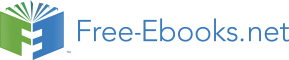

BIOTECHNOLOGY IN CHEMICAL
INDUSTRIES
The chemical industries have become a great threat in India. For the problems they cause on environmental pollution is much more than the benefit derived by their product. Some of them damage other living organisms like fishes, plants and animals; some cause health hazards to people living around the industries like respiratory ailments, skin problems and damage to nervous systems. So we have chosen to illustrate the minimization of pollution by CKD in cement Industries. Most of these problems can be controlled provided one takes the proper proportion of the mix of raw materials, which would minimize the pollution.
Cement kiln dust (CKD) emits nitrogen, carbon etc., that are pollutants of the atmosphere and the waste dust affects the smooth kiln operation of the cement industry system and it reduces the production of clinker quality. Hence the
minimization of waste CKD in kiln is an important one in the cement industry. The control of the waste CKD in a kiln is an uncertainty. Researchers approach this problem by
mathematical methods and try to account the waste CKD in a cement kiln. But, most of their methods do not properly yield results about the waste CKD in kiln. Further, the control of the waste CKD in kiln is a major problem for this alone can lead to the minimization of atmospheric pollution by the cement
11
industry. So in this chapter we minimize the waste CKD in kiln and account for the waste CKD in kiln using fuzzy control theory and fuzzy neural networks.
In this chapter fuzzy control theory (FCT) is used to study the cement kiln dust (CKD) problem in cement industries.
Using fuzzy control method this chapter tries to minimize the cement kiln dust in cement industries. Cement industries of our country happens to be one of the major contributors of dust. The dust arising in various processing units of a cement plant varies in composition. In 1990 the national average was 9 tons of CKD
generated for every 100 tons of clinker production. The control of cement kiln dust is a very important issue, because of the following reasons : 1. CKD emits nitrogen, carbon etc., which are pollutants of the atmosphere, 2. The waste dust affects the smooth kiln operation of the cement industry system and it reduces the production of clinker quality. The following creates mainly this waste dust in three ways in cement industries : (a) Cement kiln dust when not collected in time and returned into the kiln, cause air pollution, (b) Process instability and unscheduled kiln shutdowns and (c) Mixing of raw materials.
The data obtained from Graft R. Kessler [12] is used in this chapter to test the result. After using the data from Kessler [12]
this chapter tries to minimize the CKD in cement factory. The minimization of CKD plays a vital role in the control of pollution in the atmosphere.
W.Kreft [21] used the interruption of material cycles
method for taking account and further utilization of the waste dust in the cement factory. But this method does not properly account the waste CKD. Kesslar [12] has used volatile analysis to reduce CKD. In the volatile analysis method the alkali ratio is used to indicate the waste amount of CKD in clinker.
Kesslar [12] classifies the raw data under investigation in four ways :
I. Monitor and control of the system
II. Burning zone and fuel combustion improvements
III. CKD reprocessing
IV. Find the mix of raw materials in proper proportion.
The ratio of alkali should be lying between 0.5 to 1.5 in Kiln load material. But in this method the CKD was
12
approximately estimated up to 40%. He has not exactly
mentioned the percentage of CKD according to the alkali ratio in an online process. So this method has affected largely the kiln system.
In this chapter, in order to account for the waste CKD, the variables are expressed in terms of membership grades. This chapter considers all the four ways of waste CKD mentioned by Kesslar [12] and converts it into a fuzzy control model. This chapter consists of five sections. In section 1 we describe the cement kiln system and the nature of chemical waste dust which pollutes the atmosphere. In section 2 we adopt the fuzzy control theory to monitor and control the system and give suggestion for the improvement of burning and combustion zone. Section 3
deals with the determination of gas volume set point and temperature set point for CKD reprocessing which is vital for the determination of percentage of net CKD. The amount of waste dust depends largely on the mix of raw materials in proper proportion of raw material mix is shown in section 4.
The final section deals with results and conclusion obtained from our study.
