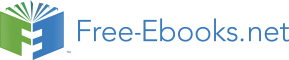

2
4
14
Sum of squares of deviations for B:
B
B − B = B − 7
( − )2
B B
7
0
0
6
-1
1
6
-1
1
9
2
4
6
Sum of squares of deviations for C:
C
C − C = C − 6
( − )2
C C
6
0
0
6
0
0
7
-1
1
5
-1
1
2
170
Sum of squares of deviations within
Varieties = ∑( − )2 + ∑( − )2
A A
B B + ∑( C − C)2
= 14 + 6 + 2
= 22
Sum of squares of deviations for total variance:
Sales -
Sales person
sales
X = sales
– 7
( Sales − )2
7
A
8
1
1
A
9
2
4
A
5
-2
4
A
10
3
9
B
7
0
0
B
6
-1
1
B
6
-1
1
B
9
2
4
C
6
-1
1
C
6
-1
1
C
7
0
0
C
5
2
4
30
171
ANOVA Table
Source of
Degrees of
Sum of squares
Variance
variation
freedom
of deviations
Between varieties
3 – 1 = 2
8
8 = 4
2
Within varieties
12 – 3 = 9
22
22 = 2.44
9
Total
12 – 1 = 11
30
Calculation of F value:
F
=
Greater Variance = 4.00 =1.6393
Smaller Variance
2.44
Degrees of freedom for greater variance ( df 1) = 2Degrees of freedom for
smaller variance ( df 1) = 9
Let us take the level of significance as 5%
The table value of F = 4.26
Inference:
The calculated value of F is less than the table value ofF. Therefore,
the null hypothesis is accepted. It is concluded that there is no significant
difference in the performance of the sales persons, at 5% level of
significance.
Method II (Short cut Method):
∑ A = 32, ∑ B = 28, ∑ C = 24.
T= Sum of all the sample items
172
= ∑ A+ ∑ B + ∑ C
= 32 + 28 + 24
= 84
N = Total number of items in all the samples = 4 + 4 + 4 =12
2
2
Correction Factor = T
84
=
= 588
N
12
Calculate the sum of squares of the observed values as follows:
Sales Person
X
X2
A
8
64
A
9
81
A
5
25
A
10
100
B
7
49
B
6
36
B
6
36
B
9
81
C
6
36
C
6
36
C
7
49
C
5
25
618
Sum of squares of deviations for total variance =
2
∑ X - correction
factor
= 618 – 588 = 30.
173
Sum of squares of deviations for variance between samples
(∑ A)2 (∑ B)2 (∑ C)2
=
+
+
− CF
N
N
N
1
2
3
2
2
2
32
28
24
=
+
+
− 588
4
4
4
1024 784 576
=
+
+
− 588
4
4
4
= 256 +196 +144 − 588
= 8
ANOVA
Table
Source of
Degrees of
Sum of squares
Variance
variation
freedom
of deviations
Bet
3-1 = 2
8
ween varieties
8 = 4
2
Within varieties
12 – 3 = 9
22
22 = 2.44
9
Total
12 – 1 = 11
30
It is to be noted that the ANOVA tables in the methods I and II
are one and the same. For the further steps of calculation of F value and
drawing inference, refer to method I.
174
Problem 2
The following are the details of plinth areas of ownership apartment
flats offered by 3 housing companies A,B,C. Use analysis of variance to
determine whether there is any significant difference in the plinth areas of
the apartment flats.
H o u s i n g
Plinth area of apartment flats
Company
A
1500
1430
1550
1450
B
1450
1550
1600
1480
C
1550
1420
1450
1430
Use analysis of variance to determine whether there is any
significant difference in the plinth areas of the apartment’s flats.
Note:
As the given figures are large, working with them will be difficult.
Therefore, we use the following facts:
i).
Variance ratio is independent of the change of origin.
ii.)
Variance ratio is independent of the change of scale.
In the problem under consideration, the numbers vary from 1420
to 1600. So we follow a method called the coding method. First, let us
subtract 1400 from each item. We get the following transformed data:
Company
Transformed measurement
A
100
30
150
50
B
50
150
100
80
C
150
20
50
30
Next, divide each entry by 10.
175
The transformed data are given below.
Company
Transformed measurement
A
10
3
15
5
B
5
15
10
8
C
15
2
5
3
We work with these transformed data. We have
∑ =
A 10+3+15+5=33
∑ B 5+
= 15+10+8=38
∑ C=15+2+5+3=25
∑ T = ∑ A+∑ B +∑ C
= 33+ 38 + 25
= 96
N = Total number of items in all the samples = 4 + 4 + 4 = 12
2
2
Correction factor = T
96
=
= 768
N
12
Calculate the sum of squares of the observed values as follows:
Company
X
X2
A
10
100
A
3
9
A
15
225
A
5
25
B
5
25
B
15
225
B
10
100
B
8
64
C
15
225
176
C
2
4
C
5
25
C
3
9
1036
Sum of squares of deviations for total variance =
2
∑ X - correction
factor
= 1036 – 768 = 268
Sum of squares of deviations for variance between samples
(∑ A)2 (∑ B)2 (∑ C)2
=
+
+
− CF
N
N
N
1
2
3
2
2
2
33
38
25
=
+
+
− 768
4
4
4
1089 1444 625
=
+
+
− 768
4
4
4
= 272.25 + 361+156.25 − 768
= 789.5 − 768
=
21.5
ANOVA Table
Source of
Degrees of
Sum of squares
Variance
variation
freedom
of deviation
Between varieties
3-1 = 2
21.5
21.5 =10.75
2
Within varieties
12 – 3 = 9
264.5
24.65 = 27.38
9
Total
12 – 1 = 11
268
177
Calculation of F value:
F = Greater Variance = 27.38 = 2.5470
Smaller Variance 10.75
Degrees of freedom for greater variance ( df 1) = 9
Degrees of freedom for smaller variance ( df 2 ) = 2
The table value of f at 5% level of significance = 19.38
Inference:
Since the calculated value of F is less than the table value of F, the
null hypothesis is accepted and it is concluded that there is no significant
difference in the plinth areas of ownership apartment flats offered by the
three companies, at 5% level of significance.
