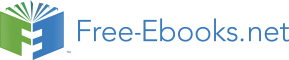

fluctuation
2
f
f
,
(5.6)
© K. Likharev
SM: Statistical Mechanics
is called the root-mean-square ( r.m.s. ) fluctuation. An advantage of this measure is that it has the same dimensionality as the variable itself, so that the ratio f/ f is dimensionless, and may be used to characterize the relative intensity of fluctuations.
As has been mentioned in Chapter 1, all results of thermodynamics are valid only if the fluctuations of thermodynamic variables (internal energy E, entropy S, etc.) are relatively small.1 Let us make a simple estimate of the relative intensity of fluctuations for an example of a system of N
independent, similar particles, and an extensive variable
N
F f .
(5.7)
k
k 1
where all single-particle functions fk are similar, besides that each of them depends on the state of only
“its own” ( k th) particle. The statistical average of such F is evidently N
F f N f ,
(5.8)
k 1
while its fluctuation variance is
N
N
N
N
~
~ ~
~
~
~ ~
~ ~
2
F
F F f
f
f f
f f
.
(5.9)
k
k '
k
k '
k k'
k 1
k' 1
k , k '1
k , k '1
Now we may use the fact that for two independent variables
~ ~
f f
,
0
k'
for k ;
(5.10)
k
k '
indeed, this relation may be considered as the mathematical definition of their independence. Hence, only the terms with k’ = k make substantial contributions to the sum (9):
~
N
2
~2
~
2
F
f N f .
(5.11)
k
k , k '
k , k ' 1
Comparing Eqs. (8) and (11), we see that the relative intensity of fluctuations of the variable F,
F
1
f
Relative
,
(5.12) fluctuation
1/ 2
F
N
f
estimate
tends to zero as the system size grows ( N ). It is this fact that justifies the thermodynamic approach to typical physical systems, with the number N of particles of the order of the Avogadro number N A ~
1024. Nevertheless, in many situations even small fluctuations of variables are important, and in this chapter we will calculate their basic properties, starting with the variance.
It should be comforting for the reader to notice that for some simple (but very important) cases, such calculation has already been done in our course. In particular, for any generalized coordinate q and generalized momentum p that give quadratic contributions of the type (2.46) to the system’s 1 Let me remind the reader that up to this point, the averaging signs … were dropped in most formulas, for the sake of notation simplicity. In this chapter, I have to restore these signs to avoid confusion. The only exception will be temperature – whose average, following (probably, bad :-) tradition, will be still called just T everywhere, besides the last part of Sec. 3, where temperature fluctuations are discussed explicitly.
Chapter 5
Page 2 of 44
SM: Statistical Mechanics
Hamiltonian (as in a harmonic oscillator), we have derived the equipartition theorem (2.48), valid in the classical limit. Since the average values of these variables, in the thermodynamic equilibrium, equal zero, Eq. (6) immediately yields their r.m.s. fluctuations:
1/ 2
1/ 2
1/ 2
T
T
1/ 2
p
( mT) ,
q
,
where .
(5.13)
2
m
m
The generalization of these classical relations to the quantum-mechanical case ( T ~ j) is provided by Eqs. (2.78) and (2.81):
1/ 2
1/ 2,
m
p
coth
,
q
coth
.
(5.14)
2
2
T
2 m
2
T
However, the intensity of fluctuations in other systems requires special calculations. Moreover, only a few cases allow for general, model-independent results. Let us review some of them.
5.2. Energy and the number of particles
First of all, note that fluctuations of macroscopic variables depend on particular conditions.2 For example, in a mechanically- and thermally-insulated system with a fixed number of particles, i.e. a member of a microcanonical ensemble, the internal energy does not fluctuate: E = 0. However, if such a system is in thermal contact with the environment, i.e. is a member of a canonical ensemble (Fig. 2.6), the situation is different. Indeed, for such a system we may apply the general Eq. (2.7), with Wm given by the Gibbs distribution (2.58)-(2.59), not only to E but also to E 2. As we already know from Sec. 2.4, the first average,
1
E
E
m
m
E W E ,
W
exp
,
Z
exp
,
(5.15)
m
m
m
m
Z
T
m
T
yields Eq. (2.61b), which may be rewritten in the form
1
Z
1
E
,
where β
,
(5.16)
Z ( )
T
more convenient for our current purposes. Let us carry out a similar calculation for E 2: 2
2
1
E
W E
E 2 exp E .
(5.17)
m
m
m m
m
Z m
It is straightforward to verify, by double differentiation, that the last expression may be rewritten in a form similar to Eq. (16):
2
2
1
1 Z
2
E
exp E
.
(5.18)
m
2
2
Z ( ) m
Z ( )
Now it is easy to use Eqs. (4) to calculate the variance of energy fluctuations:
2
~
Z
Z
Z
E
2
1
2
2
2
1
1
E
E E
.
(5.19)
Z ( )2 Z ( )
( ) Z () ()
2 Unfortunately, even in some popular textbooks, certain formulas pertaining to fluctuations are either incorrect or given without specifying the conditions of their applicability, so that the reader’s caution is advised.
Chapter 5
Page 3 of 44
SM: Statistical Mechanics
Since Eqs. (15)-(19) are valid only if the system’s volume V is fixed (because its change may affect the energy spectrum Em), it is customary to rewrite this important result as follows:
~
E
E
2
2
2
E
T
C T .
(5.20) Fluctuations
( 1
/ T )
T
V
of E
V
This is a remarkably simple, fundamental result. As a sanity check, for a system of N similar, independent particles, E and hence CV are proportional to N, so that E N 1/2 and E/ E N–1/2, in agreement with Eq. (12). Let me emphasize that the classically-looking Eq. (20) is based on the general Gibbs distribution, and hence is valid for any system (either classical or quantum) in thermal equilibrium.
Some corollaries of this result will be discussed in the next section, and now let us carry out a very similar calculation for a system whose number N of particles in a system is not fixed, because they may go to, and come from its environment at will. If the chemical potential of the environment and its temperature T are fixed, i.e. we are dealing with the grand canonical ensemble (Fig. 2.13), we may use the grand canonical distribution (2.106)-(2.107):
1
N
E
N
E
m, N
m, N
W
exp
,
Z
exp
.
