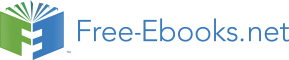

|a 1 | ≤ ¯ μ 1 = 2.2, |a 2 | ≤ ¯ μ 2 = 1.1, |b| ≥ bmn = 1.8
(86)
|d| ≤ μd fd, μd = 0.22, fd = |y|
(87)
where fd is not constant and known, whereas bmn, ¯ μ 1, ¯ μ 2, μd, bmn are positive, constant and unknown to the controller. From (86), (87) it follows that assumptions Ai, Aiv, Av of section 2
are satisfied.
The procedure of section 4 is followed in order to establish the terms involved in the control
and update laws, mentioned in remark 5. Eq. (80) can be rewritten as:
˙ x 1 = x 2
(88)
˙ x 2 = γ 2 a + bu + d
(89)
x 1 = y, x 2 = ˙ y, n = 2
(90)
A Robust State Feedback Adaptive Controller
with Improved Transient Tracking Error Bounds for Plants with Unknown Varying Control Gain
93
A Robust State Feedback Adaptive Controller with Improved Transient Tracking Error Bounds for Plants with Unknown Varying Control Gain 15
since n = 2, the state transformation based on the backstepping procedure involves the steps
0, 1, 2.
Step 0. Let
z 1 = e = y − yd = x 1 − yd
(91)
as in (9).
Step 1. Differentiating (91) with respect to time and arranging, yields:
˙ z 1 = −c 1 z 1 + z 2
(92)
z 2 = x 2 + c 1 z 1 − ˙ yd
(93)
as in (11), (12).
Step 2. Since n = 2, the second step is the last one. Differentiating (93) with respect to time,
using (89) and arranging, yields:
˙ z 2 = ˙ x 2 + c 1 ˙ z 1 − ¨ yd
= γ 2 a + bu + d + c 1 ˙ z 1 − ¨ yd
= γ 2 a + bu + d + c 1( x 2 + ϕ 1) − ¨ yd
(94)
using the definitions (91), (93), yields:
˙ z 2 = γ 2 a + bu + d + ϕ 2
(95)
ϕ 2 = c 1( z 2 − c 1 z 1) − ¨ yd
(96)
notice that the form of (95), (96) is that of (17), (18), respectively. This completes the state
transformation based on the backstepping procedure.
The parameters defined above can be summarized as:
z 1 = y − yd
(97)
z 2 = x 2 + c 1 z 1 − ˙ yd
(98)
x 1 = y, x 2 = ˙ yd
(99)
ϕ 1 = − ˙ yd
(100)
ϕ 2 = c 1( z 2 − c 1 z 1) − ¨ yd
(101)
According to remark 5, it remains to define ¯
ϕ, Vz. From (81), definition (23) and n = 2, it
follows that
¯
ϕ = |γ 2[1] |, |γ 2[2] |, fd, |ϕ 2 + c 2 z 2 |
= [ | ˙ y|, |y|, fd, |ϕ 2 + c 2 z 2 |]
(102)
From (35) and n = 2 it follows that
Vz = (1/2)( z 2 +
)
1
z 22
(103)
94
16
Will-be-set-by-IN-TECH
Applications of Nonlinear Control
Expressions (97) to (103) allow to define the control and update law. From (32), (48), (82) and
n = 2 it follows that
sgn( b) = +1
(104)
u = − 1 z
3 C
2( ¯
ϕ ˆ θ)2
(105)
bvz
˙ˆ θ = Γ ¯ ϕ|z 2 | ∂ ¯ Vz
∂
(106)
Vz
the main parameters needed to compute u and ˆ θ are: ¯ ϕ (102), ϕ 2 (101), Cbvz (28), z 2 (98), z 1
(97), ∂ ¯
Vz/ ∂Vz (42), Vz (103). In addition, Γ is a diagonal matrix whose diagonal elements are
positive constants defined by the user.
3
2
d
y,y
1
0
0
20
40
60
80
100
time
0.1
e
0
−0.10
20
40
60
80
100
time
4
u 2
0
0
20
40
60
80
100
time
Fig. 2. Example 1, upper: output y (continuous line), desired output yd (dash–dot line);
middle: tracking error e; lower: control input u.
Since the aim is that y converges towards yd, with a threshold of 0.1, we set Cbe = 0.1.
We use the reference model (5) with yd( to) = y( to), ˙ yd( to) = 0, am,1 = 1, am, o = 1.
We use the following parameter values for the control and update laws: c 1 = 2, c 2 = 2,
Γ = diag { 1, 1, 1, 1 }.
