Rector, Wa Polytechnic, Wa, Upper West Region,
Ghana, West Africa
Section 1
Crop Improvement
1
Impact of Epistasis in Inheritance of
Quantitative Traits in Crops
Bnejdi Fethi and El Gazzeh Mohamed
Laboratoire de Génétique et Biométrie Faculté des Sciences de Tunis,
Université Tunis, El Manar,
Tunisia
1. Introduction
Epistasis is the interaction between alleles of different genes, i.e. non-allelic interaction, as opposed to dominance, which is interaction between allele of the same gene, called inter-allelic or intra-genic interaction (Kearsey and Pooni, 1996). Statistical epistasis describes the deviation that occurs when the combined additive effect of two or more genes does not
explain an observed phenotype (Falconer and Mackay, 1996).
The heritability of a trait, an essential concept in genetics quantitative, “certainly one of the central points in plant breeding research is the proportion of variation among individuals in a population that” is due to variation in the additive genetic (i.e., breeding) values of individuals:
h2 = VA/VP = Variance of breeding values/ phenotypic variance (Lynch and Walsh, 1998).
This definition is now termed “heritability in the narrow-sense” (Nyquist, 1991). Estimation of this parameter was prerequisite for the amelioration of quantitative traits. As well as choosing the selective procedure, that will maximize genetic gain with one or more selection cycles.
Various methods were developed in the past, Warner (1952), Sib-Analysis, Parent-offspring regressions etc. Theses methods considered that additive-dominant model is fitted, assuming epistasis to be negligible or non existent. Because of the complexity of theoretical genetics studies on epistasis, there is a lack of information about the contribution of the epistatic components of genotypic variance when predicting gains from selection. The estimation of
epistatic components of genotypic variance is unusual in genetic studies because the limitation of the methodology, as in the case of the triple test cross, the high number of generations to be produced and assessed (Viana, 2000), and mainly because only one type of progeny, Half-Sib, Full-Sib or inbred families, is commonly included in the experiments (Viana, 2005). If there is no epistasis, generally it is satisfactory to assess the selection efficiency and to predict gain based on the broad-sense heritability. Therefore, the bias in the estimate of the additive variance when assuming the additive-dominant model is considerable. The preponderance of
epistasis effect in the inheritance of quantitative trait in crops was recently reported by many geneticists (Pensuk et al., 2004; Bnejdi and El Gazzah, 2008; Bnejdi et al. 2009; Bnejdi and El-Gazzah, 2010a; Shashikumar et al. 2010). Epistasis can have an important influence on a
number of evolutionary phenomena, including the genetic divergence between species.
4
Agricultural Science
The aims of our study were to determine the importance of epistasis effects in heredity of quantitative traits and their consequences in the bias of four methods of estimation of
narrow-sense heritability.
2. Origin of data and genetic model
Nine quantitative traits with 88 cases of combination cross-site, cross-isolate or cross-
treatment of six generations (P1, P2, F1, F2, BC1 and BC2) for three crops ( Triticum Durum, Capsicum annum and Avena sp) were collected from different works realised in our laboratory. Crops, traits and origin of data are reported in Table 1. For each trait parents of crosses were extreme. Transformations (such as Kleckowski transforms (Lynch and Walsh,
1998)) were applied to normalize the distribution of data or to make means independent of variances for several traits.
Durum Wheat
( Triticum durum)
Two crosses/two sites
Number of head per plant , Spiklets per spike and Number of grains per spike (Bnejdi and
El Gazzeh 2010b)
Four crosses/ one site
Resistance to yellowberry (Bnejdi and El Gazzah, 2008)
Four crosses/ one site
Resistance to yellowberry (Bnejdi et al., 2010a)
Four crosses/ Two sites
Grain protein content (Bnejdi and El Gazzeh, 2010a)
Two crosses/ Five salt treatments
Resistance to salt at germination stage (Bnejdi et al., 2011a)
Two crosses/ fifteen isolates
Resistance to Septoria tritici (Bnejdi et al., 2011b)
Pepper
( Capsicum annuum L.)
