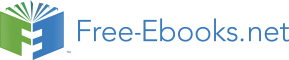

observed and expected frequencies, the greater shall be the value of χ2.
The computed value of χ2 is compared with the table value of χ2 for
given degrees of freedom at a certain specified level of significance. If at
the stated level, the calculated value of χ2 is less than the table value, the
difference between theory and observation is not considered as significant.
114
The following observation may be made with regard to the χ2 distribution:-
i. The sum of the observed and expected frequencies is always zero.
Symbolically, ∑(O – E) = ∑O - ∑E
= N – N = 0
ii. The χ2 test depends only on the set of observed and expected frequencies
and on degrees of freedom v. It is a non-parametric test.
iii. χ2 distribution is a limiting approximation of the multinomial
distribution.
iv. Even though χ2 distribution is essentially a continuous distribution it
can be applied to discrete random variables whose frequencies can be
counted and tabulated with or without grouping.
The Chi-Square Distribution
For large sample sizes, the sampling distribution of χ2 can be closely
approximated by a continuous curve known as the Chi-square distribution.
The probability function of χ2 distribution is:
F(χ2) = C (χ2)(v/2 – 1)e – x2/2
Where
e = 2.71828, v = number of degrees of freedom, C = a constant
depending only on v.
The χ2 distribution has only one parameter, v, the number of
degrees of freedom. As in case of t-distribution there is a distribution
for each different number of degrees of freedom. For very small number
of degrees of freedom, the Chi-square distribution is severely skewed
to the right. As the number of degrees of freedom increases, the curve
rapidly becomes more symmetrical. For large values of v the Chi-square
distribution is closely approximated by the normal curve.
115
The following diagram gives χ2 distribution for 1, 5 and 10 degrees of
freedom:
F(x2)
v = 1
v = 5
v = 10
0
2 4 6
8 10 12 14 16 18 20 22
χ2
χ2 Distribution
It is clear from the given diagram that as the degrees of freedom
increase, the curve becomes more and more symmetric. The Chi-square
distribution is a probability distribution and the total area under the curve
in each Chi-square distribution is unity.
Properties of χ2 Distribution
The main properties of χ2 distribution are:-
(i) The mean of the χ2 distribution is equal to the number of degrees
of freedom,
i.e.,
X = v
(ii) The variance of the χ2 distribution is twice the degrees of
freedom, Variance = 2v
(iii)
µ = 0,
1
(iv)
µ = 2v,
2
116
(v)
µ = 8v,
3
(vi)
µ = 48v + 12v2.
4
µ 2 64v2 8
3
(vii)
β = --- = ----- = --
1
µ 2 8v3 v
2
µ 48v + 12v2 12
4
(v)
β µ = ------ = --------------- = 3 + ---
1 3
µ 2 4v2 v
2
The table values of χ2 are available only up to 30 degrees of freedom.
For degrees of freedom greater than 30, the distribution of χ2 approximates
the normal distribution. For degrees of freedom greater than 30, the
approximation is acceptable close. The mean of the distribution √2χ2 is
√2v – 1, and the standard deviation is equal to 1. Thus the application of
the test is simple, for deviation of √2χ2 from √2v – 1 may be interpreted as
a normal deviate with units standard deviation. That is,
Z
=
√2χ2 - √ 2v – 1
Alternative Method Of Obtaining The Value of χ2
In a 2x2 table where the cell frequencies and marginal totals are as below:
a
b
(a+b)
c
d
(c+d)
(a+c)
(b+d)
N
N is the total frequency and ad the larger cross-product, the value
of χ2 can easily be obtained by the following formula:
117
N (ad – bc)2
χ2 = --------------------------------- or
(a + c) (b + d) (c + d) (a + b)
With Yate’s corrections
N (ab – bc - ½N)2
χ2 = -----------------------------------
(a + c) (b + d) (c + d) (a + b)
Conditions for Applying χ2 Test:
The main conditions considered for employing the χ2 test are:
(i) N must be to ensure the similarity between theoretically
correct distribution and our sampling distribution of χ2.
(ii) No theoretical cell frequency should be small when the expected
frequencies are too small. If it is so, then the value of χ2 will be overestimated
and will result in too many rejections of the null hypothesis. To avoid
making incorrect inferences, a general rule is followed that expected
frequency of less than 5 in one cell of a contingency table is too small to use.
When the table contains more than one cell with an expected frequency of
less than 5 then add with the preceding or succeeding frequency so that the
resulting sum is 5 or more. However, in doing so, we reduce the number of
categories of data and will gain less information from contingency table.
(iii) The constraints on the cell frequencies if any should be linear, i.e.,
they should not involve square and higher powers of the frequencies such
as ∑O = ∑E = N.
Uses of χ2 test:
The main uses of χ2 test are:
i. χ2 test as a test of independence. With the help of χ2 test, we can find
out whether two or more attributes are associated or not. Let’s assume
that we have n observations classified according to some attributes.
