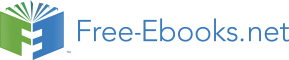

Very important
5
The scores for the four combinations are calculated as follows:
No. Of
Combi-
Score for
Total
Response
Res
nation
Response
score
pondents
Less important
1
2
1X2=2
I
Some what
3
6
3X6=18
important
5
4
5X4=20
12
40
Less important
1
5
1X5=5
II
Some what
3
3
3X3=9
important
5
4
5X4=20
12
34
Less important
1
3
1 X 3 = 3
III
Some what
3
3
3 X 3 = 9
important
5
6
5 X 6 = 30
12
42
199
Let us tabulate the scores earned by the four combinations as follows:
Combination
Total scores
I
40
II
34
III
42
IV
32
Inference:
It is concluded that the consumers consider combination III as the
most important and combination IV as the least important.
Note:
For illustrating the concepts involved, we have taken up 12
respondents in the above problem. In actual research work, we should take
a large number of respondents, say 200 or 100. In any case, the number of
respondents shall not be less than 30.
Illustrative Problem 2: Application Of Ranking Method
A marketing manager selects four combinations of features of a
product for study. The following are the ranks awarded by 10 respondents.
Rank one means the most important and rank 4 means the least important.
Res-
pondent
Rank Awarded
No.
Combination Combination Combination Combination
I
II
III
IV
1
2
1
3
4
2
1
4
2
3
3
1
2
3
4
4
3
2
4
1
200
5
4
1
2
3
6
1
2
3
4
7
4
3
2
1
8
3
1
2
4
9
3
1
4
2
10
4
1
2
3
Determine the most important and the least important combinations
of the features of the product.
Solution:
Let us assign scores to the ranks as follows:
Rank
Score
1
10
2
8
3
6
4
4
The scores for the 4 combinations are calculated as follows:
No.
Com-
Score
Rank
of
Total score
bination
for rank
respondents
1
10
3
10 X 3 = 30
2
8
1
8 X 1= 8
I
3
6
3
6 X 3 = 18
4
4
3
4 X 3 = 12
10
68
1
10
5
10 X 5 = 50
2
8
3
8 X 3 = 24
II
3
6
1
6 X 1 = 6
4
4
1
4 X 1 = 4
201
10
84
1
10
Nil
--
2
8
5
8 X 5 = 40
III
3
6
3
6 X 3 = 18
4
4
2
4 X 2 = 8
10
66
1
10
2
10 X 2 = 20
2
8
1
8 X 1 = 8
IV
3
6
3
6 X 3 = 18
4
4
4
4 X 4 = 16
10
62
The final scores for the 4 combinations are as follows:
Combination
Score
I
68
II
84
III
66
IV
62
Inference:
It is seen that combination II is the most preferred one by the
consumers and combination IV is the least preferred one.
Illustrative Problem 3:
Application Of Mini-Max Scaling Method
An insurance manager chooses 5 combinations of attributes of a
social security plan for analysis. He requests 10 respondents to indicate
their perceptions on the importance of the combinations by awarding
202
the minimum score and the maximum score for each combination in the
range of 0 to 100. The details of the responses are given below. Help
the manager in the identification of the most important and the least
important combinations of the attributes of the social security plan.
Com-
Com-
Com-
Com-
Com-
Res-
bination
bination
bination
bination bination
pondent
I
II
III
IV
V
No.
Min Max Min Max Min Max Min Max Min Max
30
60
45
85
50
70
40
75
50
80
1
35
65
50
80
50
80
35
75
40
75
2
40
70
35
80
60
80
40
70
50
80
3
40
80
40
80
60
85
50
75
60
80
4
30
75
50
80
60
75
60
75
60
85
5
35
70
35
85
50
80
40
80
40
80
6
40
80
40
75
45
75
50
70
40
80
7
30
80
40
75
50
80
50
70
60
80
8
45
75
45
75
50
80
50
80
50
80
9
55
75
40
85
35
75
45
80
40
80
10
Solution:
For each combination, consider the minimum score and the
maximum score separately and calculate the average in each case.
