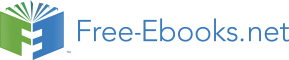

nonlinear robust synthetic controller can restrain the chaotic phenomenon of ship power
1.001
1
U1 0.999
0.998
0.1
1.02
0.2
1.01
0.3
1
0.4
0.99
0.5
0.98
ω1
δ1
Fig. 13. Phase diagram of No.1 diesel-generator set when two sets load 25% plus periodicity
load on parallel connection after using nonlinear robust synthetic controller
76
Applications of Nonlinear Control
1.001
1
U2 0.999
0.998
0.1
1.02
0.2
1.01
0.3
1
0.4
0.99
0.5
0.98
ω2
δ2
Fig. 14. Phase diagram of No.2 diesel-generator set when two sets load 25% plus periodicity
load on parallel connection after using nonlinear robust synthetic controller
system, making the nonlinear characteristic of ship power system offset and compensate,
thus improving the stability of ship power system.
Because ship power system made up of diesel-generator sets is a nonlinear system, using
nonlinear robust synthetic controller can offset and compensate the nonlinear characteristic
of ship power system, it can average the load in parallel operation control, there is no power
angular difference between the diesel-generator sets, thus avoiding the power transmission
between the sets, solving the problem of system chaotic oscillation fundamentally,
improving the voltage and frequency stability of ship power system. The research on
nonlinear robust synthetic controller of diesel-generator set provides a new control method
for ship power system, having important practical significance and extensive using
prospect.
6. Conclusion
In order to analyze the chaos phenomenon of ship power system, the nonlinear
mathematical model of two diesel-generator sets on parallel connection is built in this paper,
which reflects the relationship of interaction and mutual influence between two sets. The
light load working condition of two diesel-generator sets on parallel connection in ship
power station is analyzed by using Lyapunov index method, which proves the presence of
chaos phenomenon. A nonlinear robust synthetic controller is designed which is based on
the nonlinear mathematical model of diesel-generator set. In combining the direct feedback
linearization with robust control theory to design the synthetic controller for the diesel-
generator set, then a nonlinear robust synthetic control law is developed for the diesel-
generator set. The computer simulation results show that the nonlinear robust synthetic
Robust Control Research of Chaos
Phenomenon for Diesel-Generator Set on Parallel Connection
77
controller effectively suppresses the chaos phenomenon of ship power system, thus
providing desirable stability for ship power system.
7. References
[1] Huang Manlei, Tang Jiaheng, Guo Zhenming.The Mathematical Model of Diesel
Engine Speed Regulation System[J].Journal of Harbin Engineering University,
1997, 18(6):20~25
[2] Huang Manlei, Li Dianpu, Liu Hongda.Simulation Research on Double-pulse Speed
Governor of Diesel Engine[J].SHIP ENGINEERING, 2002, 24(3):36~38
[3] Huang Manlei, Wang Changhong.Nonlinear mathematical model of diesel-generator
set on ship[J].Journal of Harbin Engineering University, 2006, 27(1):15-19, 47.