(5.21)
m, N
G
Z
T
,
T
G
N m
Acting exactly as we did above for the internal energy, we get
1
N Em N
T Z
N
N exp
,
G ,
(5.22)
Z
T
Z
G m N
,
G
N Em N T Z
2
1
2
2
2
N
N exp
,
G ,
(5.23)
2
Z
T
Z
G m N
,
G
so that the particle number’s variance is
~ 2
T 2
2
2
Z
2
2
G
T Z
G
T Z
N
N
N N
T
G
T
,
(5.24) Fluctuations
Z
2
Z
of N
G
G
Z
G
in full analogy with Eq. (19).
In particular, for an ideal classical gas, we may combine the last result with Eq. (3.32b). (As was already emphasized in Sec. 3.2, though that result has been obtained for the canonical ensemble, in which the number of particles N is fixed, at N >> 1 the fluctuations of N in the grand canonical ensemble should be relatively small, so that the same relation should be valid for the average N in that ensemble.) Easily solving Eq. (3.32b) for N, we get
N const exp
,
(5.25)
T
where “const” means a factor constant at the partial differentiation of N over , required by Eq. (24).
Performing the differentiation and then using Eq. (25) again,
Chapter 5
Page 4 of 44
Essential Graduate Physics
SM: Statistical Mechanics
N
1
N
const exp
,
(5.26)
T
T
T
we get from Eq. (24) a very simple result:
Fluctuations
~
of N:
1/ 2
2
N
N ,
i.e. N
N
.
(5.27)
classical gas
This relation is so important that I will also show how it may be derived differently. As a byproduct of this new derivation, we will prove that this result is valid for systems with an arbitrary (say, small) N, and also get more detailed information about the statistics of fluctuations of that number. Let us consider an ideal classical gas of N 0 particles in a volume V 0, and calculate the probability WN to have exactly N N 0 of these particles in its part of volume V V 0 – see Fig. 1.
V, N
V
0 , N 0
Fig. 5.1. Deriving the binomial
and Poisson distributions.
For one particle such probability is W = V/ V 0 = N/ N 0 1, while the probability to have that particle in the remaining part of the volume is W’ = 1 – W = 1 – N/ N 0. If all particles were distinguishable, the probability of having N N 0 specific particles in volume V and ( N – N 0) specific particles in volume ( V – V
- N)
0), would be WNW’( N 0
. However, if we do not want to distinguish the
particles, we should multiply this probability by the number of possible particle combinations keeping the numbers N and N 0 constant, i.e. by the binomial coefficient N 0!/ N!( N 0 – N)!.3 As the result, the required probability is
N
N
0
N
Binomial
N
N N
N
N
N
N
(
)
!
!
0
0
W W W'
1
0
.
(5.28)
distribution
N
N!( N N )! N
N
N!( N N )!
0
0
0
0
This is the so-called binomial probability distribution, valid for any N and N 0.4
Still keeping N arbitrary, we can simplify the binomial distribution by assuming that the whole volume V 0, and hence N 0, are very large:
N N ,
(5.29)
0
where N means all values of interest, including N . Indeed, in this limit we can neglect N in comparison with N 0 in the second exponent of Eq. (28), and also approximate the fraction N 0!/( N 0 – N)!, i.e. the product of N terms, ( N
N
0 – N + 1) ( N 0 – N + 2)…( N 0 – 1) N 0, by just N 0 . As a result, we get N
N 0
N
N 0
N
N
N
N
N
N
N
N
N
0
1
W
1
1
1 W
,
(5.30)
N
W
N
N
N!
N
!
N
N
!
0
0
0
3 See, e.g., MA Eq. (2.2).
4 It was derived by Jacob Bernoulli (1655-1705).
Chapter 5
Page 5 of 44
SM: Statistical Mechanics
where, as before, W = N / N 0. In the limit (29), W 0, so that the factor inside the square brackets tends to 1/ e, the reciprocal of the natural logarithm base.5 Thus, we get an expression independent of N 0: N N N
W
e
.
(5.31) Poisson
N
N!
distribution
This is the much-celebrated Poisson distribution 6 which describes a very broad family of random phenomena. Figure 2 shows this distribution for several values of N – which, in contrast to N, are not necessarily integer.
1
N
0.8
.
0 1
0.6
WN 0.4
1
2
Fig. 5.2. The Poisson distribution for
4
0.2
several values of N . In contrast to
8
that average, the argument N may take
0
only integer values, so that the lines in
0
2
4
6
8
10
12
14
these plots are only guides for the eye.
N
In the limit of very small N, the function WN( N) is close to an exponent, WN ≈ WN N N, while in the opposite limit, N >> 1, it rapidly approaches the Gaussian (or “normal”) distribution 7
1
( N N )2
W
(5.32) Gaussian
N
distribution
2
exp
.
1/ 2 N
(
2 N
)2
(Note that the Gaussian distribution is also valid if both N and N 0 are large, regardless of the relation between them – see Fig. 3.)
Binomial distribution
N N 0
Poisson distribution
Eq. (28)
Eq. (31)
Gaussian distribution
Eq. (32)
Fig. 5.3. The hierarchy of three
1 N , N
1 N
0
major probability distributions.
5 Indeed, this is just the most popular definition of that major mathematical constant – see, e.g., MA Eq. (1.2a) with n = –1/ W.
6 Named after the same Siméon Denis Poisson (1781-1840) who is also responsible for other mathematical tools and results used in this series, including the Poisson equation – see Sec. 6.4 below.
7 Named after Carl Friedrich Gauss (1777-1855), though Pierre-Simone Laplace (1749-1827) is credited for substantial contributions to its development.
Chapter 5
Page 6 of 44
SM: Statistical Mechanics
A major property of the Poisson (and hence of the Gaussian) distribution is that it has the same variance as given by Eq. (27):
~
N
N N 2
2
N .
(5.33)
(This is not true for the general binomial distribution.) For our current purposes, this means that for the ideal classical gas, Eq. (27) is valid for any number of particles.
