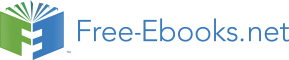

Time Period I Time Period II
Test area: Level of phenomenon Treatment Level of phenomenon
Before treatment (X) Introduced After treatment (Y)
Control area: Level of phenomenon Level of phenomenon
Without treatment Without treatment
(A) (Z)
Treatment Effect = (Y-X)-(Z-A)
This design is superior to the previous two designs because it
avoids extraneous variation resulting both from the passage of time and
from non-comparability of the rest and control areas. But at times, due to
lack of historical data time or a comparable control area, we should prefer
to select one of the first two informal designs stated above.
2. Formal Experimental Design
(i)
Completely randomized design:
This design involves only two principles i.e., the principle of
replication and the principle of randomization of experimental designs.
Among all other designs this is the simpler and easier because it’s procedure
and analysis are simple. The important characteristic of this design is
that the subjects are randomly assigned to experimental treatments. For
example, if the researcher has 20 subjects and if he wishes to test 10 under
treatment A and 10 under treatment B, the randomization process gives
every possible group of 10 subjects selected from a set of 20 an equal
opportunity of being assigned to treatment A and treatment B. One way
analysis of variance (one way ANOVA) is used to analyze such a design.
82
(ii)
Randomized block design:
R. B. Design is an improvement over the C.R. design. In the R .B.
Design, the principle of local control can be applied along with the other
two principles of experimental designs. In the R.B. design, subjects are
first divided into groups, known as blocks, such that within each group the
subjects are relatively homogenous in respect to some selected variable.
The number of subjects in a given block would be randomly assigned
to each treatment. Blocks are the levels at which we hold the extraneous
factor fixed, so that its contribution to the total variability of data can be
measured. The main feature of the R.B. design is that, in this, each treatment
appears the same number of times in each block. This design is analyzed
by the two-way analysis of variance (two-way ANOVA) technique.
(iii) Latin squares design:
The Latin squares design (L.S design) is an experimental design
which is very frequently used in agricultural research. Since agriculture
depends upon nature to a large extent, the condition of research and
investigation in agriculture is different than the other studies. For example,
an experiment has to be made through which the effects of fertilizers on
the yield of a certain crop, say wheat, are to be judged. In this situation, the
varying fertility of the soil in different blocks in which the experiment has
to be performed must be taken into consideration; otherwise the results
obtained may not be very dependable because the output happens to be
the effects of not only of fertilizers, but also of the effect of fertility of
soil. Similarly there may be the impact of varying seeds of the yield. In
order to overcome such difficulties, the L.S. design is used when there are
two major extraneous factors such as the varying soil fertility and varying
seeds. The Latin square design is such that each fertilizer will appear five
times but will be used only once in each row and in each column of the
design. In other words, in this design, the treatment is so allocated among
the plots that no treatment occurs more than once in any one row or any
one column. This experiment can be shown with the help of the following
diagram:
83
FERTILITY LEVEL
I II III IV V
X
A
B
C
D
E
1
X
B
C
D
E
A
2
X
C
D
E
A
B
3
X
D
E
A
B
C
4
X
E
A
B
C
D
5
From the above diagram, it is clear that in L.S. design the field
is divided into as many blocks as there are varieties of fertilizers. Then,
each block is again divided into as many parts as there are varieties of
fertilizers in such a way that each of the fertilizer variety is used in each of
the block only once. The analysis of L.S. design is very similar to the two-
way ANOVA technique.
(iv) Factorial design:
Factorial designs are used in experiments where the effects of
varying more than one factor are to be determined. These designs are
used more in economic and social matters where usually a large number
of factors affect a particular problem. Factorial designs are usually of two
types:
(i) Simple factorial designs and
(ii) Complex factorial designs.
(i)
Simple factorial design:
In simple factorial design, the effects of varying two factors on the
dependent variable are considered but when an experiment is done with
more than two factors, complex factorial designs are used. Simple factorial
design is also termed as a ‘two-factor-factorial design,’ whereas complex
factorial design is known as ‘multi-factor-factorial design.
