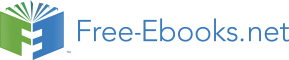

0.3sin( )
L
F
t and initial values are:
x(0) 0, x(0) 0, (0
z ) 0 . The objective of the positioning is to drive the displacement signal
x to track the reference trajectory which is shown in Fig.2.
The parameters of piezo-positioning mechanism are given in Table 1.
0
50000 N / m
4
Ns m
1
5 10 Ns / m
2
0.4
/
f 1
C
N
f
1.5
S
N
x
0.001 m /
S
s
M 1 kg
D 0.0015 Ns / m
0.3sin( )
L
F
t
Table 1. Piezoelectric Parameters
106
Applications of Nonlinear Control
15
10
Actual Position
eter)
5
m
icro
0
ent (M
-5
splacemDi
Reference
-10
-150
0.2
0.4
0.6
0.8
1
Time (Sec)
Fig. 2. Desired and Actual Piezoelectric displacement.
400
ec)
200
S
eter/
0
icrom
-200
elocity (MV
-4000
0.2
0.4
0.6
0.8
1
Time (Sec)
Fig. 3. Piezoelectric velocity.
A Robust Motion Tracking Control
of Piezo-Positioning Mechanism with Hysteresis Estimation
107
As depicted from the results, especially Fig.4, the positioning mechanism has a good
accuracy and the error between displacement and reference signal at least 4 orders is smaller
than the actual signal which means that the error is about %0.01.
5
eter)m
ano
rror (N
0
E
ent
isplacemD
-50
0.2
0.4
0.6
0.8
1
Time (Sec)
Fig. 4. Error between actual & desired displacement.
For the purpose of study the behavior of the controller in the presence of parameter
uncertainties, by considering the M
0.1 M ,
D
0.1 D and
0.4
L
F
and using
x(0) 15( m), x(0) 0, (
z 0) 0 as the initial values, we have performed another test. The
result of this test is compared with the result of the method presented in [19]. In the
simulation we consider the control gain is 2000.
As it can be seen from Fig.5 and Fig.7, the presented method has an acceptable response
and the positioning error is in about %0.25. Also, the hysteresis and disturbance voltage
and its estimation are shown in Fig.9. As it can be seen from this result, the hysteresis
identifier can estimate the hysteresis voltage precisely. For the purpose of comparison the
accuracy of the proposed method with the recently proposed method in [19], we simulate
the piezo-positioning mechanism with the method of [19]. The displacement error of [19]
is shown in Fig.10. Noted that in the simulation of [19] we use these control gains:
5
k 10 , k 9000, k 50
p
v
s
and
10 . Comparison of Fig.7 and Fig.10 depicts that the
error in the presented method is smaller than the method of [19]. Also, it is clear that in
addition of smaller number of control gains, the gain of the controller in the presented
method in comparison with [19] is very smaller. Experimental implementation of the high
gain needs more complexity and also it may be generate noise. Although method of [19] is
108
Applications of Nonlinear Control
30
25
Reference
eter) 20
Actual Position
icromM 15
ent (m
lace 10
ispD
5
00
0.1
0.2
0.3
0.4
0.5
Time (Sec)
Fig. 5. Displacement of piezoelectric.
800
ec)
400
S
eter/m
0
icro
-400
elocity (MV
-8000
0.1
0.2
0.3
0.4
0.5
Time (Sec)
Fig. 6. Velocity of piezoelectric.
A Robust Motion Tracking Control
of Piezo-Positioning Mechanism with Hysteresis Estimation
109
.15
.1
eter)m
icro
.05
Mr (
rro
0
ent E -.05
-.1
isplacemD
-.150
0.1
0.2
0.3
0.4
0.5
Time (Sec)
Fig. 7. Error between actual & desired displacement.
1
0.5
)V (
nput
0
rol I
ontC
-0.5
-10
0.1
0.2
0.3
0.4
0.5
Time (Sec)
Fig. 8. Control input.
110
Applications of Nonlinear Control
1
)
0.5
oltage (V
e Vnc
0
sturba
Di
Error
-0.5
ysteresis &H
-10
0.05
0.1
0.15
0.2
0.25
0.3
0.35
0.4
0.45
0.5
Time (Sec)
Fig. 9. Estimated & actual values of hysteresis and disturbance voltages and error between
them.