118
We may ask whether the attributes are related or independent. Thus,
we can find out whether there is any association between skin colour
of husband and wife. To examine the attributes that are associated,
we formulate the null hypothesis that there is no association against
an alternative hypothesis and that there is an association between the
attributes under study. If the calculated value of χ2 is less than the
table value at a certain level of significance, we say that the result of the
experiment provides no evidence for doubting the hypothesis. On the
other hand, if the calculated value of χ2 is greater than the table value
at a certain level of significance, the results of the experiment do not
support the hypothesis.
ii. χ2 test as a test of goodness of fit. This is due to the fact that it enables
us to ascertain how appropriately the theoretical distributions such as
binomial, Poisson, Normal, etc., fit empirical distributions. When an
ideal frequency curve whether normal or some other type is fitted to
the data, we are interested in finding out how well this curve fits with
the observed facts. A test of the concordance of the two can be made
just by inspection, but such a test is obviously inadequate. Precision
can be secured by applying the χ2 test.
iii. χ2 test as a test of homogeneity. The χ2 test of homogeneity is an
extension of the chi-square test of independence. Tests of homogeneity
are designed to determine whether two or more independent random
samples are drawn from the same population or from different
populations. Instead of one sample as we use with independence
problem we shall now have 2 or more samples. For example, we may be
interested in finding out whether or not university students of various
levels, i.e., middle and richer poor income groups are homogeneous in
performance in the examination.
Illustration:
In an anti-diabetes campaign in a certain area, a particular
medicine, say x was administered to 812 persons out of a total population
of 3248. The number of diabetes cases is shown below:
119
Treatment
Diabetes
No Diabetes Total
Medicine x
20
792
812
No Medicine x
220
2216
2436
Total
240
3008
3248
Discuss the usefulness of medicine x in checking malaria.
Solution:
Let us take the hypothesis that quinine is not effective in checking
diabetes. Applying χ2 test :
(A) X (B) 240 x 812
Expectation of (AB) = ------------ = ------------ = 60
N 3248
Or E , i.e., expected frequency corresponding to first row and first column
1
is 60. The bale of expected frequencies shall be:
60
752
812
180
2256
2436
240
3008
3248
O
E
(O – E)2
(O – E)2/E
20
60
1600
26.667
220
180
1600 8.889
792
752
1600 2.218
2216
2256
1600 0.709
[∑(O – E)2/E] = 38.593
120
χ2 = [∑(O – E)2/E] = 38.593
V = (r – 1) (c – 1) = (2 – 1) (2 – 1) = 1
For
v = 1, χ2 = 3.84
0.05
The calculated value of χ2 is greater than the table value. The hypothesis
is rejected. Hence medicine x is useful in checking malaria.
Illustration:
In an experiment on immunization of cattle from tuberculosis the
following results were obtained:
Affected
not affected
Inoculated
10
20
Not inoculated
15
5
Calculate χ2 and discuss the effect of vaccine in controlling susceptibility
to tuberculosis (5% value of χ2 for one degree of freedom = 3.84).
Solution:
Let us take the hypothesis that the vaccine is not effective in
controlling susceptibility to tuberculosis. Applying χ2 test:
N(ad – bc)2 50 (11x5 – 20x15)2
χ2 = -------------------------- = ------------------------ = 8.3
(a+b) (c+d)(a+c)(b+d) 30x20x25x25
Since the calculated value of χ2 is greater than the table value the hypothesis
is not true. We, therefore, conclude the vaccine is effective in controlling
susceptibility to tuberculosis.
***
121122
CHAPTER – IV
Statistical Applications
A BRIEF INTRODUCTION TO STATISTICAL APPLICATIONS
A manager in a business organization – whether in the top level,
or the middle level, or the bottom level - has to perform an important
role of decision making. For solving any organizational problem – which
most of the times happens to be complex in nature -, he has to identify
a set of alternatives, evaluate them and choose the best alternative. The
experience, expertise, rationality and wisdom gained by the manager over
a period of time will definitely stand in good stead in the evaluation of the
alternatives available at his disposal. He has to consider several factors,
sometimes singly and sometimes jointly, during the process of decision
making. He has to deal with the data of not only his organization but also
of other competing organizations.
It would be a challenging situation for a manager when he has
to face so many variables operating simultaneously, something internal
and something external. Among them, he has to identify the important
variables or the dominating factors and he should be able to distinguish
one factor from the other. He should be able to find which factors have
similar characteristics and which factors stand apart. He should be able to
know which factors have an inter play with each other and which factors
remain independent. It would be advantageous to him to know whether
there is any clear pattern followed by the variables under consideration.
At times he may be required to have a good idea of the values that the
variables would assume in future occasions. The task of a manager becomes
all the more difficult in view of the risks and uncertainties surrounding
the future events. It is imperative on the part of a manager to understand
the impact of various policies and programmes on the development of
the organization as well as the environment. Also he should be able to
understand the impact of several of the environmental factors on his
organization. Sometimes a manager has to take a single stage decision and
at times he is called for to take a multistage decision on the basis of various
factors operating in a situation. 123
Statistical analysis is a tool for a manager in the process of decision
making by means of the data on hand. All managerial activities involve
an analysis of data. Statistical approach would enable a manager to have a
scientific guess of the future events also. Statistical methods are systematic
and built by several experts on firmly established theories and consequently
they would enable a manager to overcome the uncertainties associated with
future occasions. However, statistical tools have their shortcomings too.