5.3. Volume and temperature
What are the r.m.s. fluctuations of other thermodynamic variables – like V, T, etc.? Again, the answer depends on specific conditions. For example, if the volume V occupied by a gas is externally fixed (say, by rigid walls), it evidently does not fluctuate at all: V = 0. On the other hand, the volume may fluctuate in the situation when the average pressure is fixed – see, e.g., Fig. 1.5. A formal calculation of these fluctuations, using the approach applied in the last section, is complicated by the fact that it is physically impracticable to fix its conjugate variable, P, i.e. suppress its fluctuations. For example, the force F( t) exerted by an ideal classical gas on a container’s wall (whose measure the pressure is) is the result of individual, independent hits of the wall by particles (Fig. 4), with the time scale c ~ r B/ v 21/2 ~ r B/( T/ m)1/2 ~ 10-16 s, so that its frequency spectrum extends to very high frequencies, virtually impossible to control.
F ( t)
Fig. 5.4. The force exerted by gas
particles on a container’s wall, as a
F
function of time (schematically).
0
t
However, we can use the following trick, very typical for the theory of fluctuations. It is almost evident that the r.m.s. fluctuations of the gas volume are independent of the shape of the container. Let us consider a particular situation similar to that shown in Fig. 1.5, with the container of a cylindrical shape, with the base area A.8 Then the coordinate of the piston is just q = V/ A, while the average force exerted by the gas on the cylinder is F = PA – see Fig. 5. Now if the piston is sufficiently massive, its free oscillation frequency near the equilibrium position is small enough to satisfy the following three conditions.
First, besides balancing the average force F and thus sustaining the average pressure P =
F / A of the gas, the interaction between the heavy piston and the relatively light particles of the gas is weak, because of a relatively short duration of the particle hits (Fig. 4). As a result, the full energy of the system may be represented as a sum of those of the particles and the piston, with a quadratic contribution to the piston’s potential energy by small deviations from the equilibrium:
8 As a math reminder, the term “cylinder” does not necessarily mean the “circular cylinder”; the shape of its base may be arbitrary; it just should not change with height.
Chapter 5
Page 7 of 44
Essential Graduate Physics
SM: Statistical Mechanics
~
2
V
U
q~ ,
q~
where
q q
,
(5.34)
p
2
A
and is the effective spring constant arising from the finite compressibility of the gas.
F P A
A, M
V
~
q
V V V ( t)
A
Fig. 5.5. Deriving Eq. (37).
Second, at 0, this spring constant may be calculated just as for constant variations of the volume, with the gas remaining in quasi-equilibrium at all times:
F
P
A 2
.
(5.35)
q
V
This partial derivative9 should be calculated at whatever the given thermal conditions are, e.g., with S =
const for adiabatic conditions (i.e., a thermally insulated gas), or with T = const for isothermal conditions (including a good thermal contact between the gas and a heat bath), etc. With that constant denoted as X, Eqs. (34)-(35) give
2
~
1
P V
1 P ~
2
2
U A
V .
(5.36)
p
2
V A
2 V
X
X
Finally, assuming that = (/ M)1/2 is sufficiently small (namely, << T) because of a sufficiently large piston mass M, we may apply, to the piston’s fluctuations, the classical equipartition theorem: U p = T/2, giving10
~
V
V 2
T
.
(5.37a) Fluctuations
of V
X
P
X
Since this result is valid for any A and , it should not depend on the system’s geometry and piston’s mass, provided that it is large in comparison with the effective mass of a single system component (say, a gas molecule) – the condition that is naturally fulfilled in most experiments. For the 9 As already was discussed in Sec. 4.1 in the context of the van der Waals equation, for the mechanical stability of a gas (or liquid), the derivative P/ V has to be negative, so that is positive.
10 One may meet statements that a similar formula,
~
P
P 2
T
,
(WRONG!)
X
V
X
is valid for pressure fluctuations. However, a such statement does not take into account a different physical nature of pressure (Fig. 4), with its very broad frequency spectrum. This issue will be discussed later in this chapter.
Chapter 5
Page 8 of 44
SM: Statistical Mechanics
particular case of fluctuations at constant temperature ( X = T),11 we may use the definition (3.58) of the isothermal bulk compressibility KT of the gas to rewrite Eq. (37a) as
~2
TV
V
.
(5.37b)
T
KT
For an ideal classical gas of N particles, with the equation of state V = NT/ P, it is easier to use directly Eq. (37a), again with X = T, to get
2
~
NT V
V
1
2
V
T
,
i.e.
T
,
(5.38)
2
1/ 2
T
P
N
V
N
in full agreement with the general trend given by Eq. (12).
Now let us proceed to fluctuations of temperature, for simplicity focusing on the case V = const.
Let us again assume that the system we are considering is weakly coupled to a heat bath of temperature T 0, in the sense that the time of temperature equilibration between the two is much larger than the time of internal equilibration, called thermalization. Then we may assume that, on the former time scale, T
changes virtually simultaneously in the whole system, and consider it a function of time alone:
~
T T T ( t) .
(5.39)
Moreover, due to the (relatively) large , we may use the stationary relation between small fluctuations of temperature and the internal energy of the system:
~
~
E t
( )
E
T t
( )
,
δ
that
so
T
.
(5.40)
C
C
V
V
With those assumptions, Eq. (20) immediately yields the famous expression for the so-called thermodynamic fluctuations of temperature:
Fluctuations
E
T
T
.
(5.41)
of T
1/ 2
C
C
V
V
The most straightforward application of this result is to analyses of so-called bolometers –
broadband detectors of electromagnetic radiation in microwave and infrared frequency bands. (In particular, they are used for measurements of the CMB radiation, which was discussed in Sec. 2.6). In such a detector (Fig. 6), the incoming radiation is focused on a small sensor (e.g., either a small piece of a germanium crystal or a superconductor thin film at temperature T T c, etc.), which is well isolated thermally from the environment. As a result, the absorption of an even small radiation power P leads to a noticeable change T of the sensor’s average temperature T and hence of its electric resistance R, which is probed up by low-noise external electronics.12 If the power does not change in time too fast, T
is a certain function of P, turning to 0 at P = 0. Hence, if T is much lower than the environment temperature T 0, we may keep only the main, linear term in its Taylor expansion in P: 11 In this case, we may also use the second of Eqs. (1.39) to rewrite Eq. (37) via the second derivative (2 G/ P 2) T.
12 Besides low internal electric noise, a good sensor should have a sufficiently large temperature responsivity dR/ dT, making the noise contribution by the readout electronics insignificant – see below.
Chapter 5
Page 9 of 44
Essential Graduate Physics
SM: Statistical Mechanics
P
T T T
,
(5.42)
0
G
where the coefficient G P/ T is called the thermal conductance of the (perhaps small but unavoidable) thermal coupling between the sensor and the heat bath – see Fig. 6.