Com-
Com-
Com-
Com-
Com-
bination
bination
bination
bination bination
I
II
III
IV
V
Min Max Min Max Min Max Min Max Min Max
Total
380 730 420 800 510 780 460 750 490 800
Average 38 73
42
80 51 78 46 75 49 80
203
Consider the mean values obtained for the minimum and maximum
of each combination and calculate the range for each combination as
Range = Maximum Value – Minimum Value
The measure of importance for each combination is calculated as follows:
Measure of importance for a combination of attributes
Range for that combination
=
× 100
Sum of the ranges for al the combinations
Tabulate the results as follows:
Measure of
Combination Max. Value Min. Value
Range
Importance
I
73
38
35
21.875
II
80
42
38
23.750
III
78
51
27
16.875
IV
75
46
29
18.125
V
80
49
31
19.375
Sum of the ranges
160
100.000
Inference:
It is concluded that combination II is the most important one and
combination III is the least important one.
APPROACHES FOR CONJOINT ANALYSIS
The following two approaches are available for conjoint analysis:
1. Multi-factor evaluation approach
2. Two-factor evaluation approach
204
MULTI-FACTOR EVALUATION APPROACH IN CONJOINT
ANALYSIS
Suppose a researcher has to analyze n factors. It is possible that
each factor can assume a value in different levels.
Product Profile
A product profile is a description of all the factors under
consideration, with any one level for each factor.
Suppose, for example, there are 3 factors with the levels given below.
Factor
1 : 3
levels
Factor
2 : 2
levels
Factor
3 : 4
levels
Then we have 3× 2× 4 = 24 product profiles. For each respondent
in the research survey, we have to provide 24 data sheets such that each
data sheet contains a distinct profile. In each profile, the respondent is
requested to indicate his preference for that profile in a rating scale of 0
to 10. A rating of 10 indicates that the respondent’s preference for that
profile is the highest and a rating of 0 means that he is not all interested in
the product with that profile.
Example:
consider the product ‘Refrigerator’ with the following factors and
levels:
Factor 1
:
Capacity of 180 liters; 200 liters; 230 liters
Factor 2
:
Number of doors: either 1 or 2
Factor 3
:
Price : rs. 9000; rs. 10,000; rs. 12,000
Sample profile of the product
Profile Number :
Capacity
: 200 liters
Number of Doors : 1
205
Price
: Rs. 10,000
Rating of Respondent:
(in the scale of 0 to 10)
Steps In Multi-Factor Evaluation Approach:
1. Identify the factors or features of a product to be analyzed. If they are
too many, select the important ones by discussion with experts.
2. Find out the levels for each factor selected in step 1.
3. Design all possible product profiles. If there are n factors with levels
L , L ,…L respectively, then the total number of profiles = L L …L .
1
2
n
1 2
n
4. Select the scaling technique to be adopted for multi-factor evaluation
approach (rating scale or ranking method).
5. Select the list of respondents using the standard sampling technique.
6. Request each respondent to give his rating scale for all the profiles of
the product. Another way of collecting the responses is to request each
respondent to award ranks to all the profiles: i.e., rank 1 for the best
profile, rank 2 for the next best profile etc.
7. For each factor profile, collect all the responses from all the participating
respondents in the survey work.
8. With the rating scale awarded by the respondents, find out the score
secured by each profile.
9. Tabulate the results in step 8. Select the profile with the highest score.
This is the most preferred profile.
10. implement the most preferred profile in the design of a new product.
Two-Factor Evaluation Approach In Conjoint Analysis
When several factors with different levels for each factor have to be
analyzed, the respondents will have difficulty in evaluating all the profiles
in the multi-factor evaluation approach. Because of this reason, two-factor
evaluation approach is widely used in conjoint analysis.
206
Suppose there are several factors to be analyzed with different
levels of values for each factor, then we consider any two factors at a time
with their levels of values. For each such case, we have a data sheet called
a two-factor table. If there are n factors, then the number of such data
sheets is .
n
−
n( n 1)
=
2
2
Let us consider the example of ‘Refrigerator’ described in the multi-
factor approach. For the two factors (i) capacity and (ii) price, we have the
following data sheet.