2.1 Description of waste CKD in cement kiln
The data available from any cement industry is used as the information and also as the knowledge about the problem. This serves as the past experience for our study for adapting the fuzzy control theory in this section. This chapter analysis the data via membership functions of fuzzy control method and minimizes the waste CKD in cement industries. Since the
cement industry, emits the cement kiln dusts into the
atmosphere, this waste dust pollutes the atmosphere.
This analysis not only estimates the cement kiln dust in cement industries but also gives condition to minimize the waste CKD so that the industry will get maximum profit by minimizing the waste CKD in cement industry.
CKD is particulate matter that is collected from kiln exhaust gases and consist of entrained particles of clinker, raw materials and partially calcined raw materials. The present pollution in 13
environment is generated by CKD along with potential future liabilities of stored dust and this should make CKD reduction a high priority. Here we calculate and minimize the net CKD in kiln system. This chapter tackles the problem of minimizing waste CKD in kiln system in four stages. At the first stage we monitor and control the system. In the second stage we adopt time-to- time improved techniques in burning zone and
combustion. At the third stage CKD reprocessing is carried out and in the fourth stage we optimize the mix of raw materials in proper proportion using fuzzy neutral network. The above stage-by-stage process is shown in the following figure 2.1.1. Fuzzy control theory and fuzzy neutral network (FNN) is used in this chapter for the above – described method to minimize the CKD
in kiln system.
Step 1: Monitor
Step 4: Optimize
and control of the
and mix the raw
system
material in proper
proportion
CKD
Reduction
Final Step
Step 3: CKD
Step 2: Burning
Reprocessing
zone and
combustion
improvement
FIGURE 1: CKD Reduction using fuzzy control
The fuzzy controller is composed of linguistic control rule, which are conditional linguistic statements of the relationship between inputs and outputs. One of the attractive properties of fuzzy controller is its ability to emulate the behaviour of a human operator. Another important characteristic of a fuzzy controller is its applicability to systems with model uncertainty or even to unknown model systems. The use of fuzzy control 14
applications has expanded at an increasing rate in recent years.
In this chapter we use fuzzy control to monitor waste dust in cement kiln system and CKD reprocessing. The fuzzy control in kiln system is described in the figure 2.1.2. We use fuzzy neural network method and tries to find a proper proportion of material mix in cement industries.
The authors aim to achieve a desired level of lime saturation factor (LSF), silica modulus (SM) and alumina modulus (AM) of the raw mix, to produce a particular quality of the cement by controlling the mix proportions of the raw materials. To achieve an appropriate raw mix proportion is very difficult, due to the inconsistency in the chemical composition ratio given for the raw materials.
Fuzzy neural network model is used to obtain a desired
quality of clinker. The raw mix as per the norms of cement industries should maintain the ranges like LSF 1.02 to 1.08, SM
2.35 to 2.55 and AM 0.95 to 1.25, which are the key factors for the burnability of clinker to obtain a good quality of cement.
Fuzzy control theory method is used to minimize waste cement kiln dust. Fuzzy control theory allows varying degrees of set membership based on a membership function defined over a range of values. The membership function usually varies from 0
to 1.
Gross CKD
Gross CKD
Burning zone
Net
CKD
Dust
Fuzzy
Kiln
Collector
Control
Recycled CKD
Recycled CKD
Clinker
Raw materials
FIGURE 2: Fuzzy control in kiln system
15
2.2 Monitoring and control of the system using FCT
and improvement of burning zone and combustion
Monitoring and control of the system is the most effective method towards CKD reduction in environment. CKD consists mainly of raw materials, which contain volatile compounds, therefore, tracking and control of the volatile compounds throughout the system often allows for the minimal CKD. The initial step in our plan towards CKD reduction is to identify the amount of the CKD. Here the indirect weighing method is
applied to identify the amount of the CKD. Calculating
sulphur/alkali ratio is a good indication of a possible imbalance.
This ratio is calculated as the molar ratio of SO3/(K2O)+Na2O) in kiln load material.