Problem 3
A finance manager has collected the following information on the
performance of three financial schemes.
Sum of squares of
Source of variation
Degrees of freedom
deviations
Treatments
5
15
Residual
2
25
Total (corrected)
7
40
Interpret the information obtained by him.
Note: ‘Treatments’ means ‘Between varieties’.
‘Residual’ means ‘Within varieties’ or ‘Error’.
178
Solution:
Number of schemes = 3 (since 3 – 1 = 2)
Total number of sample items = 8 (since 8 – 1 = 7)
Let us calculate the variance.
Variance between varieties =
15 = 7.5
2
Variance between varieties =
25 = 5
5
F
=
Greater Variance = 7.5 =1.5
Smaller Variance
5
Degrees of freedom for greater variance
( df 1) = 2
Degrees of freedom for smaller variance
( df 1) = 5
The total value of F at 5% level of significance
= 5.79
Inference:
Since the calculated value of F is less than the table value of F
we accept the null-hypothesis and conclude that there is no significant
difference in the performance of the three financial schemes.
179
SELF ASSESSMENT QUESTIONS---END OF CHAPTER
QUESTIONS.[IMPORTANT FOR EXAMS]
1. Define analysis of variance.
2. State the assumptions in analysis of variance.
3. Explain the classification of linear models for the sample
data.
4. Explain anova table.
5. Explain how inference is drawn from anova table.
6. Explain the managerial applications of analysis of variance.
180
CHAPTER IV
3. Partial And Multiple Correlation
The Concept Of Partial Correlation
The Concept Of Multiple Correlation
Determine Partial Correlation Coefficient
Determine Multiple Correlation Coefficient
I. PARTIAL CORRELATION
Simple correlation is a measure of the relationship between a
dependent variable and another independent variable. For example, if
the performance of a sales person depends only on the training that he
has received, then the relationship between the training and the sales
performance is measured by the simple correlation coefficient r. However,
a dependent variable may depend on several variables. For example, the
yarn produced in a factory may depend on the efficiency of the machine,
the quality of cotton, the efficiency of workers, etc. It becomes necessary
to have a measure of relationship in such complex situations. Partial
correlation is used for this purpose. The technique of partial correlation
proves useful when one has to develop a model with 3 to 5 variables.
Suppose Y is a dependent variable, depending on n other variables
X , X , …, X .. Partial correlation is a measure of the relationship between
1
2
n
Y and any one of the variables X , X ,…,X , as if the other variables have
1
2
n
been eliminated from the situation.
181
The partial correlation coefficient is defined in terms of simple correlation
coefficients as follows:
Let r denote the correlation of X and X by eliminating the
12. 3
1
2
effect of X .3
Let r be the simple correlation coefficient between X and X .
12
1
2
Let r be the simple correlation coefficient between X and X .
13
1
3
Let r be the simple correlation coefficient between X and X .
23
2
3
Then we have
−
r 12 r 13 r 23
r 12.3 =
2
2
(1− r 13) (1− r 23)
Similarly,
−
r 13 r 12 r 32
r 13.2 =
2
2
(1− r 12) (1− r 32)
−
and
r 23 r 21 r 13
r 32.1 =
2
2
(1− r 21) (1− r 13)
182
Problem 1
Given that r = 0.6, r = 0.58, r = 0.70 determine the partial
12
13
23
correlation coefficient r12.3
Solution:
We have
0.6−0.58 x 0.70
=
2
2
(1−(0.58) ) (1−(0.70) )
0.6−0.406
=
(1−0.3364) (1−0.49)
0.194
= 0.6636 x 0.51
0.194
=
0.8146 x 0.7141
0.194
= 0.5817
= 0.3335
183
Problem 2
If r = 0.75, r = 0.80, r = 0.70, find the partial correlation
12
13
23
coefficient r
13.2
Solution:
We have
r − r r
13
12
32
r 13.2 =
2
2
(1− r 12) (1− r 32)
−
0.8 0.75 X 0.70
=
2
2
(1− (0.75) ) (1− (0.70) )
0.8 − 0.525
= (1−0.5625) (1−0.49)
0.275
= (0.4375) (0.51)
0.275
=
0.6614 X 0.7141
0.275
=
0.4723
= 0.5823
II. MULTIPLE CORRELATION
When the value of a variable is influenced by another variable, the
relationship between them is a simple correlation. In a real life situation, a
variable may be influenced by many other variables. For example, the sales
achieved for a product may depend on the income of the consumers, the
price, the quality of the product, sales promotion techniques, the channels
of distribution, etc. In this case, we have to consider the joint influence
184
of several independent variables on the dependent variable. Multiple
correlations arise in this context.
Suppose Y is a dependent variable, which is influenced by n other
variables X , X , …,X . The multiple correlation is a measure of the
1
2
n