.5
eter)m
icro
(Mr
rro
0
ent E
splacemDi
.50
0.1
0.2
0.3
0.4
0.5
Time (Sec)
Fig. 10. Displacement Error of the method presented in [19].
A Robust Motion Tracking Control
of Piezo-Positioning Mechanism with Hysteresis Estimation
111
robust against disturbances and uncertainties and in comparison with other methods has
higher precision, it needs high value gains to perform this task. While the proposed
method can perform these tasks with lower cost and also it has the capability of hysteresis
estimation.
For the comparison between the presented method and the method of [19] another
simulation test is carried out. In this case M
0.2 M , D
0.2 D and
0.4
L
F
. The result is
shown in Fig.11.
As it can be depicted the presented method has the better response and using the proposed
controller the error is decreased. Note that in this case the reference is similar to the
Fig.5.
The last simulation is devoted to the reference signal of Fig.2 by considering M
0.2 M ,
D
0.2 D and
0.4
L
F
. Also in this case the error of the proposed controller is less than
the method of [19].
Error of [19]
0.3
0.2
eter)
0.1
icrom
r (M
0
rro
Error of
E
Proposed
-0.1
Method
-0.2
0
0.2
0.4
0.6
0.8
1
Time (Sec)
Fig. 11. Comparison between the displacement error of the presented controller and the
controller proposed in [19].
112
Applications of Nonlinear Control
0.3
Error of [19]
0.2
0.1
Error of
Proposed
Method
eter)
0
icrom
r (M -0.1
rroE
-0.2
-0.3
-0.4
0.2
0.6
1
1.4
1.8
Time (Sec)
Fig. 12. Comparison between the displacement error of the presented controller and the
controller proposed in [19].
7. Conclusion
A robust tracking motion controller is designed to control a positioning of piezoelectric
actuator system with hysteresis phenomenon. The Lugre hysteresis model is used to model
the nonlinearities in the system under study. Using the presented controller, we can
estimate the nonlinear hysteresis and disturbances imposed to the piezoelectric actuator and
compensate their effects. The performance and efficiency of the designed controller for a
positioning system is compared with recently proposed robust method. The results obtained
depict the validity and performance of the presented approach.
8. References
[1] Spanner, K. and S. Vorndran, 2003. Advances in piezo-nanopositioning technology.
Proc. IEEE/ASME int. conf. advanced intelligent mechatronics, Kobe,
Japan.
[2] Yi B.J., G.B. Chung, H.Y. Na, W.K. Kim and I.H. Suh, 2003. Design and experiment of a
3-DOF parallel micromechanism utilizing flexurehinges. IEEE Trans Robotics
Automation, 19(4): 604–12.
A Robust Motion Tracking Control
of Piezo-Positioning Mechanism with Hysteresis Estimation
113
[3] EI Rifai, O.M., 2002. Modeling and control of undesirable dynamics in atomic force
microscopes. PhD dissertation, MIT, February.
[4] Hung, X.C. and D.Y. Lin, 2003. Tracking control of a piezoelectric actuator based on
experiment hysteretic modeling. Proc. Of the IEEE/ASME Int. Conf. Advanced
Intelligent Mechatronics, Kobe, Japan.
[5] Ge, P. and M. Jouaneh, 1997. Generalized priesach model for hysteresis nonlinearity of
piezoceramic actuators. Precision Eng., 20: 99-111.
[6] Richter, H., E.A. Misawa, D.A. Lucca and H. Lu, 2001. Modeling nonlinear behavior in a
piezoelectric actuator. Prec. Eng. J., 25: 128-137.
[7] Krasnosellskii, M.A. and A.V. Pokrovskii, 1989. Systems with hysteresis, Springer-
Verlag, Berlin.
[8] Stepanenko, Y. and C.Y. Su, 1998. Intelligent control of piezoelectric actuators. Proc.
IEEE Conf. Decision & Control, 4234-4239.
[9] Wen, Y.K. 1976. Method for random vibration of hysteresis system. J. Eng. Mechanics
Division, 102: 249-263.
[10] Zhou, J., C. Wen and C. Zhang, 2007. Adaptive backstepping control of piezo-
positioning mechanism with hysteresis. Trans. CSME, 31(1): 97-110.
[11] Yavari, F., M.J. Mahjoob, and C. Lucas, 2007. Fuzzy control of piezoelectric actuators
with hysteresis for nanopositioning. 13th IEEE/IFAC Int. Conf. on Methods and
Models in Automation and Robotics, 685-690, August.