[4] Yang Zhengling, Lin Kongyuan.Study on the relation between classical swing
equations and chaos[J].Automation of Electric Power Systems, 2000,
24(7):20~22,45
[5] Jia Hongjie,Yu Yixin,Wang Chengshan.Chaotic phenomena in power systems and its
studies [J].Proceedings of the CSEE, 2001, 21(7):26~30
[6] Wang Baohua, Yang Chengwu, Zhang Qiang. Summary of bifurcation and chaos
research in electric power system[J].Transactions of China Electrotechnical
Society, 2005, 20(7):1~10
[7] Bernstein D S, Hadded W M.LQG control with an H∞ performance bound: A Riccati
equation approach.IEEE Trans on Automatic Control, 1989, 34(3):293-305
[8] Zhou K, Glover K, Bodenheimer B, et al.Mixed H2 and H∞ performance objectives I:
Robust performance analysis.IEEE Trans on Automatic Control, 1994, 39(8):1564-
1574
[9] Doyle J, Zhou K, Glover K, et al.Mixed H2 and H∞ performance objectives II: Optimal
Control.IEEE Trans on Automatic Control, 1994, 39(8):1575-1587
[10] Khargonekar P P, Rotea M A.Mixed H2/H∞ control: A convex optimization
approach.IEEE Trans on Automatic Control, 1991, 36(7):824-837
[11] Sun Yu-song, Sun Yuan-zhang, Lu Qiang, Shao Yi-xiang.Research on Nonlinear
Robust Control Strategy for Hydroelectric Generator’s Valve[J].Proceedings of the
CSEE, 2001,21(2):56-59,65
[12] Li Wen-lei, Jing Yuan-wei, Liu Xiao-ping.Nonlinear robust control for turbine main
steam valve[J].Control Theory and Applications, 2003,20(3):387-390
[13] Wang Jin-hua.Design of mixed H2/H∞ controller[J].Control Theory and
Applications, 2004,21(1):45-53
[14] Robert Lashlee, Vittal Rao and Frank Kern.Mixed H2 and H∞ Optimal Control of Smart
Structures.Proceedings of the 33th conference on decision and control, Lake
Buena Vista, FL, December 1994:115-120
[15] Curtis P. Mracek and D. Brett Ridgely.Normal Acceleration Command Following of
the F-16 Using Optimal Control Methodologies: A Comparison
[16] Kap Rai Lee, Do Chang Oh, Kyeong Ho Bang and Hong Bae Park.Mixed H2/H∞
Control for Underwater Vehicle with Time Delay and Parameter
Uncertainty.Proceedings of the American Control Conference, Albuquerque,
New Mexico, June 1997:3225-3229
78
Applications of Nonlinear Control
[17] Kap Rai Lee, Do Chang Oh, Kyeong Ho Bang and Hong Bae Park.Mixed H2/H∞
Control with Regional Pole Placement for Underwater Vehicle
Systems.Proceedings of the American Control Conference, Chicago, Illinois, June
2000:80-84
[18] Feng Wu, Keat-Choon Goh and Steve Walsh.Robust H2 Performance Analysis for A
High-Purity Distillation Column.Computers Chem. Energy, 1997, 21:8161-8166
5
5
A Robust State Feedback Adaptive Controller
with Improved Transient Tracking Error Bounds
for Plants with Unknown Varying Control Gain
A. Rincon1, F. Angulo2 and G. Osorio2
1Universidad Católica de Manizales
2Universidad Nacional de Colombia - Sede Manizales - Facultad de Ingeniería y
Arquitectura - Departamento de Ingeniería Eléctrica, Electrónica y Computación -
Percepción y Control Inteligente - Bloque Q, Campus La Nubia, Manizales,
Colombia
1. Introduction
The design of robust model reference adaptive control (MRAC) schemes for plants in
controllable form, comprising unknown varying but bounded coefficients and varying control
gain has attracted a great deal of research. Many nonlinear systems may be described by the
controllable form; for instance, second order plants (see (Hong & Yao, 2007), (Hsu et al., 2006),
(Yao & Tomizuka, 1994), (Jiang & Hill, 1999)) and systems whose nonlinear behavior or part
of it, is represented by some function approximation technique (cf. Nakanishi et al. (2005),
(Chen et al., 2008), (Tong et al., 2000), (Huang & Kuo, 2001), (Yousef & Wahba, 2009), (Hsu
et al., 2006), (Koo, 2001), (Labiod & Guerra, 2007)).
The state adaptive backstepping (SAB) of (Kanellakopoulos et al., 1991) is a common
framework for the design of adaptive controllers for plants in controllable form. As is well
known, a major difficulty in introducing robustness techniques to SAB based schemes is that
the states zi and the stabilizing functions must be differentiable to certain extent (see (Yao &
Tomizuka, 1997), (Yao, 1997), (Ge & Wang, 2003)).