CKD VOLATILE ANALYSIS
Volatile Molecular
Weight
Na2O 62
K2O 94.2
SO3 80
Ratio of alkali = SO3 /K2O + Na2O = 80/156.2 = 0.512
This ratio should be between the values 0.5 to 1.5 in Kiln load material. The industry knows upto 40% of CKD exits, when the alkali ratio is between the values 0.5 to 1.5. But they cannot say exactly how much percentage of CKD waste comes from kiln by using the ratio of alkali in the online process. If industry knows this correct percentage of CKD in the online process, they can change some condition in the kiln and thus reduce the CKD in the online process. We adopt fuzzy control to estimate the percentage of CKD by using the ratio of alkali.
The alkali ratio, kiln load material in tons and percentage of CKD are measured from the past happening process in kiln on a scale from 0.5 to 1.5, 5 to 25 tons and 0 to 40% respectively.
16
That is we assign the sulphur/alkali ratio shortly termed as alkali ratio, alkali ratio to be approximately low (L) when its value is 0.5, medium (M) when its value is 1 high (H) when its value is 1.5. In a similar way we give kiln load material ≅ {5 tons [first stage (FS)], 15 tons [second stage (SS)] and 25 tons [third stage (TS)]}. Percentage of CKD ≅ {0 [very less (VL)], 10 [less (L)], 20 [medium (M)], 30 [high (H)] and 40 [very high (VH)]}. (‘≅’
Denotes approximately equal). The terms of these parameters are presented in figures 2.2.1, 2.2.2 and 2.2.3.
L
M
H
Legend
MSG – Membership grade
1
L-low, M-medium, H-high
M
S
G
0.5
1
1.5
X Alkali ratio
FIGURE 2.2.1: Alkali ratio- input parameter
Legend
FS
SS
TS
MSG – Membership grade
FS- First stage, SS-Second
1
stage, TS- Third Stage
M
S
G
5 15 25
Y Kiln load material in tons
FIGURE 2.2.2: Kiln load material in tons-output parameter 17
VL
L
M
H
VH
1
M
S
G
0 10 20 30 40
Z Percentage of CKD
Legend
MSG – Membership grade
VL- very less, L-low, M-medium,
H-high, VH- very high
FIGURE 2.2.3: Percentage of CKD – output parameter
For the terms of alkali ratio, kiln load material in tons and percentage of CKD we give the following membership
functions:
μ
⎧ (X) = (1− X) 0.5 0.5 ≤ X ≤1
L
⎪
μ( )
⎪
⎧(X − 0.5) 0.5 0.5 ≤ X ≤1
X
= μ
⎨
(X) = ⎨
(2.2.1)
M
alkali ratio
⎪
⎩(1.5 − X) 0.5 1≤ X ≤1.5
⎪μ (X) = (X −1) 0.5 1≤ X ≤1.5
⎩ H
⎧ μ
Y = (15 − Y) 10 5 ≤ Y ≤ 15
FS (
)
⎪
μ( )
⎪
⎧ (Y − 5) 10 5 ≤ Y ≤15
Y
= μ
⎨
=
ki ln
Y
⎨
(2.2.2)
SS (
)
ratio in tons
⎪
⎩(25 − Y) 10 15 ≤ Y ≤ 25
⎪μ Y = (Y −15) 10 15 ≤ Y ≤ 25
⎩ TS ( )
18
⎧ μ
Z = (10 − Z) 10 0 ≤ Z ≤ 10
VL (
)
⎪
⎧ Z 10 0 ≤ Z ≤10
⎪ μ Z = ⎨
L (
)
⎪
⎩(20 − Z) 10 10 ≤ Z ≤ 20
⎪⎪
μ(
⎧ −
≤ ≤
Z)
(Z 10) 10 10
Z
20
= ⎨ μ
=
percentage
Z
⎨
(2.2.3)
M (
)
of CKD
⎪
⎩(30 − Z) 10 20 ≤ Z ≤ 30
⎪
⎧(Z − 20) 10 20 ≤ Z ≤ 30
⎪μ Z = ⎨
H (
)
⎪
⎩(40 − Z) 10 30 ≤ Z ≤ 40
μ
⎪
Z = (Z − 30) 10 30 ≤ Z ≤ 40
⎩ VH ( )
By applying the “if … and … then” rules [refer 11] to the three-membership functions μ(X), μ(Y) and μ(Z) we get the
following table of rules.