~
T T T ( t)
G
T T T
0
T 0
R( T )
P
Fig. 5.6. The conceptual scheme of a bolometer.
to readout electronics
The power may be detected if the electric signal from the sensor, which results from the change
T, is not drowned in spontaneous fluctuations. In practical systems, these fluctuations are contributed by several sources including electronic amplifiers. However, in modern systems, these “technical”
contributions to noise are successfully suppressed,13 and the dominating noise source is the fundamental sensor temperature fluctuations, described by Eq. (41). In this case, the so-called noise-equivalent power (“NEP”), defined as the level of P that produces the signal equal to the r.m.s. value of noise, may be calculated by equating the expressions (41) (with T = T 0) and (42):
T
0
NEP
G
P
T
T
.
(5.43)
1/ 2
CV
This expression shows that to decrease the NEP, i.e. improve the detector’s sensitivity, both the environment temperature T 0 and the thermal conductance G should be reduced. In modern receivers of radiation, their typical values are of the order of 0.1 K and 10-10 W/K, respectively.
On the other hand, Eq. (43) implies that to increase the bolometer’s sensitivity, i.e. to reduce the
NEP, the CV of the sensor, and hence its mass, should be increased. This conclusion is valid only to a certain extent, because due to technical reasons (parameter drifts and the so-called 1/ f noise of the sensor and external electronics), the incoming power has to be modulated with as high frequency as technically possible (in practical receivers, the cyclic frequency = /2 of the modulation is between 10 and 1,000 Hz), so that the electrical signal might be picked up from the sensor at that frequency. As a result, the CV may be increased only until the thermal constant of the sensor,
C
V
,
(5.44)
G
becomes close to 1/, because at >> 1 the useful signal drops faster than noise. So, the lowest (i.e.
the best) values of the NEP,
13 An important modern trend in this progress [see, e.g., P. Day et al., Nature 425, 817 (2003)] is the replacement of the resistive temperature sensors R( T) with thin and narrow superconducting strips with temperature-sensitive kinetic inductance L k( T) – see the model solution of EM Problem 6.19. Such inductive sensors have zero dc resistance, and hence vanishing Johnson-Nyquist noise at typical signal pickup frequencies of a few kHz – see Eq.
(81) and its discussion below.
Chapter 5
Page 10 of 44
SM: Statistical Mechanics
(NEP)
1/ 2 1/ 2
T G ,
with ~ 1,
(5.45)
min
0
are reached at 1. (The exact values of the optimal product , and of the numerical constant ~ 1
in Eq. (45), depend on the exact law of the power modulation in time, and the readout signal processing procedure.) With the parameters cited above, this estimate yields (NEP)min/1/2 ~ 310-17 W/Hz1/2 – a very low power indeed.
However, perhaps counter-intuitively, the power modulation allows the bolometric (and other broadband) receivers to register radiation with power much lower than this NEP! Indeed, picking up the sensor signal at the modulation frequency , we can use the subsequent electronics stages to filter out all the noise besides its components within a very narrow band, of width << , around the modulation frequency (Fig. 7). This is the idea of a microwave radiometer,14 currently used in all sensitive broadband receivers of radiation.
input
power modulation
frequency
noise density
0
Fig. 5.7. The basic idea of the Dicke
frequency
pick-up
radiometer.
to output
In order to analyze this opportunity, we need to develop theoretical tools for a quantitative description of the spectral distribution of fluctuations. Another motivation for that description is a need for analysis of variables dominated by fast (high-frequency) components, such as pressure – please have one more look at Fig. 4. Finally, during such an analysis, we will run into the fundamental relation between fluctuations and dissipation, which is one of the main results of statistical physics as a whole.
5.4. Fluctuations as functions of time
In the previous sections, the averaging … of any function was assumed to be over an appropriate statistical ensemble of many similar systems. However, as was discussed in Sec. 2.1, most physical systems of interest are ergodic. If such a system is also stationary, i.e. the statistical averages of its variables do not change with time, the averaging may be also understood as that over a sufficiently long time interval. In this case, we may think about fluctuations of any variable f as of a random process
~
~
taking place in just one system, but developing in time: f f ( t) .
There are two mathematically equivalent approaches to the description of such random functions of time, called the time-domain picture and the frequency-domain picture, their relative convenience 14 It was pioneered in the 1950s by Robert Henry Dicke, so that the device is frequently called the Dicke radiometer. Note that the optimal strategy of using similar devices for time- and energy-resolved detection of single high-energy photons is different – though even it is essentially based on Eq. (41). For a recent brief review of such detectors see, e.g., K. Morgan, Phys. Today 71, 29 (Aug. 2018), and references therein.
Chapter 5
Page 11 of 44
SM: Statistical Mechanics
depending on the particular problem to be solved. In the time domain, we need to characterize a random
~
~
fluctuation f ( t) by some deterministic function of time. Evidently, the average f ( t) cannot be used for this purpose, because it equals zero – see Eq. (2). Of course, the variance (3) does not equal zero, but if the system is stationary, that average cannot depend on time either. Because of that, let us consider the following average:
~
~
f ( t) f ( t' ) .
(5.46)
Generally, this is a function of two arguments. However, in a stationary system, the average like (46) may depend only on the difference,
τ t' t ,
(5.47)
between the two observation times. In this case, the average (46) is called the correlation function of the variable f:
~
~
K ( ) f ( t) f ( t ) .
(5.48) Correlation
f
function
Again, here the averaging may be understood as that either over a statistical ensemble of macroscopically similar systems or over a sufficiently long interval of the time argument t, with the argument kept constant. The correlation function’s name15 catches the idea of this notion very well: Kf() characterizes the mutual relation between the fluctuations of the variable f at two times separated by the given interval . Let us list the basic properties of this function.16
First of all, Kf () has to be an even function of the time delay . Indeed, we may write
~
~
~
~
~
~
K (
) f ( t) f ( t ) f ( t ) f ( t) f ( t' ) f ( t' ) , (5.49)
f
with t’ t – . For stationary processes, this average cannot depend on the common shift of two observation times, so that the averages (48) and (49) have to be equal:
K (
) K ( ) .
