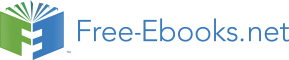

Algorithm: Quantum Simulation
Speedup: Superpolynomial
Description: It is believed that for any physically realistic Hamiltonian H on n degrees of freedom, the corresponding time evolution operator e−iHt can be implemented using poly(n,t) gates. Unless BPP=BQP, this problem is not solvable in general on a classical computer in polynomial time. Many techniques for quantum simulation have been developed for general classes of Hamiltonians [25,95,92,5,12,170,205,211,244,245,278,293,294,295,372,382], chemical dynamics [63,68,227,310,375], condensed matter physics [1,99, 145], relativistic quantum mechanics (the Dirac and Klein-Gordon equations) [367,369,370,371], open quantum systems [376, 377,378,379], and quantum field theory [107,166,228,229,230,368]. The exponential complexity of classically simulating quantum systems led Feynman to first propose that quantum computers might outperform classical computers on certain tasks [40]. Although the problem of finding ground energies of local Hamiltonians is QMA-complete and therefore probably requires exponential time on a quantum computer in the worst case, quantum algorithms have been developed to approximate ground [102,231,232,233,234,235,308,321,322,380,381] and thermal [132,121,281,282,307] states for some classes of Hamiltonians. Efficient quantum algorithms have been also obtained for preparing certain classes of tensor network states [323,324,325,326,327,328].
Algorithm: Knot Invariants
Speedup: Superpolynomial
Description: As shown by Freedman [42, 41], et al., finding a certain additive approximation to the Jones polynomial of the plat closure of a braid at ei2π/5 is a BQP-complete problem. This result was reformulated and extended to ei2π/k for arbitrary k by Aharonov et al. [4,2]. Wocjan and Yard further generalized this, obtaining a quantum algorithm to estimate the HOMFLY polynomial [93], of which the Jones polynomial is a special case. Aharonov et al. subsequently showed that quantum computers can in polynomial time estimate a certain additive approximation to the even more general Tutte polynomial for planar graphs [3]. It is not fully understood for what range of parameters the approximation obtained in [3] is BQP-hard. (See also partition functions.) Polynomial-time quantum algorithms have also been discovered for additively approximating link invariants arising from quantum doubles of finite groups [174]. (This problem is not known to be BQP-hard.) As shown in [83], the problem of finding a certain additive approximation to the Jones polynomial of the trace closure of a braid at ei2π/5 is DQC1-complete.
Algorithm: Three-manifold Invariants
Speedup: Superpolynomial
Description: The Turaev-Viro invariant is a function that takes three-dimensional manifolds as input and produces a real number as output. Homeomorphic manifolds yield the same number. Given a three-manifold specified by a Heegaard splitting, a quantum computer can efficiently find a certain additive approximation to its Turaev-Viro invariant, and this approximation is BQP-complete [129]. Earlier, in [114], a polynomial-time quantum algorithm was given to additively approximate the Witten-Reshitikhin-Turaev (WRT) invariant of a manifold given by a surgery presentation. Squaring the WRT invariant yields the Turaev-Viro invariant. However, it is unknown whether the approximation achieved in [114] is BQP-complete. A suggestion of a possible link between quantum computation and three-manifold invariants was also given in [115].
Algorithm: Partition Functions
Speedup: Superpolynomial
Description: For a classical system with a finite set of states S the partition function is Z=∑s∈Se−E(s)/kT, where T is the temperature and k is Boltzmann's constant. Essentially every thermodynamic quantity can be calculated by taking an appropriate partial derivative of the partition function. The partition function of the Potts model is a special case of the Tutte polynomial. A quantum algorithm for approximating the Tutte polynomial is given in [3]. Some connections between these approaches are discussed in [67]. Additional algorithms for estimating partition functions on quantum computers are given in [112,113,45,47]. A BQP-completeness result (where the "energies" are allowed to be complex) is also given in [113]. A method for approximating partition functions by simulating thermalization processes is given in [121]. A quadratic speedup for the approximation of general partition functions is given in [122]. A method based on quantum walks, achieving polynomial speedup for evaluating partition functions is given in [265].