The rules given in Table 2.2.1 read as follows :
Table 2.2.1
Y FS SS
TS
X
L VL
M H
M L M H
H M H VH
For example :
If alkali ratio is L and kiln load material in tons is FS then percentage of CKD is VL. If alkali ratio is H and kiln load material in tons is TS then percentage of CKD is VH; and so on.
Rules of evaluation using the membership functions defined by the equation (2.2.1) and (2.2.2), if alkali ratio is 1.2 and kiln load material is 17 tons we get the fuzzy inputs as μM(1.2) = 0.6, μH(1.2) = 0.4, μSS(17) = 0.8 and μTS(17) = 0.2. Induced decision table for percentage of CKD is as follows.
19
Table
2.2.2
Y
0
μ
X
SS (17) = 0.8
μTS(17) = 0.2
0 0 0
0
μM(1.2)=0.6
0
μM(Z)
μH(Z)
μH(1.2)=0.4
0
μH(Z)
μVH(Z)
Conflict resolutions of the four rules is as follows:
Rule 1 : If X is M and Y is SS then Z is M
Rule 2 : If X is M and Y is TS then Z is H
Rule 3 : If X is H and Y is SS then Z is H
Rule 4 : If X is H and Y is TS then Z is VH
Now, using Table 2.2.2 we calculate the strength values of the four rules as 0.6, 0.2, 0.4 and 0.2. Control output for the percentage of CKD is given in table 2.2.3.
Table
2.2.3
Y
0 μ
X
SS (17) = 0.8
μTS(17) = 0.2
0 0
0
0
μM(1.2)=0.6 0 min{[0.6, μM(Z)]} min{[0.2,
μH(Z)]}
μH(1.2)=0.4 0 min{[0.4, μH(Z)]} min{[0.2,
μVH(Z)]}
To find the aggregate(agg) of the control outputs, we obtain the maximum of the minimum. This is given by the following figure 2.2.4, that is μagg (Z) = max {min {[0.6, μM(Z)] min {[0.4, μH(Z)],)], min [0.2, μvH(Z)]}. By applying the mean of
maximum method for defuzzification that is the intersection points of the line μ = 0.6 with the triangular fuzzy number μM(Z) in equation (2.2.3) we get the crisp output to be 20%.
20
VL
L
M
H
VH
1
M
S
G
0 10 20 30 40
Z Percentage of CKD
FIGURE 2.2.4: Aggregated output and defuzzificztion for
the percentage of CKD
Rules of evaluation using the membership function defined by the equation (1) and (2), if alkali ratio is 0.5 and kiln load material is 5 tons we get the fuzzy inputs as μL(0.5) = 1, μH(0.5)
= 0, μrs(5) = 1 and μss(5) = 0. Induced decision table for percentage of CKD is as follows.
Table 2.2.4
Y
μ
X
FS(5) = 1
μSS(5) = 0 0
μL(0.5) = 1 μVL(Z)
μM(Z )
0
μM(0.5 )= 0 μL(Z)
μM(Z )
0
0 0
0
0
Conflict resolutions of the four rules is as follows:
Rule 1 : If X is L and Y is FS then Z is VL
Rule 2 : If X is L and Y is SS then Z is M
Rule 3 : If X is M and Y is FS then Z is L
Rule 4 : If X is M and Y is SS then Z is M.
Now, using Table 2.2.4 we calculate the strength values of the four rules as 1, 0, 0 and 0. Control output for the percentage of CKD is given in Table 2.2.5.