(5.50)
f
f
Second, at 0 the correlation function tends to the variance:
~
~
~
K ( )
0 f ( t) f ( t)
2
f
0 .
(5.51)
f
In the opposite limit, when is much larger than certain characteristic correlation time c of the system,17 the correlation function has to tend to zero because the fluctuations separated by such time interval are virtually independent ( uncorrelated). As a result, the correlation function typically looks like one of the plots sketched in Fig. 8.
15 Another term, the autocorrelation function, is sometimes used for the average (48) to distinguish it from the mutual correlation function, f 1( t) f 2( t + ), of two different stationary processes.
16 Please notice that this correlation function is the direct temporal analog of the spatial correlation function briefly discussed in Sec. 4.2 – see Eq. (4.30).
17 Note that the correlation time c is the direct temporal analog of the correlation radius r c that was discussed in Sec. 4.2 – see the same Eq. (4.30).
Chapter 5
Page 12 of 44
SM: Statistical Mechanics
K ( )
f
2
ˆ f
Fig. 5.8. The correlation function of
0
fluctuations: two typical examples.
c
c
Note that on a time scale much longer than c , any physically-realistic correlation function may be well approximated with a delta function of . (For example, for a process which is a sum of independent very short pulses, e.g., the gas pressure force exerted on the container wall (Fig. 4), such approximation is legitimate on time scales much longer than the single pulse duration, e.g., the time of particle’s interaction with on the wall at the impact.)
~
In the reciprocal, frequency domain, the same process f ( t) is represented as a Fourier integral,18
~
f ( t)
i
f e
t ,
(5.52)
d
with the reciprocal transform being
1
~
f
f t() ei tdt .
(5.53)
2
~
If the function f ( t) is random (as it is in the case of fluctuations), with zero average, its Fourier transform f is also a random function (now of frequency), also with a vanishing statistical average.
Indeed, now thinking of the operation … as an ensemble averaging, we may write
1 ~
i t
1 ~
f
f ( t) e
dt
f ( t) ei t dt 0
.
(5.54)
2
2
The simplest non-zero average may be formed similarly to Eq. (46), but with due respect to the complex-variable character of the Fourier images:
*
1
~
~
i( ω't' ωt)
f f
ω'
dt' dt f t f t' e
.
(5.55)
2
( ) ( )
2
It turns out that for a stationary process, the averages (46) and (55) are directly related. Indeed, since the integration over t’ in Eq. (55) is in infinite limits, we may replace it with the integration over
t’ – t (at fixed t), also in infinite limits. Replacing t’ with t + in the expressions under the integral, we see that the average is just the correlation function Kf(), while the time exponent is equal to exp{ i( ’ – ) t}exp{ i ’}. As a result, changing the order of integration, we get
1
1
*
i( ' ) t i '
i '
i(
f f
'
) t
'
. (5.56)
2 2 dt d K ( ) e
e
K ( ) e
d
e
dt
f
2
f
2
But the last integral is just 2( – ’),19 so that we finally get
18 The argument of the function f is represented as its index with a purpose to emphasize that this function is
~
different from f ( t) , while (very conveniently) still using the same letter for the same variable.
Chapter 5
Page 13 of 44
SM: Statistical Mechanics
f f * S (
) ( '
),
ω'
(5.57)
f
where the real function of frequency,
1
i
1
Spectral
S ()
,
(5.58)
f
K () e d
f
K ()cos d
density of
2
f
0
fluctuations
is called the spectral density of fluctuations at frequency . According to Eq. (58), the spectral density is just the Fourier image of the correlation function, and hence the reciprocal Fourier transform is:20,21
Wiener-
K ( )
i
.
(5.59) Khinchin
f
S ()
e
d 2
f
S ()cos d
f
theorem
0
In particular, for the fluctuation variance, Eq. (59) yields
~
2
f
K (0)
.
(5.60)
f
S () d 2
f
S () d
f
0
The last relation shows that the term “spectral density” describes the physical sense of the function Sf() very well. Indeed, if a random signal f( t) had been passed through a frequency filter with a small bandwidth << of positive cyclic frequencies, the integral in the last form of Eq. (60) could be limited to the interval = 2, i.e. the variance of the filtered signal would become
~
2
f
2 S
( )
4 S
( )
.
(5.61)
f
f
(A popular alternative definition of the spectral density is S f() 4 Sf(), making the average (61) equal to just S f().)
To conclude this introductory (mostly mathematical) section, let me note an important particular case. If the spectral density of some process is nearly constant within all the frequency range of interest, Sf() = const = Sf(0),22 Eq. (59) shows that its correlation function may be well approximated with a delta function:
K ( ) S (0)
i
e
d 2 S (0) ( ) .
(5.62)
f
f
f
From this relation stems another popular name of the white noise, the delta-correlated process. We have already seen that this is a very reasonable approximation, for example, for the gas pressure force fluctuations (Fig. 4). Of course, for the spectral density of a realistic, limited physical variable the approximation of constant spectral density cannot be true for all frequencies (otherwise, for example, 19 See, e.g., MA Eq. (14.4).
20 The second form of Eq. (59) uses the fact that, according to Eq. (58), Sf() is an even function of frequency –
just as Kf() is an even function of time.
21 Although Eqs. (58) and (59) look not much more than straightforward corollaries of the Fourier transform, they bear a special name of the Wiener-Khinchin theorem – after the mathematicians N. Wiener and A. Khinchin who have proved that these relations are valid even for the functions f( t) that are not square-integrable, so that from the point of view of standard mathematics, their Fourier transforms are not well defined.
22 Such process is frequently called the white noise, because it consists of all frequency components with equal amplitudes, reminding the white light, which consists of many monochromatic components with close amplitudes.
Chapter 5
Page 14 of 44
SM: Statistical Mechanics
the integral (60) would diverge, giving an unphysical, infinite value of its variance), and may be valid only at frequencies much lower than 1/c.