84
(ii)
Complex factorial designs:
When the experiments with more than two factors at a time are
conducted, it involves the use of complex factorial designs. A design which
considers three or more independent variables simultaneously is called a
complex factorial design. In case of three factors with one experimental
variable, two treatments and two levels, complex factorial design will
contain a total of eight cells. This can be seen through the following
diagram:
2x2x2 COMPLEX FACTORIAL DESIGN
Experimental variable
Treatment A
Treatment B
Control
Control
Control
Control
variable 2
variable 2 variable 2
variable 2
Level I
Level II
Level III
Level II
Level I
Control
Cell 1
Cell 3
Cell 5
Cell 7
variable 2
Cell 2
Cell 4
Cell 6
Cell 8
Level II
A pictorial presentation is given of the design shown above in the following:
Experimental Variable
Treatment Treatment
Control Variable II A
B
Level II
Level I
Level I
Level
II
Control Variable I
85
The dotted line cell in this diagram corresponds to cell I of the above
stated 2x2x2 design and is for treatment A, level I of the control variable
1, and levelIi of the control variable 2. From this design, it is possible to
determine the main effects for three variables i.e., one experimental and
true control variables. The researcher can also determine the interaction
between each possible pair of variables (such interactions are called ‘first
order interactions’) and interaction between variable taken in triplets
(such interactions are called second order interactions). In case of a 2x2x2
design, the further given first order interactions are possible:
Experimental variable with control variable 1 (or EV x CV 1);
Experimental variable with control variable 2 (or EV x CV 2);
Control variable 1 with control variable 2 (or CV 1 x CV 2);
There will be one second order interaction as well in the given design (it is
between all the three variables i.e., EV x CV 1 x CV 2).
To determine the main effect for the experimental variable, the
researcher must necessarily compare the combined mean of data in cells 1,
2, 3 and 4 for Treatment A with the combined mean of data in cells 5,6,7
and 8 for Treatment B. In this way the main effect of experimental variable,
independent of control variable 1 and variable 2, is obtained. Similarly, the
main effect for control variable 1, independent experimental variable and
control variable 2, is obtained if we compare the combined mean of data in
cells 1, 3, 5 and 7 with the combined mean of data in cells 2, 4, 6 and 8 of
our 2x2x2 factorial design. On similar lines, one can determine the effect
of control variable 2 independent of experimental variable and control
variable 1, if the combined mean of data in cells 1,2,5 and 6 are compared
with the combined mean of data in cells 3,4,7 and 8.
To obtain the first order interaction, say, for EV x CV 1 in the above
stated design, the researcher must necessarily ignore control variable 2
for which purpose he may develop 2x2 design from the 2x2x2 design by
combining the data of the relevant cells of the latter design as has been
shown on next page:
86
Experimental Variable
Treatment A
Treatment B
Control
Level I
Cells 1,3
Cells 5,7
Variable 1
Level II
Cells 2,4
Cells 6,8
Similarly, the researcher can determine other first order interactions.
The analysis of the first order interaction in the manner described above is
essentially a simple factorial analysis as only two variables are considered
at a time and the remaining ones are ignored. But the analysis of the second
order interaction would not ignore one of the three independent variables
in case of a 2x2x2 design. The analysis would be termed as a complex
factorial analysis.
It may, however, be remembered that the complex factorial design
need not necessarily be of 2x2x2 type design, but can be generalized to
any number and combinations of experimental and control independent
variables. Of course, the greater the number of independent variables
included in a complex factorial design, the higher the order of the
interaction analysis possible. But the overall task goes on becoming more
and more complicated with the inclusion of more and more independent
variables in our design.
Factorial designs are used mainly because of the two advantages -
(i) They provide equivalent accuracy (as happens in the case of experiments
with only one factor) with less labour and as such are source of economy.