Two crosses/ Two isolates
Resistance to Phytophthora nicotianae (Bnejdi et al., 2009)
Two crosses/ Six isolates
Resistance to Phytophthora nicotianae (Bnejdi et al., 2010b)
Oates
(Avena sp.)
Two crosses/ Two isolates
Resistance to P. coronate Cda. f. sp. avenae Eriks (Bnejdi et al., 2010c)
Table 1. Traits assessed in each crop and date of publication
Impact of Epistasis in Inheritance of Quantitative Traits in Crops
5
2.1 Best genetic model
Weighted least squares regression analyses were used to solve for mid-parent [M] pooled additive [A], pooled dominance [D] and pooled digenic epistatic ([AA], [DD] and [AD])
genetic effects, following the models and assumptions described in Mather and Jinks (1982).
A simple additive-dominance genetic model containing only M, A and D effects was first tested using the joint scaling test described in Rowe and Alexander (1980). Adequacy of the genetic model was assessed using a chi-square goodness-of-fit statistic derived from
deviations from this model. If statistically significant at P < 0.05, genetic models containing digenic epistatic effects were then tested until the chi-square statistic was non-significant.
3. Phenotypic resemblance between relatives
We now will use the covariance (and the related measures of correlations and regression
slopes) to quantify the phenotypic resemblance between relatives. Quantitative genetics as a field traces back to Fisher’s 1918 paper showing how to use the phenotypic covariance to
estimate genetic variances, whereby the phenotypic covariance between relatives is
expressed in terms of genetic variances, as we detail below.
3.1 Parent-offspring regressions
There are three types of parent-offspring regressions: two single parent - offspring
regressions (plotting offspring mean versus either the trait value in their male parent Pf or their female parent Pm), and the mid-parent-offspring regression (the offspring mean regressed on the mean of their parents, the mid-parent MP = ( Pf + Pm) / 2).
The slope of the (single) parent-offspring regression is estimated by
Co (
v O, P)
1
n
b
, where
(
Cov O, P)
( Oi i
P n .
O P)
o/ p
Var( P)
n1 i1
Where Oi is the mean trait value in the offspring of parent i (Pi) and we examine n pairs of parent-offspring. One could compute separate regressions using males ( Pm) and females ( Pf), although the later potentially includes maternal effect contributions and hence single-parent regressions usually restricted to fathers.
Co (
v O, P)
o
b / p
Var( P)
2
2
2
2
A
AA AAA AAAA
Co (
v O, P)
(
.........)
2
4
8
16
2
2
2
2
*
Co (
v O, P) A
1 AA AAA AAAA
o
b /
(
.........)
p
2
2
Var( P)
2
4
8
16
P
P
2
2
2
2
*
Co (
v O, P) h
1 AA AAA AAAA
o
b /
(
.........)
p
2
Var( P)
2
4
8
16
P
Assuming an absence of epistasis we have
6
Agricultural Science
2
1
2
2
A h
o
b / p
2
1
(
Cov O, P)
2
2
2
A , giving
P
2
h 2 o
b / p
3.2 Full-sib analysis
The covariance full-sib analysis is equal to:
1 2
1 2
1 2
1 2
1 2
1 2
(
Cov FS)
......)
2 A 4 D 4 AA 8 AD 16 DD 8 AAA
2
(
Cov FS) h
1 1
2
1 2
1 2
1 2
1 2
(
.....)
2
2
2
4 D 4 AA 8 AD 16 DD 8 AAA
P
P
So, when epistasis was considered negligible
1 2
Co (
v FS)
2 A
2
2 Co (
v FS)
h
2
P
3.3 Half-sib analysis
Based on half-sib analysis, narrow-sense heritability was calculated as:
1 2
1 2
1 2
(
Cov HS)
......
4 A 16 AA 64 AAA
2
(
Cov HS) h
1 1
2
1 2
(
......)
2
2
4
16 AA 64