[12] Xu, J.H. 1993. Neural network control of a piezo tool positioner. Canadian Conf. Elect.
& Comp. Eng., 1: 333-336.
[13] Jung, S.B. and S.W. Kim, 1994. Improvement of scanning accuracy of pzt piezoelectric
actuator by feed-forward model-reference control. Precision Engineering, 16: 49-55.
[14] Tao, G. and P.V. Koktokovic, 1995. Adaptive control of plants with unknown hysteresis.
IEEE Transaction on Auotomatic Control, 40: 200-212.
[15] Huwang, L. and C. Jans, 2003. A reinforcement discrete neuro-adaptive control of
unknown piezoelectric actuator systems with dominant hysteresis. IEEE Trans.
Neural Networks, 14: 66-78.
[16] Lin, F.J., H.J. Shieh, and P.K. Huang, 2006. Adaptive wavelet neural network control
with hystereis estimation for piezo-positioning mechanism. IEEE Trans. Neural
Networks, 17: 432-444.
[17] Jan, C. and C.L. Hwang, 2004. A nonlinear observer-based sliding-mode control for
piezoelectric actuator systems: theory and experiments. J Chinese Inst. Eng.,27(1):
9-22.
[18] Shieh H.J., F.J. Lin, P.K. Huang, and L.T. Teng, 2004. Adaptive tracking control solely
using displacement feedback for a piezo-positioning mechanism. IEE Proc Control
Theory Appl,151(5): 653–60.
[19] Liaw, H.C., B. Shirinzadeh, and J. Smith, 2008. Robust motion traking control of piezo-
driven flexure-based four bar mehanism for micro/nano manipulation.
Mechatronics, 18: 111-120.
[20] Yannier, S. and A. Sabanovic, 2007. Continuous Time Controller Based on SMC and
Disturbance Observer for Piezoelectric Actuators. Int. Rev. Elect. Eng (I.R.E.E.), 3 (6).
114
Applications of Nonlinear Control
[21] Spooner, J.T., M. Maggiore, R. Ordonez, and K.M. Passino, 2002. Stable Adaptive
Control and Estimation for Nonlinear Systems. John Wiley and Sons, Inc, NY.
0
7
Nonlinear Observer-Based
Control Allocation
Fang Liao1, Jian Liang Wang2 and Kai-Yew Lum1
1 National University of Singapore
2 Nanyang Technological University
Singapore
1. Introduction
Control allocation is the process of mapping virtual control inputs (such as torque and force)
into actual actuator deflections in the design of control systems (Benosman et al., 2009;
Bodson, 2002; Buffington et al., 1998; Liao et al., 2007; 2010). Essentially, it is considered as
a constrained optimization problem as one usually wants to fully utilize all actuators in order
to minimize power consumption, drag and other costs related to the use of control, subject to
constraints such as actuator position and rate limits. In the design of control allocation, full
state information is required. However, in practice, states may not be measurable. Hence,
estimation of these unmeasurable states becomes inevitable.
The unmeasurable states are generally estimated based on available measurements and
the knowledge of the physical system. For linear systems, the property of observability
guarantees the existence of an observer. Luenberger or Kalman observers are known to give
a systematic solution (Luenberger, 1964). In the case of nonlinear systems, observability in
general depends on the input of the system. In other words, observability of a nonlinear
system does not exclude the existence of inputs for which two distinct initial states generate
identical measured outputs. Hence, in general, observer gains can be expected to depend on
the applied input (Nijmeijer & Fossen, 1999). This makes the design of a nonlinear observer
for a general nonlinear system a challenging problem. Although various results have been
proposed over the past decades (Ahmed-Ali & Lamnabhi-Lagarrigue, 1999; Alamir, 1999;
Besancon, 2007; Besancon & Ticlea, 2007; Bestle & Zeitz, 1983; Bornard & Hammouri, 1991;
Gauthier & Kupka, 1994; Krener & Isidori, 1983; Krener & Respondek, 1985; Michalska &
Mayne, 1995; Nijmeijer & Fossen, 1999; Teel & Praly, 1994; Tsinias, 1989; 1990; Zimmer, 1994),
none of them can claim to provide a general solution with the same convergence properties as
in the linear case.