The results are shown in figures 2 and 3. We have choosen yd( t 0) ≈ y( t 0) in order to obtain a
rapid convergence of y towards yd. Figure 2 shows that. i) the tracking error e converges
asymptotically towards Ω e = {e : |e| ≤ 0.1 }. ii) The output y converges towards yd with threshold 0.1 without large transient differences. Figure 3 shows that ˆ θ 1, ..., ˆ θ 4 are not
decreasing with respect to time. This occurs because ˙ˆ θ is non-negative. The procedure for the
sample plant (80) is simpler in comparison with adapive controllers that use the Nussbaum
gain method.
A Robust State Feedback Adaptive Controller
with Improved Transient Tracking Error Bounds for Plants with Unknown Varying Control Gain
95
A Robust State Feedback Adaptive Controller with Improved Transient Tracking Error Bounds for Plants with Unknown Varying Control Gain 17
0.1
^ θ 1 0.05
00
20
40
60
80
100
time
0.2
^ θ 2 0.1
00
20
40
60
80
100
time
0.2
^ θ 3 0.1
00
20
40
60
80
100
time
0.4
^ θ 4 0.2
00
20
40
60
80
100
time
Fig. 3. Example 1, entries of the updated parameter vector ˆ θ, from upper to lower: ˆ θ 1; ˆ θ 2, ˆ θ 3, ˆ θ 4.
9. Acknowledgements
A. Rincon acknowledges financial support provided by “Programa de becas para estudiantes
sobresalientes de posgrado”, Universidad Nacional de Colombia - vicerrectoría de
Investigación. This work was partially supported by Universidad Nacional de Colombia -
Manizales, project 12475, Vicerrectoría de Investigación, DIMA.
10. References
Bashash, S. & Jalili, N. (2009). Robust adaptive control of coupled parallel piezo–flexural
nanopositioning stages, IEEE/ASME Transactions on Mechatronics Vol. 14(No.
1): 11–20.
Bechlioulis, C. & Rovithakis, G. (2009). Adaptive control with guaranteed transient and
steady state tracking error bounds for strict feedback systems, Automatica Vol. 45(No.
2): 532–538.
Cao, C. & Hovakimyan, N. (2006). Design and analysis of a novel l 1 adaptive controller,
part i: control signal and asymptotic stability, Proceedings of the 2006 American Control
Conference, Publisher, Minneapolis (USA), pp. 3397–3402.
Cao, C. & Hovakimyan, N. (2008a).
Design and analysis of a novel adaptive control
architecture with guaranteed transient performance, IEEE Transactions on Automatic
Control Vol. 53(No. 2): 586–591.
Cao, C. & Hovakimyan, N. (2008b). l 1 adaptive output feedback controller for systems of
unknown dimension, IEEE Transactions on Automatic Control Vol. 53(No. 3): 815–821.
Chen, B., Liu, X., Liu, K. & Lin, C. (2009). Novel adaptive neural control design for nonlinear
mimo time–delay systems, Automatica Vol. 45(No. 6): 1554–1560.
96
18
Will-be-set-by-IN-TECH
Applications of Nonlinear Control
Chen, C., Lin, C. & Chen, T. (2008). Intelligent adaptive control for mimo uncertain nonlinear
systems, Expert Systems with Applications Vol. 35(No. 3): 865 – 877.
Chen, W. (2009). Adaptive backstepping dynamic surface control for systems with periodic
disturbances using neural networks, IET Control Theory and Applications Vol. 3(No.
10): 1383–1394.
Dobrokhodov, V., Kitsios, I., Kaminer, I. & Jones, K. (2008). Flight validation of a metrics driven
l 1 adaptive control, Proceedings of AIAA Guidance, Navigation and Control Conference
and Exhibit, Publisher, Honolulu (USA), pp. 1–22.
Du, H., Ge, S. & Liu, J. (2010). Adaptive neural network output feedback control for a class
of non–afine non–linear systems with unmodelled dynamics, IET Control Theory and
Applications Vol. 5(No. 3): 465–477.
Feng, Y., Hong, H., Chen, X. & Su, C. (2008).
Robust adaptive controller design for a
class of nonlinear systems preceded by generalized prandtl-ishlinskii hysteresis
representation, Proceedings of 7 th World Congress on Intelligent Control and Automation,
Publisher, Chongqing (China), pp. 382–387.
Feng, Y., Hu, Y. & Su, C. (2006). Robust adaptive control for a class of perturbed strict-feedback
nonlinear systems with unknown prandtl-ishlinskii hysteresis, Proceedings of the 2006
IEEE International Symposium on Intelligent Control, Publisher, Munich (Germany),
pp. 106–111.
Feng, Y., Su, C. & Hong, H. (2008).
Universal contruction of robust adaptive control
laws for a class of nonlinear systems preceded by generalized prandtl-ishlinskii
representation, Proceedings of the 3rd IEEE Conference on Industrial Electronics and
Applications, Publisher, Singapore, pp. 153–158.