The limitations do not reflect on the subject. Rather they shall be traced
to the methods of data collection and recording of data. Even with highly
sophisticated statistical methods, one may not arrive at valid conclusions
if the data collected are devoid of representative character.
In any practical problem, one has to see whether the assumptions
are reasonable or not, whether the data represents a wide spectrum,
whether the data is adequate, whether all the conditions for the statistical
tests have been fulfilled, etc. If one takes care of these aspects, it would be
possible to arrive at better alternatives and more reliable solutions, thereby
avoiding future shocks. While it is true that a statistical analysis, by itself,
cannot solve all the problems faced by an organization, it will definitely
enable a manager to comprehend the ground realities of the situation. It
will for sure provide a foresight in the identification of the crucial variables
and the key areas so that he can locate a set of possible solutions within his
ambit. A manager has to have a proper blend of the statistical theories and
practical wisdom and he shall always strive for a holistic approach to solve
any organizational problem. A manager has to provide some safe-guarding
measures against the limitations of the statistical tools. In the process he
will be able to draw valid inferences thereby providing a clue as to the
direction in which the organization shall move in future. He will be ably
guided by the statistical results in the formulation of appropriate strategies
for the organization. Further, he can prepare the organization to face the
possible problems of business fluctuations in future and minimize the
risks with the help of the early warning signals indicated by the relevant
statistical tools.
A marketing manager of a company or a manager in a service
organization will have occasions to come across the general public and
consumers with several social and psychological variables which are
difficult to be measured and quantified.
124
Depending on the situation and the requirement, a manager may have
to deal with the data of just one variable (univariate data), or data on
two variables (bivariate data) or data concerning several simultaneous
variables (multivariate data).
The unit on hand addresses itself to the role of a manager as a
decision maker with the help of data available with him. Different statistical
techniques which are suitable for different requirements are presented
in this unit in a simple style. A manager shall know the strengths and
weaknesses of various statistical tools. He shall know which statistical
tool would be the most appropriate in a particular context so that the
organization will derive the maximum benefit out of it.
The interpretation of the results from statistical analysis occupies
an important place. Statistics is concerned with the aggregates and
not just the individual data items or isolated measurements of certain
variables. Therefore the conclusions from a statistical study will be valid
for a majority of the objects and normal situations only. There are always
extreme cases in any problem and they have to be dealt with separately.
Statistical tools will enable a manager to identify such outliers (abnormal
cases or extreme variables) in a problem. A manager has to evaluate the
statistical inferences, interpret them in the proper context and apply them
in appropriate situations.
While in an actual research problem, one has to handle a large
quantum of data, it is not possible to treat such voluminous data by
a beginner in the subject. Keeping this point in mind, any numerical
example in the present unit is based on a few data items only. It would be
worthwhile to the budding managers to make a start in solving statistical
problems by practicing the ones furnished in this unit.
The candidates are suggested to use hand calculators for solving
statistical problems. There will be frequent occasions to use statistical
tables of f-values furnished in this unit. The candidates are suggested to
have with them a copy of the tables for easy, ready reference. The books
and articles listed under the references may be consulted for further study
or applications of statistical techniques in relevant research areas.
***
125126
CHAPTER IV
1. Correlation And Regression Analysis
The Concept Of Correlation
Determination Of Simple Correlation Coefficient
Properties Of Correlation Coefficient
The Concept Of Rank Correlation
Determination Of Rank Correlation Coefficient
The Concept Of Regression
The Principle Of Least Squares
Normal Equations
Determination Of Regression Equations
127
SIMPLE CORRELATION
Correlation
Correlation means the average relationship between two or more
variables. When changes in the values of a variable affect the values of
another variable, we say that there is a correlation between the two
variables. The two variables may move in the same direction or in opposite
directions. Simply because of the presence of correlation between two
variables, we cannot jump to the conclusion that there is a cause-effect
relationship between them. Sometimes, it may be due to chance also.
Simple correlation
We say that the correlation is simple if the comparison involves
two variables only.
TYPES OF CORRELATION
Positive correlation
If two variables x and y move in the same direction, we say that
there is a positive correlation between them. In this case, when the value
of one variable increases, the value of the other variable also increases and
when the value of one variable decreases, the value of the other variable
also decreases. Eg. The age and height of a child.
Negative correlation
If two variables x and y move in opposite directions, we say that
there is a negative correlation between them. i.e., when the value of one
variable increases, the value of the other variable decreases and vice versa.
Eg. The price and demand of a normal good.
128
The following diagrams illustrate positive and negative correlations
between x and y.
y
y
x x
Positive Correlation
Negative Correlation
Perfect Positive Correlation
If changes in two variables are in the same direction and the changes
are in equal proportion, we say that there is a perfect positive correlation
between them.
Perfect Negative Correlation
If changes in two variables are in opposite directions and the
absolute values of changes are in equal proportion, we say that there is a
perfect negative correlation between them.
y
y
x