Algorithm: Adiabatic Algorithms
Speedup: Unknown
Description: In adiabatic quantum computation one starts with an initial Hamiltonian whose ground state is easy to prepare, and slowly varies the Hamiltonian to one whose ground state encodes the solution to some computational problem. By the adiabatic theorem, the system will track the instantaneous ground state provided the variation of the Hamiltonian is sufficiently slow. The runtime of an adiabatic algorithm scales at worst as 1/γ3 where γ is the minimum eigenvalue gap between the ground state and the first excited state [185]. If the Hamiltonian is varied sufficiently smoothly, one can improve this to O˜(1/γ2) [247]. Adiabatic quantum computation was first proposed by Farhi et al. as a method for solving NP-complete combinatorial optimization problems [96, 186]. Adiabatic quantum algorithms for optimization problems typically use "stoquastic" Hamiltonians, which do not suffer from the sign problem. Such algorithms are sometimes referred to as quantum annealing. Adiabatic quantum computation with non-stoquastic Hamiltonians is as powerful as the quantum circuit model [97]. Adiabatic algorithms using stoquastic Hamiltonians are probably less powerful [183], but may be nevertheless more powerful than classical computation. The asymptotic runtime of adiabatic optimization algorithms is notoriously difficult to analyze, but some progress has been achieved [179,180,181,182,187,188,189,190,191,226]. (Also relevant is an earlier literature on quantum annealing, which originally referred to a classical optimization algorithm that works by simulating a quantum process, much as simulated annealing is a classical optimization algorithm that works by simulating a thermal process. See e.g. [199, 198].) Adiabatic quantum computers can perform a process somewhat analogous to Grover search in O(N−−√) time [98]. Adiabatic quantum algorithms achieving quadratic speedup for a more general class of problems are constructed in [184] by adapting techniques from [85]. Adiabatic quantum algorithms have been proposed for several specific problems, including PageRank [176], machine learning [192, 195], and graph problems [193, 194]. Some quantum simulation algorithms also use adiabatic state preparation.
Algorithm: Quantum Approximate Optimization
Speedup: Superpolynomial
Description: For many combinatorial optimization problems, finding the exact optimal solution is NP-complete. There are also hardness-of-approximation results proving that finding an approximation with sufficiently small error bound is NP-complete. For certain problems there is a gap between the best error bound achieved by a polynomial-time classical approximation algorithm and the error bound proven to be NP-hard. In this regime there is potential for exponential speedup by quantum computation. In [242] a new quantum algorithmic technique called the Quantum Approximate Optimization Algorithm (QAOA) was proposed for finding approximate solutions to combinatorial optimization problems. In [243] it was subsequently shown that QAOA solves a combinatorial optimization problem called Max E3LIN2 with a better approximation ratio than any polynomial-time classical algorithm known at the time. However, an efficient classical algorithm achieving an even better approximation ratio (in fact, the approximation ratio saturating the limit set by hardness-of-approximation) was subsequently discovered [260]. Presently, the power of QAOA relative to classical computing is an active area of research [300, 301, 302, 314].
Algorithm: Semidefinite Programming
Speedup: Superpolynomial
Description: Given a list of m + 1 Hermitian n×n matrices C,A1,A2,…,Am and m numbers b1,…,bm, the problem of semidefinite programming is to find the positive semidefinite n×n matrix X that maximizes tr(CX) subject to the constraints tr(AjX)≤bj for j=1,2,…,m. Semidefinite programming has many applications in operations research, combinatorial optimization, and quantum information, and it includes linear programming as a special case. Improving upon [313], a quantum algorithm is given in [383] that approximately solves semidefinite programs to within ±ϵ in time O˜(m−−√logm⋅poly(logn,r,ϵ−1)), where r is the rank of the semidefinite program. This constitutes a quadratic speedup over the fastest classical algorithms when r is small compared to n. The quantum algorithm is based on amplitude amplification and quantum Gibbs sampling [121, 307].
Algorithm: Zeta Functions
Speedup: Superpolynomial
Description: Let f(x,y) be a degree-d polynomial over a finite field Fp. Let Nr be the number of projective solutions to f(x,y = 0 over the extension field Fpr. The zeta function for f is defined to be Zf(T)=exp(∑∞r=1NrrTr). Remarkably, Zf(T) always has the form Zf(T)=Qf(T)(1−pT)(1−T) where Qf(T) is a polynomial of degree 2g and g=12(d−1)(d−2) is called the genus of f. Given Zf(T) one can easily compute the number of zeros of f over any extension field Fpr. One can similarly define the zeta function when the original field over which f is defined does not have prime order. As shown by Kedlaya [64], quantum computers can determine the zeta function of a genus g curve over a finite field Fpr in poly(logp,r,g) time. The fastest known classical algorithms are all exponential in either log(p) or g. In a diffent, but somewhat related contex, van Dam has conjectured that due to a connection between the zeros of Riemann zeta functions and the eigenvalues of certain quantum operators, quantum computers might be able to efficiently approximate the number of solutions to equations over finite fields [87].
Algorithm: Weight Enumerators
Speedup: Superpolynomial
Description: Let C be a code on n bits, i.e. a subset of Zn2. The weight enumerator of C is SC(x,y)=∑c∈Cx|c|yn−|c| where |c| denotes the Hamming weight of c. Weight enumerators have many uses in the study of classical codes. If C is a linear code, it can be defined by C={c:Ac=0} where A is a matrix over Z2 In this case SC(x,y)=∑c:Ac=0x|c|yn−|c|. Quadratically signed weight enumerators (QWGTs) are a generalization of this: S(A,B,x,y)=∑c:Ac=0(−1)cTBcx|c|yn−|c|. Now consider the following special case. Let A be an
Reads:
115
Pages:
176
Published:
Apr 2023
"No Filter, No Problem" by Famium is your ultimate guide to creating a visually stunning, engaging Instagram presence. Packed with insider secrets and practic...
Formats: PDF, Epub, Kindle, TXT