Feng, Y., Su, C., Hong, H. & Ge, S. (2007). Robust adaptive control for a class of nonlinear
systems with generalized prandtl-ishlinskii hysteresis, Proceedings of the 46 th IEEE
Conference on Decision and Control, Publisher, New Orleans (LA, USA), pp. 4833–4838.
Ge, S. & Tee, K. (2007). Approximation–based control of nonlinear mimo time–delay systems,
Automatica Vol. 43(No. 1): 31–43.
Ge, S. & Wang, J. (2003). Robust adaptive tracking for time-varying uncertain nonlinear
systems with unknown control coefficients, IEEE Transactions on Automatic Control
Vol. 48(No. 8): 1463–1469.
Ho, H., Wong, Y. & Rad, A. (2009). Adaptive fuzzy sliding mode control with chattering
elimination for nonlinear siso systems, Simulation Modelling Practice and Theory Vol.
17(No. 7): 1199–1210.
Hong, Y. & Yao, B. (2007). A globally stable saturated desired compensation adaptive robust
control for linear motor systems with comparative experiments, Automatica Vol.
43(No. 10): 1840–1848.
Hsu, C., Lin, C. & Lee, T. (2006). Wavelet adaptive backstepping control for a class of nonlinear
systems, IEEE Transactions on Neural Networks Vol. 17(No. 5): 1175–1183.
Huang, A. & Kuo, Y. (2001). Sliding control of non-linear systems containing time-varying
uncertainties with unknown bounds, International Journal of Control Vol. 74(No.
3): 252–264.
Ioannou, P. & Sun, J. (1996). Robust Adaptive Control, Prentice-Hall PTR Upper Saddle River,
New Jersey.
A Robust State Feedback Adaptive Controller
with Improved Transient Tracking Error Bounds for Plants with Unknown Varying Control Gain
97
A Robust State Feedback Adaptive Controller with Improved Transient Tracking Error Bounds for Plants with Unknown Varying Control Gain 19
Jiang, Z. & Hill, D. (1999). A robust adaptive backstepping scheme for nonlinear systems with
unmodeled dynamics, IEEE Transactions on Automatic Control Vol. 44(No. 9): 1705–
1711.
Kanellakopoulos, I., Kokotović, P. & Morse, A. (1991).
Systematic design of adaptive
controllers for feeedback linearizable systems, IEEE Transactions on Automatic Control
Vol. 36(No. 11): 1241 – 1253.
Koo, K. (2001). Stable adaptive fuzzy controller with time-varying dead zone, Fuzzy Sets and
Systems Vol. 121(No. 1): 161–168.
Labiod, S. & Guerra, T. (2007). Adaptive fuzzy control of a class of siso nonaffine nonlinear
systems, Fuzzy Sets and Systems Vol. 158(No. 10): 1126 – 1137.
Li, D. & Hovakimyan, N. (2008). Filter design for feedback–loop trade–off of l 1 adaptive
controller:
a linear matrix inequality approach, Proceedings of IAA Guidance,
Navigation and Control Conference and Exhibit, Publisher, Honolulu (USA), pp. 1–12.
Li, Y., Qiang, S., Zhuang, X. & Kaynak, O. (2004). Robust and adaptive backstepping control
for nonlinear systems using rbf neural networks, IEEE Transactions on Neural Networks
Vol. 15(No. 3): 693–701.
Liu, C. & Tong, S. (2010).
Fuzzy adaptive decentralized control for nonlinear systems
with unknown high-frequency gain sign based on k–filter, Proceedings of Control and
Decision Conference, Publisher, China, pp. 1576–1581.
Nakanishi, J., Farrell, J. & Schaal, S. (2005). Composite adaptive control with locally weighted
statistical learning, Neural Networks Vol. 18(No. 1): 71–90.
Park, B., Yoo, S., Park, J. & Choi, Y. (2009).
Adaptive neural sliding mode control of
nonholonomic wheeled mobile robots with model uncertainty, IEEE Transactions on
Control Systems Technology Vol. 17(No. 1): 201–214.
Psillakis, H. (2010). Further results on the use of nussbaum gains in adaptive neural network
control, IEEE Transactions on Automatic Control Vol. 55(No. 12): 2841–2846.
Ren, B., Ge, S., Lee, T. & Su, C. (2008). Adaptive neural control for uncertain nonlinear systems
in pure-feedback form with hysteresis input, Proceedings of the 47th IEEE Conference
on Decision and Control, Publisher, Cancun (Mexico), pp. 86–91.
Royden, H. (1988). Real Analysis, Prentice Hall Upper Saddle River, New Jersey.
Slotine, J. & Li, W. (1991). Applied Nonlinear Control, Prentice Hall Englewood Cliffs, New
Jersey.