Data Sheet (Two Factor Table) No:
Factor: price of refrigerator
Factor: Capacity
Price
of Refrigerator
Rs. 9,000
Rs. 10,000
Rs. 12,000
180 liters
200 liters
230 liters
In this case, the data sheet is a matrix of 3 rows and 3 columns.
Therefore, there are 3×3 = 9 places in the matrix. The respondent has
to award ranks from 1 to 9 in the cells of the matrix. A rank of 1 means
the respondent has the maximum preference for that entry and a rank of 9
means he has the least preference for that entry. Compared to multi-factor
evaluation approach, the respondents will find it easy to respond to two-
factor evaluation approach since only two factors are considered at a time.
Steps in two-factor evaluation approach:
Identify the factors or features of a product to be analyzed.
1.
Find out the levels for each factor selected in step 1.
2.
Consider all possible pairs of factors. If there are n factors, then the
207
n
−
number of pairs is
n( n 1)
=
. For each pair of factors, prepare
2
2
a two-factor table, indicating all the levels for the two factors. If L and L
1
2
are the respective levels for the two factors, then the number of cells in the
corresponding table is L L2.
1
3.
Select the list of respondents using the standard sampling technique.
4.
Request each respondent to award ranks for the cells in each two-
factor table. I.e., rank 1 for the best cell, rank 2 for the next best cell, etc.
5.
For each two-factor table, collect all the responses from all the
participating respondents in the survey work.
6.
With the ranks awarded by the respondents, find out the score
secured by each cell in each two-factor table.
7.
Tabulate the results in step 7. Select the cell with the highest score.
Identify the two factors and their corresponding levels.
8.
Implement the most preferred combination of the factors and their
levels in the design of a new product.
Application:
The two factor approach is useful when a manager goes for market
segmentation to promote his product. The approach will enable the top
level management to evolve a policy decision as to which segment of the
market has to be concentrated more in order to maximize the profit from
the product under consideration.
SELF ASSESSMENT QUESTIONS---END OF CHAPTER QUES-
TIONS[IMPORTANT FOR EXAMS]
1. Explain the purpose of ‘factor analysis’.
2. What is the objective of ‘conjoint analysis’? Explain.
3. State the steps in the development of conjoint analysis.
208
5. Enumerate the advantages and disadvantages of conjoint analysis.
6. What is a ‘product profile’? Explain.
7. What are the steps in multi-factor evaluation approach in conjoint
analysis?
8. What is a ‘two-factor table’? Explain.
9. Explain two-factor evaluation approach in conjoint analysis.
209
Statistical Table-1: F-values at 1% level of significance
df : degrees of freedom for greater variance
1
df : degrees of freedom for smaller variance
2
df2
1
2
3
4
5
6
7
8
9
10
/df1
1 4052.1 4999.5 5403.3 5624.5 5763.6 5858.9 5928.3 5981.0 6022.4 6055.8
2 98.5 99.0 99.1 99.2 99.2 99.3
99.3 99.3 99.3 99.3
3 34.1 30.8 29.4 28.7 28.2 27.9
27.6 27.4 27.3 27.2
4 21.1 18.0 16.6 15.9 15.5 15.2
14.9 14.7 14.6 14.5
5 16.2 13.2 12.0 11.3 10.9 10.6
10.4 10.2 10.1 10.0
6 13.7 10.9 9.7
9.1
8.7
8.4
8.2
8.1
7.9
7.8
7 12.2 9.5 8.4
7.8
7.4
7.1
6.9
6.8
6.7
6.6
8 11.2 8.6 7.5
7.0
6.6
6.3
6.1
6.0
5.9
5.8
9 10.5 8.0 6.9
6.4
6.0
5.8
5.6
5.4
5.3
5.2
10 10.0 7.5 6.5
5.9
5.6
5.3
5.2
5.0
4.9
4.8
11 9.6 7.2 6.2
5.6
5.3
5.0
4.8
4.7
4.6
4.5
12 9.3 6.9 5.9
5.4
5.0
4.8
4.6
4.4
4.3
4.2
13 9.0 6.7 5.7
5.2
4.8
4.6
4.4
4.3
4.1
4.1