Over the past decades, a variety of methods have been developed for constructing nonlinear
observers for nonlinear systems (Ahmed-Ali & Lamnabhi-Lagarrigue, 1999; Alamir, 1999;
Besancon, 2007; Besancon & Ticlea, 2007; Bestle & Zeitz, 1983; Bornard & Hammouri, 1991;
Gauthier & Kupka, 1994; Krener & Isidori, 1983; Krener & Respondek, 1985; Michalska &
Mayne, 1995; Nijmeijer & Fossen, 1999; Teel & Praly, 1994; Tsinias, 1989; 1990; Zimmer,
1994). They may be classified into optimization-based methods (Alamir, 1999; Michalska
116
Applications of Nonlinear Control
2
Will-be-set-by-IN-TECH
& Mayne, 1995; Zimmer, 1994) and feedback-based methods (Bestle & Zeitz, 1983; Bornard
& Hammouri, 1991; Gauthier & Kupka, 1994; Krener & Isidori, 1983; Krener & Respondek,
1985; Teel & Praly, 1994; Tsinias, 1989; 1990). Optimization-based methods obtain an estimate
ˆ x( t) of the state x( t) by searching for the best estimate ˆ x(0) of x(0) (which can explain the evolution y( τ) over [0, t]) and integrating the deterministic nonlinear system from ˆ x(0)
and under u( τ). These methods take advantage of their systematic formulation, but suffer
from usual drawbacks of nonlinear optimization (like computation burden, local minima,
and so on).
Feedback-based methods can correct on-line the estimation ˆ x( t) from the
error between the measurement output and the estimated output. These methods include
linearization methods (Bestle & Zeitz, 1983; Krener & Isidori, 1983; Krener & Respondek,
1985), Lyapunov-based approaches (Tsinias, 1989; 1990), sliding mode observer approaches
(Ahmed-Ali & Lamnabhi-Lagarrigue, 1999) and high gain observer approaches (Bornard &
Hammouri, 1991; Gauthier & Kupka, 1994; Teel & Praly, 1994), and so on. Among them,
linearization methods (Krener & Isidori, 1983) transform nonlinear systems into linear systems
by change of state variables and output injection. It is applicable to a special class of nonlinear
systems. Sliding mode observer approaches (Ahmed-Ali & Lamnabhi-Lagarrigue, 1999) is
to force the estimation error to join a stabilizing variety. The difficulty is to find a variety
attainable and having this property. High gain observer approaches (Besancon, 2007) use the
uniform observability and weight a gain based on the linear part so as to make the linear
dynamics of the observer error to dominate the nonlinear one. Due to the requirement of the
uniform observability, these approaches can only be applied to a class of nonlinear systems
with special structure. Interestingly, Lyapunov-based approaches (Tsinias, 1989; 1990) provide
a general sufficient Lyapunov condition for the observer design of a general class of nonlinear
systems and the proposed observer is a direct extension of Luenberger observer in linear case.
In this chapter, we extend the control allocation approach developed in (Benosman et al., 2009;
Liao et al., 2007; 2010) from state feedback to output feedback and adopt the Lyapunov-type
observer for a general class of nonlinear systems in (Tsinias, 1989; 1990) to estimate the
unmeasured states. Sufficient Lyapunov-like conditions in the form of the dynamic update
law are proposed for the control allocation design via output feedback.
The proposed
approach ensures that the estimation error and its rate converge exponentially to zero as
t → +∞ and the closed-loop system exponentially converges to the stable reference model
as t → +∞. The advantage of the proposed approach is that it is applicable to a wide class
of nonlinear systems with unmeasurable states, and it is computational efficiency as it is not
necessary to optimize the control allocation problem exactly at each time instant.
This chapter is organized as follows. In Section 2, the observer-based control allocation
problem is formulated where the control allocation design is based on the estimated states
which exponentially converge to the true states as t → +∞. In Section 3, the main result of
the observer-based control allocation design is presented in the form of dynamic update law.
An illustrative example is given in Section 4, followed by some conclusions in Section 5.
Throughout this chapter, given a real map f (v, w), (v, w) ∈ R n × R m, Dv f (v0, w0) denotes its derivative with respect to v at the point (v0, w0). For given real map h(v) with v ∈ R n, Dh(v0) denotes its derivative with respect to v at the point v0. In addition, · represent the induced 2-norm.