5.5. Fluctuations and dissipation
Now we are equipped mathematically to address one of the most important issues of statistical physics, the relation between fluctuations and dissipation This relation is especially simple for the following hierarchical situation: a relatively “heavy”, slowly moving system, weakly interacting with an environment consisting of rapidly moving, “light” components. A popular theoretical term for such a system is the Brownian particle, named after botanist Robert Brown who was first to notice (in 1827) the random motion of small particles (in his case, pollen grains), caused by their random hits by fluid’s molecules, under a microscope. However, the family of such systems is much broader than that of small mechanical particles. Just for a few examples, such description is valid for an atom interacting with electromagnetic field modes of the surrounding space, a clock pendulum interacting with molecules of the air around it, current and voltage in electric circuits, etc.23
One more important assumption of this theory is that the system’s motion does not violate the thermal equilibrium of the environment – well fulfilled in many cases. (Think, for example, about a typical mechanical pendulum – its motion does not overheat the air around it to any noticeable extent.) In this case, the averaging over a statistical ensemble of similar environments, at a fixed, specific motion of the system of interest, may be performed assuming their thermal equilibrium.24 I will denote such a
“primary” averaging by the usual angle brackets …. At a later stage, we may carry out additional,
“secondary” averaging, over an ensemble of many similar systems of interest, coupled to similar environments. When we do, such double averaging will be denoted by double angle brackets ….
Let me start from a simple classical system, a 1D harmonic oscillator whose equation of evolution may be represented as
~
~
q
m q
F ( t) F ( t) F ( t) F F ( t),
with F ( t) 0 ,
(5.63)
det
env
det
where q is the (generalized) coordinate of the oscillator, Fdet( t) is the deterministic external force, while both components of the force Fenv( t) represent the impact of the environment on the oscillator’s motion.
Again, on the time scale of the fast-moving environmental components, the oscillator’s motion is slow.
The average component F of the force exerted by the environment on such a slowly moving object is frequently independent of its coordinate q but does depend on its velocity q . For most such systems, the Taylor expansion of the force in small velocity has a non-zero linear term:
F
q ,
(5.64)
where the constant is usually called the drag (or “kinematic friction”, or “damping”) coefficient, so that Eq. (63) may be rewritten as
23 To emphasize this generality, in the forthcoming discussion of the 1D case, I will use the letter q rather than x for the system’s displacement.
24 For a usual (ergodic) environment, the primary averaging may be interpreted as that over relatively short time intervals, c << t << , where c is the correlation time of the environment, while is the characteristic time scale of motion of our “heavy” system of interest.
Chapter 5
Page 15 of 44
SM: Statistical Mechanics
~
Langevin
q
m q q
F ( t) F ( t) .
(5.65) equation
det
for classical
oscillator
This method of describing the environmental effects on an otherwise Hamiltonian system is called the Langevin equation.25 Due to the linearity of the differential equation (65), its general solution may be represented as a sum of two independent parts: the deterministic motion of the damped linear
~
oscillator due to the external force Fdet( t), and its random fluctuations due to the random forceF ( t) exerted by the environment. The former effects are well known from classical dynamics,26 so let us focus on the latter part by taking Fdet( t) = 0. The remaining term on the right-hand side of Eq. (65) describes the fluctuating part of the environmental force; in contrast to the average component (64), its intensity (read: its spectral density at relevant frequencies ~ 0 (/ m)1/2) does not vanish at q( t) = 0, and hence may be evaluated ignoring the system’s motion.27
Plugging into Eq. (65) the representation of both variables in the Fourier form similar to Eq.
(52), and requiring the coefficients before the same exp{- i t} to be equal on both sides of the equation, for their Fourier images we get the following relation:
m 2 q i q q
F ,
(5.66)
which immediately gives us q, i.e. the (random) complex amplitude of the coordinate fluctuations:
F
q
F
.
(5.67)
(
m 2 ) i
m
( 2 2 ) i
0
Now multiplying Eq. (67) by its complex conjugate for another frequency (say, ’), averaging both parts of the resulting equation, and using the formulas similar to Eq. (57) for each of them,28 we get the following relation between spectral densities of the oscillations and the random force: 29
1
S ()
S () .
(5.68)
q
2
m ( 2
2
)2
2
2
F
0
In the so-called low-damping limit ( << m0), the fraction on the right-hand side of Eq. (68) has a sharp peak near the oscillator’s own frequency 0 (describing the well-known effect of high- Q
resonance), and may be well approximated in that vicinity as
25 Named after Paul Langevin, whose 1908 work was the first systematic development of A. Einstein’s ideas on Brownian motion (see below) using this formalism. A detailed discussion of this approach, with numerical examples of its application, may be found, e.g., in the monograph by W. Coffey, Yu. Kalmykov, and J. Waldron, The Langevin Equation, World Scientific, 1996.
26 See, e.g., CM Sec. 5.1. Here I assume that the variable f( t) is classical, with the discussion of the quantum case postponed until the end of the section.
27 Note that the direct secondary statistical averaging of Eq. (65) with Fdet = 0 yields q = 0! This, perhaps a bit counter-intuitive result becomes less puzzling if we recognize that this is the averaging over a large statistical ensemble of random sinusoidal oscillations with all values of their phase, and that the (equally probable) oscillations with opposite phases give mutually canceling contributions to the sum in Eq. (2.6).
28 At this stage, we restrict our analysis to random, stationary processes q( t), so that Eq. (57) is valid for this variable as well, if the averaging in it is understood in the … sense.
29 Regardless of the physical sense of such a function of , and of whether its maximum is situated at a finite frequency 0 as in Eq. (68) or at = 0, it is often referred to as the Lorentzian (or “Breit-Wigner”) line.
Chapter 5
Page 16 of 44
SM: Statistical Mechanics
1
1
2 m 0
,
with
.
(5.69)
2
m 2
(
2 2
)
1
0
2 220 2
In contrast, the spectral density S F () of fluctuations of a typical environment is changing relatively slowly, so that for the purpose of integration over frequencies near 0 we may replace S F () with S F
(0). As a result, the variance of the environment-imposed random oscillations may be calculated, using Eq. (60), as30
d
2
1
~
q
2 S () d 2
.
(5.70)
q
S () d 2 S ( )
q
0
F
2
2
2
2
m
0
1
0
0
This is a well-known table integral,31 equal to , so that, finally:
2
1
~
q
2 S ( )
S ( )
S ( ) .
(5.71)
0
F
2
2
2
2
0
0
m
m F
F
0
0
But on the other hand, the weak interaction with the environment should keep the oscillator in thermodynamic equilibrium at the same temperature T. Since our analysis has been based on the classical Langevin equation (65), we may only use it in the classical limit 0 << T, in which we may use the equipartition theorem (2.48). In our current notation, it yields
T
~2
q
.