The robust SAB scheme of (Zhou et al., 2004), (Su et al., 2009), (Feng, Hong, Chen & Su,
2008) has the advantage that the knowledge on the upper or lower bounds of the plant
coefficients can be relaxed if the controller is properly designed and the control gain is constant
or known. The approach is based on the truncation method of (Slotine & Li, 1991), pp. 309.
The stabilizing functions are smoothed at each i-th step in order to render it differentiable
enough. The following benefits are obtained: i) the scheme is robust with respect to unknown
varying but bounded coefficients, ii) upper or lower bounds of the plant coefficients are not
required to be known, and iii) the tracking error converges to a residual set whose size is
user–defined.
The specific case of unknown varying control gain is an important issue, more difficult to
handle than other unknown varying coefficients. The varying control gain is usually handled
by means of robustness techniques (cf. (Wang et al., 2004), (Huang & Kuo, 2001), (Bechlioulis
80
2
Will-be-set-by-IN-TECH
Applications of Nonlinear Control
& Rovithakis, 2009), (Li et al., 2004)) or the Nussbaum gain technique (cf. (Su et al., 2009),
(Feng, Hong, Chen & Su, 2008), (Feng et al., 2006), (Ge & Wang, 2003)). The above methods
are applicable to plants in parametric–pure feedback or controllable form, and with controllers
that use the SAB or the MRAC as the control framework.
In (Wang et al., 2004), a system with dead zone in the actuator is considered, assuming that
both dead zone slopes have the same value. The input term is rewritten as the sum of an input
term with constant control gain plus a bounded disturbance-like term. The disturbance term
is rejected by means of a robust technique, based on (Slotine & Li, 1991) pp. 309. Nevertheless,
this strategy is not valid for different values of the slopes. Other robustness techniques
comprise a control law with compensating terms and either a projection modification of the
update law, as in cf. (Huang & Kuo, 2001), or a σ modification as in (Bechlioulis & Rovithakis,
2009), (Li et al., 2004). Nevertheless, some lower or upper bounds of the plant coefficients are
required to be known.
The Nussbaum gain technique can relax this requirement, as can be noticed from (Su et al.,
2009), (Feng, Hong, Chen & Su, 2008), (Feng, Su & Hong, 2008). The main drawbacks of
the Nussbaum gain method are (see (Su et al., 2009), (Feng, Hong, Chen & Su, 2008), (Feng
et al., 2006), (Ge & Wang, 2003), (Feng et al., 2007), (Feng, Su & Hong, 2008), (Ren et al.,
2008), (Zhang & Ge, 2009), (Du et al., 2010)): i) the upper bound of the transient behavior of
the tracking error is significantly modified in comparison with that of the disturbance-free
case: the value of this bound depends on the time integral of terms that comprise Nussbaum
terms, and ii) the controller involves an additional state, which is necessary to compute the
Nussbaum function.
Other drawbacks are: i) the control gain is assumed to be the product of a unknown constant
and a known function, as in (Tong et al., 2010), (Liu & Tong, 2010), ii) the control gain is
assumed upper bounded by some unknown constant, as in (Zhang & Ge, 2009), (Du et al.,
2010), (Su et al., 2009), (Feng, Hong, Chen & Su, 2008), (Feng et al., 2006), (Ge & Wang, 2003),
(Feng et al., 2007), (Feng, Su & Hong, 2008), (Ren et al., 2008), iii) the control gain is assumed
upper bounded by a known function, as in (Ge & Tee, 2007), (Psillakis, 2010), iv) upper
or lower bounds of the plant coefficients are required to be known to achieve asymptotic
convergence of the tracking error to a residual of user–defined size, as in (Ge & Tee, 2007),
(Chen et al., 2009), (Feng et al., 2006), (Ge & Wang, 2003), (Feng et al., 2007), (Ren et al., 2008),
(Ge & Tee, 2007), (Tong et al., 2010), (Liu & Tong, 2010), iv) the control or update law involves
signum type signals, as in (Zhang & Ge, 2009), (Du et al., 2010), (Psillakis, 2010), (Su et al.,
2009), (Feng, Hong, Chen & Su, 2008), (Feng, Su & Hong, 2008).