Su, C., Feng, Y., Hong, H. & Chen, X. (2009). Adaptive control of system involving complex
hysteretic nonlinearities:
a generalised prandtl-ishlinskii modelling approach,
International Journal of Control Vol. 82(No. 10): 1786–1793.
Tong, S., Liu, C. & Li, Y. (2010). Fuzzy–adaptive decentralized output–feedback control for
large–scale nonlinear systems with dynnamical uncertainties, IEEE Transaction on
Fuzzy Systems Vol. 18(No. 5): 1–1.
Tong, S., Tang, J. & Wang., T. (2000). Fuzzy adaptive control of multivariable nonlinear
systems, Fuzzy Sets and Systems Vol. 111(No. 2): 153–167.
Wang, X., Su, C. & Hong, H. (2004). Robust adaptive control of a class of nonlinear systems
with unknown dead-zone, Automatica Vol. 40(No. 3): 407–413.
Wen, C., Zhou, J. & Wang, W. (2009). Decentralized adaptive backst epping stabilization of
interconnected systems with dynamic input and output interactions, Automatica Vol.
45(No. 1): 55–67.
98
20
Will-be-set-by-IN-TECH
Applications of Nonlinear Control
Yao, B. (1997). High performance adaptive robust control of nonlinear systems: a general
framework and new schemes, Proceedings of the 36 th Conference on Decision and
Control, Publisher, San Diego (California, USA), pp. 2489–2494.
Yao, B. & Tomizuka, M. (1994).
Robust adaptive sliding mode control of manipulators
with guaranteed transient performance, Proceedings of the American Control Conference,
Publisher, Baltimore (Maryland, USA), pp. 1176–1180.
Yao, B. & Tomizuka, M. (1997).
Adaptive robust control of siso nonlinear systems in a
semi-strict feedback form, Automatica Vol. 33(No. 5): 893–900.
Yousef, H. & Wahba, M. (2009). Adaptive fuzzy mimo control of induction motors, Expert
Systems with Applications Vol. 36(No. 3): 4171–4175.
Zhang, T. & Ge, S. (2009). Adaptive neural network tracking control of mimo nonlinear
systems with unknown dead zones and control directions, IEEE Transactions on
Neural Networks Vol. 20(No. 3): 483–497.
Zhou, J., Wen, C. & Wang, W. (2009). Adaptive backstepping control of uncertain systems
with unknown input time–delay, Automatica Vol. 45(No. 6): 1415–1422.
Zhou, J., Wen, C. & Zhang, Y. (2004). Adaptive backstepping control of a class of uncertain
nonlinear systems with unknown backlash-like hysteresis, IEEE Transactions on
Automatic Control Vol. 49(No. 10): 1751–1757.
6
A Robust Motion Tracking Control
of Piezo-Positioning Mechanism
with Hysteresis Estimation
Amir Farrokh Payam,
Mohammad Javad Yazdanpanah and Morteza Fathipour
Department of Electrical and Computer Engineering, University of Tehran, Tehran,
Iran
1. Introduction
Piezoelectric actuators are the most suited actuation devices for high precision motion
operations in the positioning tasks include miro/nano-positioning [1]. These actuators have
unlimited motion resolution and posses some advantages such as ignorable friction,
noiseless, zero backlash and easy maintenance, in comparison with the conventional
actuated systems which are based on the sliding or revolute lower pairs [2].
Producing large forces, fast response and high efficiency are major advantages of
piezoelectric actuators. But, it has some drawbacks such as hysteresis behavior, drift in time,
temperature dependence and vibration effects. Molecular friction at sites of materials
imperfections due to domain walls motion is the general cause of hysteresis in piezoelectric
materials [3]. The hysteresis is a major nonlinearity for piezo-actuators and often limits
system performance via undesirable oscillations or instability. Therefore, it is difficult to
obtain an accurate trajectory tracking control. Numerous mathematical methods have been
proposed to analyze the hysteresis behavior of piezoelectric actuators. These studies may be
categorized in asymmetrical and symmetrical methods. The asymmetrical types of hysteretic
models include polynomial model [4], Preisach’s model [5], neural network model [6] and
Karasnoselskii and Pokrovskii [7]. The symmetrical types of hysteretic model include
Duhem model [8], Bouc-Wen model [9] and Lugre model [9].
The asymmetrical methods establish the nonlinear relations between the input and output
based on the measured input/output data sets. Because superposition of a basic hysteresis
operator is a fundamental principle in these models, they are also called to operator based
model. Although, an operator based model may give a good match with experimental data,
the dynamics of the piezoelectric material is not formulated in these modeling methods and
model parameter identification and implementation is more difficult in this case. The
symmetrical methods employ nonlinear differential equations in order to describe
hysteresis. In this case, the dynamics of the piezoelectric materials are described but the non-
symmetric hysteresis is not modeled. However these models are more tractable for control
design. In order to include the hysteresis effect and compensating its effect, Lugr