21
Table
2.2.5
Y
μ
X
FS (5) = 1
μSS(5) = 0
0
μL (0.5) = 1 min {[1, μVL(Z)]} min
{[0,
μM(Z)]} 0
μH(0.5) = 0
min {[0, μL(Z)]} min
{[0,
μM(Z)]} 0
0 0
0
0
To find the aggregate of the control outputs, we obtain the maximum of the minimum. This is given by the following
figure 2.2.5 that is μagg (Z) = {min {l, μVL(Z)]}, min{[0, μM(Z)]}, min {[0, μL (Z)]}. By applying the mean of maximum method for defuzzification that is the intersection points of the line μ =1 with the triangular fuzzy number μVL(Z) in equation (3) and get the crisp output to be 0%.
VL
L
M
H
VH
1
M
S
G
0 10 20 30 40
Z Percentage of CKD
FIGURE 2.2.5: Aggregated output and defuzzificztion for
the percentage of CKD
Rules of evaluation using the membership function defined by the equations (1) and (2), if alkali ratio is 1 and kiln load material is 15 tons we get the fuzzy inputs as μL(1) = 0, μH (1) =
0 and μm (1) = 1, μFS (15) = 0, μSS(15) = 1, μTS (15) = 0, Induced decision table for percentage of CKD is as follows.
22
Table 2.2.6
Y μ
X
FS(15) = 0
μSS(15) = 1 μTS(15) = 0
μL(1) = 0
μVL(Z)
μM(Z )
μH(Z )
μM(1) = 1
μL(Z)
μM(Z )
μH(Z )
μH(1) = 0
μM(Z )
μH(Z )
μVH(Z )
Conflict resolutions of the nine rules is as follows :
Rule 1 : If X is L and Y is FS then Z is VL
Rule 2 : If X is L and Y is SS then Z is M
Rule 3 : If X is L and Y is TS then Z is H
Rule 4 : If X is M and Y is FS then Z is L.
Rule 5 : If X is M and Y is SS then Z is M.
Rule 6 : If X is M and Y is TS then Z is H.
Rule 7 : If X is H and Y is FS then Z is L.
Rule 8 : If X is H and Y is SS then Z is M.
Rule 9 : If X is H and Y is TS then Z is H.
Now, using Table 2.2.6 we calculate the strength values of the nine rules as 0, 0, 0, 0, 1, 0, 0, 0, 0. Control output for the percentage of CKD is given in Table 2.2.7.
Table 2.2.7
Y
μ
X
FS (15) = 0
μSS(15) = 1
μTS(15) = 1
μL (1)=0 min{[0,μVL(Z)]} min{[0,μM(Z)]} min{[0,μH(Z)]}
μM(1)=1 min{[0,μL(Z)]} min{[0,μM(Z)]} min{[0,μH(Z)]}
μH(1)=0 min{[0,μM(Z)]} min{[0,μH(Z)]} min{[0,μH(Z)]}
To find the aggregate of the control outputs, we obtain the maximum of the minimum. This is given by the following
figure 2.2.6, that is μagg (Z) = max {min {0, μVL(Z)]}, min{[0, μM(Z)]}, min {[0, μL(Z)]}, {min {l, μH(Z)]}, min{[0, μVH(Z)]}.
By applying the mean of maximum method for defuzzification that is the intersection points of the line μ =1 with the triangular 23
fuzzy number μVL(Z) in equation (2.2.3) and get the crisp output to 20%.
VL
L
M
H
VH
1
M
S
G
0 10 20 30 40
Z Percentage of CKD
FIGURE 2.2.6: Aggregated output and defuzzification for
the percentage of CKD
Rules of evaluation using the membership function defined by the equations (2.2.1) and (2.2.2), if alkali ratio is 1.5 and kiln load material is 25 tons we get the fuzzy inputs as μM (1.5) = 0, μH (1.5) = 1, μSS (25) = 0 and μTS (25) = 1. Induced decision table for percentage of CKD is as follows.
Table 2.2.8
Y
0
μ
X
SS(25) = 0
μTS(25) = 1
0 0
0
0
μM(1.5) = 0 0
μM(Z)
μH(Z )
μH(1.5) = 1 0
μH(Z)
μVH(Z )
Conflict resolutions of the four rules is as follows :
Rule 1 : If X is M and Y is SS then Z is M
Rule 2 : If X is M and Y is TS then Z is H