Recent adaptive control schemes based on the direct Lyapunov method achieve improved
transient performance. For instance, L 1 adaptive control, with the drawback that the control
gain is assumed constant, as in (Cao & Hovakimyan, 2006), (Cao & Hovakimyan, 2008a), (Cao
& Hovakimyan, 2008b), (Dobrokhodov et al., 2008), (Li & Hovakimyan, 2008).
Other works have the following drawbacks:
i) The control gain is assumed constant, as in (Zhou et al., 2009), (Wen et al., 2009), (Bashash &
Jalili, 2009).
ii) The control gain is assumed upper bounded by some unknown constant, as in (Chen, 2009),
(Ho et al., 2009) and (Park et al., 2009).
A Robust State Feedback Adaptive Controller
with Improved Transient Tracking Error Bounds for Plants with Unknown Varying Control Gain
81
A Robust State Feedback Adaptive Controller with Improved Transient Tracking Error Bounds for Plants with Unknown Varying Control Gain 3
iii) The control gain is assumed upper bounded by some known function as in (Bechlioulis &
Rovithakis, 2009).
iv) Upper or lower bounds of plant parameters are required to be known to achieve
asymptotic convergence of the tracking error to a residual set of user–defined size, as in
(Bashash & Jalili, 2009), (Chen, 2009), (Ho et al., 2009), (Park et al., 2009) and (Bechlioulis
& Rovithakis, 2009).
In this chapter, we develop a controller that overcomes the above drawbacks, so that:
Bi) The upper bound of tracking error transient value does not depend on time integral terms.
Bii) Additional states are not used in the controller.
Biii) The control gain is not required to be upper bounded by a constant.
Biv) The control gain is not required to be bounded by a known function.
Bv) Upper or lower bounds of the plant parameters are not required to be known.
Bvi) The control and update laws do not involve signum type signals.
Bvii) The tracking error converges to a residual set whose size is user–defined.
We consider systems described by the controllable form model with arbitrary relative degree,
unknown varying but bounded coefficients and varying control gain. We use the SAB of
(Kanellakopoulos et al., 1991) as a basic framework for the control design, preserving a simple
definition of the states resulting from the backstepping procedure. We use the Lyapunov–like
function method to handle the unknown time varying behavior of the plant parameters. All
closed loop signals remain bounded so that parameter drifting is prevented.
The key elements to handle the varying behavior of the control gain are: i) introduce the
control gain in the term involving the adjusted parameter vector, by means of the inequality
that relates the control gain and its lower bound, and ii) apply the Young’s inequality.
In current works that deal with plants in controllable form and time varying parameters and
use the state transformation based on the backstepping procedure, they modify the defined
states at each step of the state transformation in order to tackle the unknown time varying
behavior of the plant parameters. Instead of altering the state transformation, we formulate a
Lyapunov–like function, such that its magnitude and time derivative vanish when the states
resulting from the state transformation reach a target region.
The control design and proof of boundedness and convergence properties are simpler in
comparison to current works that use the Nussbaum gain method. The controller is also
simpler as it does not introduce additional states that would be necessary to handle the
unknown time varying control gain.
The chapter is organized as follows. In section 2 we detail the plant model. In section 3 we
present the goal of the control design. In section 4 we carry out a state transformation, based
on the state backstepping procedure. In section 5 we derive the control and update laws. In
section 6 we prove the boundedness of the closed loop signals. In section 7 we prove the
convergence of the tracking error e, finally, in section 8 we present an example.
82
4
Will-be-set-by-IN-TECH
Applications of Nonlinear Control
2. Problem statement
In this section we detail the plant and the reference model. Consider the following plant in
controllable form:
y( n) = γn a + bu + d
(1)
where y( t) ∈ R is the system output, u( t) ∈ R the input, a a vector of varying entries, γn a known vector, b the control gain, and d a disturbance-like term. We make the following
assumptions:
Ai) The vector a involves unknown, time varying, bounded entries a 1, · · · , aj, which satisfy:
|a 1 | ≤ ¯ μ 1, · · · , |aj| ≤ ¯ μj, where ¯ μ 1, · · · , ¯ μj are unknown, positive constants.