Using factorial designs, we can determine the effects of two (in simple
factorial design) or more (in case of complex factorial design) factors
(or variables) in one single experiment. (ii) they permit various other
comparisons of interest. For example, they give information about such
effects which cannot be obtained by treating one single factor at a time.
The determination of interaction effects is possible in case of factorial
designs.
87
Conclusion
There are several research designs and the researcher must decide
in advance of collection and analysis of data as to which design would prove
to be more appropriate for his research project. He must give due weight
to various points such as type of universe and it’s nature, the objective of
the study, the source list or the sampling frame, desired standard accuracy
and the like when taking a decision in respect of the design for his research
project.
SUMMARY
Experiment is the process of examining the truth of a statistical
hypothesis related to some research problem. There are two types of
experiments - absolute and comparative. There are three types of research
designs - research design for descriptive and diagnostic research, research
design for exploratory research studies and research design for hypothesis
testing. Prof. Fisher has laid three principles of experimental design. They
are Principle of Replication, Principle of Randomization and Principle of
Local Control. There are different kinds of experimental designs. Some of
them are Informal experimental design, After only with control design,
Formal experimental design, Completely randomized design, Randomized
block design, Latin square design and Factorial design.
SELF ASSESMENT QUESTIONS (SAQS)
1. Explain the meaning and types of experiment (Ref. Introduction and
types of research design next to introduction)
2. Explain informal designs. (Ref. i,ii,iii in informal experiment design
portion.)
3. Explain formal experimental design and control. (Ref. i,ii,iii,iv in
formal experiment design section)
4. Explain complex factorial design.
***
88CHAPTER II
Lesson 4. Observation
1 Steps In Observation
Meaning And Characteristics Of Observation
Types Of Observation
Stages Of Observation
Problems, Merits And Demerits
Introduction
Observation is a method that employs vision as its main means
of data collection. It implies the use of eyes rather than of ears and the
voice. It is accurate watching and noting of phenomena as they occur
with regard to the cause and effect or mutual relations. It is watching
other persons’ behavior as it actually happens without controlling it. For
example, watching bonded labourer’s life, or treatment of widows and
their drudgery at home, provide graphic description of their social life and
sufferings. Observation is also defined as “a planned methodical watching
that involves constraints to improve accuracy”.
89
CHARACTERISTICS OF OBSERVATION
Scientific observation differs from other methods of data collection
specifically in four ways: (i) observation is always direct while other
methods could be direct or indirect; (ii) field observation takes place in
a natural setting; (iii) observation tends to be less structured; and (iv) it
makes only the qualitative (and not the quantitative) study which aims at
discovering subjects’ experiences and how subjects make sense of them
(phenomenology) or how subjects understand their life (interpretivism).
Lofland (1955) has said that this method is more appropriate
for studying lifestyles or sub-cultures, practices, episodes, encounters,
relationships, groups, organizations, settlements and roles etc. Black and
Champion (1976) have given the following characteristics of observation:
ӹ Behavior is observed in natural surroundings.
ӹ It enables understanding significant events affecting social relations
of the participants.
ӹ It determines reality from the perspective of observed person himself.
ӹ It identifies regularities and recurrences in social life by comparing
data in our study with that of other studies.
Besides, four other characteristics are:
ӹ Observation involves some control pertaining to the observation
and to the means he uses to record data. However, such controls do
not exist for the setting or the subject population.
ӹ It is focused on hypotheses-free inquiry.
ӹ It avoids manipulations in the independent variable i.e., one that is
supposed to cause other variable(s) and is not caused by them.
ӹ Recording is not selective.
Since at times, observation technique is indistinguishable from
experiment technique, it is necessary to distinguish the two.
90
Observation involves few controls than the experiment technique.
1. The behaviour observed in observation is natural, whereas in
experiment it is not always so.
2. The behavior observed in experiment is more molecular (of a smaller
unit), while one in observation is molar.