(5.72)
2
2
Comparing Eqs. (71) and (72), we see that the spectral density of the random force exerted by the environment has to be fundamentally related to the damping it provides:
S
(
)
T .
(5.73a)
0
F
Now we may argue (rather convincingly :-) that since this relation does not depend on oscillator’s parameters m and , and hence its eigenfrequency 0 = (/ m)1/2, it should be valid at any relatively low frequency (c << 1). Using Eq. (58) with 0, it may be also rewritten as a formula for the effective low-frequency drag coefficient:
No dissipation
1
1
~
~
without
K d
F 0F d
fluctuations
.
(5.73b)
F
T
T
0
0
Formulas (73) reveal an intimate, fundamental relation between the fluctuations and the dissipation provided by a thermally-equilibrium environment. Parroting the famous political slogan, there is “no dissipation without fluctuation” – and vice versa. This means in particular that the phenomenological description of dissipation barely by the drag force in classical mechanics32 is 30 Since in this case the process in the oscillator is entirely due to its environment, its variance should be obtained by statistical averaging over an ensemble of many similar (oscillator + environment) systems, and hence, following our convention, it is denoted by double angular brackets.
31 See, e.g. MA Eq. (6.5a).
32 See, e.g., CM Sec. 5.1.
Chapter 5
Page 17 of 44
SM: Statistical Mechanics
(approximately) valid only when the energy scale of the process is much larger than T. To the best of my knowledge, this fact was first recognized in 1905 by A. Einstein,33 for the following particular case.
Let us apply our result (73) to a free 1D Brownian particle, by taking = 0 and Fdet( t) = 0. In this case, both relations (71) and (72) give infinities. To understand the reason for that divergence, let us go back to the Langevin equation (65) with not only = 0 and Fdet(t)= 0, but also m 0 – just for the sake of simplicity. (The latter approximation, frequently called the overdamping limit, is quite appropriate, for example, for the motion of small particles in viscous fluids – such as in R. Brown’s experiments.) In this approximation, Eq. (65) is reduced to a simple equation,
~
~
q F ( t), with F ( t) 0 ,
(5.74)
which may be readily integrated to give the particle’s displacement during a finite time interval t: 1 t ~
q( t) q( t) q(0)
F ( t' ) dt' .
(5.75)
0
Evidently, at the full statistical averaging of the displacement, the fluctuation effects vanish, but this does not mean that the particle does not move – just that it has equal probabilities to be shifted in either of two possible directions. To see that, let us calculate the variance of the displacement: t
t
t
t
1
~
1
q~
~
2 t
( )
F ( )F ( )
(
) .
(5.76)
2 dt' dt"
t'
t" 2 dt' dt"K t' t"
F
0
0
0
0
As we already know, at times >> c, the correlation function may be well approximated by the delta function – see Eq. (62). In this approximation, with S (0) expressed by Eq. (73a), we get F
t
t
t
2
2
2
2
q~
Δ
t
( )
S 0
T
T
dt' dt" t"
( t' )
dt'
t 2 Dt ,
(5.77)
F
2
2
0
0
0
with
T
D
.
(5.78) Einstein’s
relation
The final form of Eq. (77) describes the well-known law of diffusion (“random walk”) of a 1D
system, with the r.m.s. deviation from the point of origin growing as (2 Dt)1/2. The coefficient D is this relation is called the coefficient of diffusion, and Eq. (78) describes the extremely simple and important34
Einstein’s relation between that coefficient and the drag coefficient. Often this relation is rewritten, in the SI units of temperature, as D = k B T K, where 1/ is the mobility of the particle. The physical sense of becomes clear from the expression for the deterministic velocity (particle’s “drift”), which follows from the averaging of both sides of Eq. (74) after the restoration of the term Fdet( t) in it: 33 It was published in one of the three papers of Einstein’s celebrated 1905 “triad”. As a reminder, another paper started the (special) relativity theory, and one more was the quantum description of the photoelectric effect, essentially starting the quantum mechanics. Not too bad for one year, one young scientist!
34 In particular, in 1908, i.e. very soon after Einstein’s publication, it was used by J. Perrin for an accurate determination of the Avogadro number N A. (It was Perrin who graciously suggested naming this constant after A.
Avogadro, honoring his pioneering studies of gases in the 1810s.)
Chapter 5
Page 18 of 44
SM: Statistical Mechanics
1
v
q( t) F ( t) F
( t) ,
(5.79)
drift
det
det
so that the mobility is just the drift velocity given to the particle by a unit force.35
Another famous embodiment of the general Eq. (73) is the thermal (or “Johnson”, or “Johnson-Nyquist”, or just “Nyquist”) noise in resistive electron devices. Let us consider a two-terminal, dissipation-free “probe” circuit, playing the role of the harmonic oscillator in our analysis carried out above, connected to a resistive device (Fig. 9), playing the role of the probe circuit’s environment. (The noise is generated by the thermal motion of numerous electrons, randomly moving inside the resistive device.) For this system, one convenient choice of the conjugate variables (the generalized coordinate and generalized force) is, respectively, the electric charge Q I( t) dt that has passed through the “probe”
circuit by time t, and the voltage V across its terminals, with the polarity shown in Fig. 9. (Indeed, the product V dQ is the elementary work d W done by the environment on the probe circuit.)
probe
I
V
R, T
circuit
Fig. 5.9. A resistive device as a dissipative
environment of a two-terminal probe circuit.
Making the corresponding replacements, q Q and F V in Eq. (64), we see that it becomes
Q
V
I .
(5.80)
Comparing this relation with Ohm’s law, V = R(- I),36 we see that in this case, the coefficient has the physical sense of the usual Ohmic resistance R of our dissipative device,37 so that Eq. (73a) becomes R
S
( )
T .
(5.81a)
V
Using last equality in Eq. (61), and transferring to the SI units of temperature ( T = k B T K), we may bring this famous Nyquist formula 38 to its most popular form:
Nyquist
~
formula
2
V
4 k T R
.
(5.81b)
B K
35 Note that in solid-state physics and electronics, the charge carrier mobility is usually defined as vdrift/E =
evdrift/Fdet e (where E is the applied electric field), and is traditionally measured in cm2/Vs.
36 The minus sign is due to the fact that in our notation, the current flowing in the resistor, from the positive terminal to the negative one, is (- I) – see Fig. 9.