Aii) The entries of the vector γn are known linear or nonlinear functions of y, · · · , y( n− 1) .
Aiii) The terms y , ˙ y, · · · , y( n− 1) are available for measurement.
Aiv) The term d represents either external disturbances or unknown model terms that satisfy:
|d| ≤ μd fd,
(2)
where μd is an unknown positive constant, and fd is a known function that depends on y, · · · ,
y( n− 1). In the case that d is bounded, we have fd = 1. The term d may come from the product of a known function gd with an unknown varying but bounded coefficient cg: d = cggd,
|cg| ≤ μd, so that fd = |gd|, where μd is an unknown positive constant whereas gd is a known function.
Av) The control gain b satisfies:
|b| ≥ bm > 0, b = 0 ∀t ≥ to
(3)
where bm is an unknown lower bound, and the value of the signum of b is constant and
known.
Remark. We recall that μd, bm, ¯ μ 1, · · · , ¯ μj are unknown constants. In contrast, the values of y,
· · · , y( n− 1), γn, fd, sgn( b) are required to be known. Notice in assumption Av that we do not require the control gain b to be upper bounded by any constant. That is a major contribution
with respect to current works that use the Nussbaum gain method, e.g (Su et al., 2009), (Feng,
Hong, Chen & Su, 2008), (Feng, Su & Hong, 2008), (Feng et al., 2007), (Ge & Wang, 2003). The
requirement about the value of the signum of b is a common and acceptable requirement.
3. Control goal
Let
e( t) = y( t) − yd( t)
(4)
(
(
y n) + a
n− 1) + · · · + a
d
m, n− 1 yd
m, o yd = am, or
(5)
Ω e = {e : |e| ≤ Cbe}
(6)
A Robust State Feedback Adaptive Controller
with Improved Transient Tracking Error Bounds for Plants with Unknown Varying Control Gain
83
A Robust State Feedback Adaptive Controller with Improved Transient Tracking Error Bounds for Plants with Unknown Varying Control Gain 5
where e( t) is the tracking error, yd( t) is the desired output, Ω e is a residual set, r is the reference signal. Moreover, am, n− 1, · · · , am, o are constant coefficients defined by the user, such that the polynomial K( p) is Hurwitz, being K( p) defined as K( p) = p( n) + am, n− 1 p( n− 1) + · · · +
am, o. The reference signal r( t) is bounded and user–defined. The constant Cbe is positive and user–defined.
The objective of the MRAC design is to formulate a controller, provided by the plant model
(1) subject to assumptions Ai to Av, such that:
i) The tracking error e converges asymptotically to the residual set Ω e.
ii) The control signals are bounded and do not involve discontinuous signals.
4. State transformation based on the state backstepping
In this section we carry out a state transformation by following the steps 0, · · · , n of the
backstepping procedure. The plant model (1) can be rewritten as follows:
˙ xi = xi+1, 1 ≤ i ≤ n − 1
(7)
˙ xn = a γn( x 1, · · · , xn) + bu + d
(8)
x 1 = y, x 2 = ˙ y, · · · , xn = y( n− 1)
The model (7, 8) can be obtained by making γ 1 = · · · = γn− 1 = 0 in the parametric - pure
feedback form of (Kanellakopoulos et al., 1991). We use the SAB of (Kanellakopoulos et al.,
1991) as the basic framework for the formulation of the control and update laws.
We develop the SAB for the plant model (7, 8), and introduce a new robustness technique.
Since the order of the plant is n, the procedure comprises the steps 0, · · · , n, to be carried out
in a sequential manner.
Step 0. We begin by defining the state z 1 as the tracking error:
z 1 = e = y − yd = x 1 − yd
(9)
Step i (1 ≤ i ≤ n − 1). At each i-th step, we obtain the dynamics of the state zi by deriving it
with respect to time, and using the definitions of ˙ xi+1 provided by (7). For the sake of clarity,
we develop the step 1 and then we s