3. In observation, fewer subjects are watched for long periods of time in
more varied circumstances than in experiment.
4. Training required in observation study is directed more towards
sensitizing the observer to the flow of events, whereas training in
experiments serves to sharpen the judgment of the subject.
5. In observational study, the behavior observed is more diffused.
Observational methods differ from one another along several variables
or dimensions.
Merits:
1. We get original data
2. We get more accurate and reliable data.
3. Satisfactory information can be extracted by the investigator
through indirect questions.
4. Data are homogeneous and comparable.
5. Additional information can be gathered.
6. Misinterpretation of questions can be avoided.
Demerits:
1. It is time consuming and costs more.
SELF ASSESSMENT QUESTIONS (SAQS)
1. What are the characteristics of observation?
2. How do you differentiate observation from experiment?
----
91
92
CHAPTER– III
Statistical Analysis
CONTENTS
1. Probability
2. Probability Distribution
2.1 Binomial Distribution
2.2 Poisson Distribution
2.3 Normal Distribution
3. Testing of Hypothesis
3.1 Small Sample
3.2 Large Sample Test
4. Χ2 Test
1.
PROBABILITY
If an experiment is repeated under essentially homogeneous and
similar conditions, two possible conclusions can be arrived. They are: the
results are unique and the outcome can be predictable and result is not
unique but may be one of the several possible outcomes. In this context,
it is better to understand various terms pertaining to probability before
examining the probability theory. The main terms are explained as follows:
(i)
Random Experiment
An experiment which can be repeated under the same conditions
and the outcome cannot be predicted under any circumstances is known
93
as random experiment. For example: An unbiased coin is tossed. Here
we are not in a position to predict whether head or tail is going to occur.
Hence, this type of experiment is known as random experiment.
(ii)
Sample Space
A set of possible outcomes of a random experiment is known as
sample space. For example, in the case of tossing of an unbiased coin twice,
the possible outcomes are HH, HT, TH and TT. This can be represented in
a sample space as S= (HH, HT, TH, TT).
(iii) An Event
Any possible outcomes of an experiment are known as an event. In
the case of tossing of an unbiased coin twice, HH is an event. An event
can be classified into two. They are: (a) Simple events, and (ii) Compound
events. Simple event is an event which has only one sample point in the
sample space. Compound event is an event which has more than one
sample point in the sample space. In the case of tossing of an unbiased
coin twice HH is a simple event and TH and TT are the compound events.
(iv) Complementary Event
A and A’ are the complementary event if A’ consists of all those
sample point which is not included in A. For instance, an unbiased
dice is thrown once. The probability of an odd number turns up are
complementary to an even number turns up. Here, it is worth mentioning
that the probability of sample space is always is equal to one. Hence, the P
(A’) = 1 - P (A).
(v)
Mutually Exclusive Events
A and B are the two mutually exclusive events if the occurrence of
A precludes the occurrence of B. For example, in the case of tossing of
an unbiased coin once, the occurrence of head precludes the occurrence
of tail. Hence, head and tail are the mutually exclusive event in the case
of tossing of an unbiased coin once. If A and B are mutually exclusive
events, then the probability of occurrence of A or B is equal to sum of their
individual probabilities. Symbolically, it can be presented as:
94
P (A U B) = P (A) + P (B)
If A and B is joint sets, then the addition theorem of probability can be
stated as:
P (A U B ) = P(A) + P(B) - P(AB)
(vi) Independent Event
A and B are the two independent event if the occurrence of A does
not influence the occurrence of B. In the case of tossing of an unbiased
coin twice, the occurrence of head in the first toss does not influence the
occurrence of head or tail in the toss. Hence, these two events are called
independent events. In the case of independent event, the multiplication
theorem can be stated as the probability of A and B is the product of their
individual probabilities. Symbolically, it can be presented as:-
P (A B) = P (A) * P (B)
Addition Theorem of Probability
Let
A and B be the two mutually exclusive events, then the
probabi