37 Due to this fact, Eq. (64) is often called the Ohmic model of the environment’s response, even if the physical nature of the variables q and F is completely different from the electric charge and voltage.
38 It is named after Harry Nyquist who derived this formula in 1928 (independently of the prior work by A.
Einstein, M. Smoluchowski, and P. Langevin) to describe the noise that had been just discovered experimentally by his Bell Labs’ colleague John Bertrand Johnson. The derivation of Eq. (73) and hence Eq. (81) in these notes is essentially a twist of the derivation used by H. Nyquist.
Chapter 5
Page 19 of 44
SM: Statistical Mechanics
Note that according to Eq. (65), this result is only valid at a negligible speed of change of the coordinate q (in our current case, negligible current I), i.e. Eq. (81) expresses the voltage fluctuations as would be measured by a virtually ideal voltmeter, with its input resistance much higher than R.
On the other hand, using a different choice of generalized coordinate and force, q , F I (where V( t) dt is the generalized magnetic flux, so that d W = I V( t) dt Id), we get 1/ R, and Eq. (73) yields the thermal fluctuations of the current through the resistive device, as measured by a virtually ideal ammeter, i.e. at V 0:
1
~
4
2
k T
S
( )
T ,
i.e. I
B K
.
(5.81c)
I
R
R
The nature of Eqs. (81) is so fundamental that they may be used, in particular, for the so-called Johnson noise thermometry.39 Note, however, that these relations are valid for noise in thermal equilibrium only. In electric circuits that may be readily driven out of equilibrium by an applied voltage V, other types of noise are frequently important, notably the shot noise, which arises in short conductors, e.g., tunnel junctions, at applied voltages with V >> T / q, due to the discreteness of charge carriers.40 A straightforward analysis (left for the reader’s exercise) shows that this noise may be characterized by current fluctuations with the following low-frequency spectral density: I
q
~
S
( )
,
i.e. I 2
2 I
q
,
(5.82) Schottky
I
2
formula
where q is the electric charge of a single current carrier. This is the Schottky formula,41 valid for any relation between the average I and V. The comparison of Eqs. (81c) and (82) for a device that obeys the Ohm law shows that the shot noise has the same intensity as the thermal noise with the effective temperature
q V
T
T .
(5.83)
ef
2
This relation may be interpreted as a result of charge carrier overheating by the applied electric field, and explains why the Schottky formula (82) is only valid in conductors much shorter than the energy relaxation length l e of the charge carriers.42 (Another mechanism of shot noise suppression, which may become noticeable in highly conductive nanoscale devices, is the Fermi-Dirac statistics of electrons.43) Now let us return for a minute to the bolometric Dicke radiometer (see Figs. 6-7 and their discussion in Sec. 4), and use the Langevin formalism to finalize its analysis. For this system, the Langevin equation is an extension of the usual equation of heat balance:
39 See, e.g., J. Crossno et al., Appl. Phys. Lett. 106, 023121 (2015), and references therein.
40 Another practically important type of fluctuations in electronic devices is the low-frequency 1/f noise that was already mentioned in Sec. 3 above. I will briefly discuss it in Sec. 8.
41 It was derived by Walter Hans Schottky as early as 1918, i.e. even before Nyquist’s work.
42 See, e.g., Y. Naveh et al., Phys. Rev. B 58, 15371 (1998). In practically used metals, l e is of the order of 30 nm even at liquid-helium temperatures (and much shorter at room temperatures), so that the usual “macroscopic”
resistors do not exhibit the shot noise.
43 For a review of this effect see, e.g., Ya. Blanter and M. Büttiker, Phys. Repts. 336, 1 (2000).
Chapter 5
Page 20 of 44
SM: Statistical Mechanics
dT
~
C
G( T T ) P ( t) P ( t) ,
(5.84)
V
0
det
dt
~
where Pdet P describes the (deterministic) power of the absorbed radiation and P represents the effective source of temperature fluctuations. Now we can use Eq. (84) to carry out a calculation of the spectral density ST() of temperature fluctuations absolutely similarly to how this was done with Eq.
(65), assuming that the frequency spectrum of the fluctuation source is much broader than the intrinsic bandwidth 1/ = G/ CV of the bolometer, so that its spectral density at frequencies ~ 1 may be well approximated by its low-frequency value S (0):
P
2
1
S
.
(5.85)
T
S 0
P
i C
V
G
Then, requiring the variance of temperature fluctuations, calculated from this formula and Eq. (60), 2
T
2
~2
T
2 S () d 2 S
d
T
1
0
P
i C
G
0
0
V
(5.86)
d
S
2 S 0 1
0
P
P
2
2
2
C
G C
G C
V 0
/ V
,
V
to coincide with our earlier “thermodynamic fluctuation” result (41), we get
G 2
S ( )
0
T .
(5.87)
P
0
The r.m.s. value of the “power noise” within a bandwidth << 1/ (see Fig. 7) becomes equal to the deterministic signal power Pdet (or more exactly, the main harmonic of its modulation law) at 1/ 2
~
P P
2
P
2 S ( )
0
2
.
(5.88)
P
G
T
min
1/2
1/2 0
This result shows that our earlier prediction (45) may be improved by a substantial factor of the order of (/)1/2, where the reduction of the output bandwidth is limited only by the signal accumulation time t ~ 1/, while the increase of is limited by the speed of (typically, mechanical) devices performing the power modulation. In practical systems this factor may improve the sensitivity by a couple of orders of magnitude, enabling observation of extremely weak radiation. Maybe the most spectacular example is the recent measurements of the CMB radiation, which corresponds to blackbody temperature T K 2.726 K, with accuracy T K ~ 10-6 K, using microwave receivers with the physical temperature of all their components much higher than T. The observed weak (~10-5 K) anisotropy of the CMB radiation is a major experimental basis of all modern cosmology.44
Returning to the discussion of our main result, Eq. (73), let me note that it may be readily generalized to the case when the environment’s response is different from the Ohmic form (64). This opportunity is virtually evident from Eq. (66): by its derivation, the second term on its left-hand side is just the Fourier component of the average response of the environment to the system’s displacement: 44 See, e.g., a concise book by A. Balbi, The Music of the Big Bang, Springer, 2008.
Chapter 5
Page 21 of 44
SM: Statistical Mechanics
F
i
q .