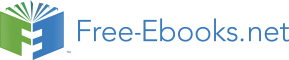

(15174
Y) 2154 13020 Y 15174
⎪ μ (Y) = (Y −13020) 2154 13020≤Y≤15174
⎩ TS
(2.3.2)
⎧ μ (Z) = (5 − Z) 5 0 ≤ Z ≤ 5
VL
⎪
⎧ Z 5 0 ≤ Z ≤ 5
⎪ μ (Z) = ⎨
L
⎪
⎩(10 − Z) 5 5 ≤ Z ≤10
⎪⎪
μ(
⎧
−
≤ ≤
Z)
(Z 5) 5 5
Z 10
= ⎨μ
=
percentage
(Z)
⎨
(2.3.3)
M
of net CKD
⎪
⎩(15 − Z) 5 10 ≤ Z ≤15
⎪
⎧(Z −10) 5 10 ≤ Z ≤15
⎪μ (Z) = ⎨
H
⎪
⎩(20 − Z) 5 15 ≤ Z ≤ 20
⎪ μ (Z) = (Z−15) 5 15≤ Z ≤ 20
⎩ VH
By applying the if … and … then rules to the three-membership function μ(X), μ(Y) and μ(Z), we get the following table of rules. The rules given in Table 2.3.1 read as follows:
Table 2.3.1
Y FS SS TS
X
L VL
M
H
M L M H
H M
H
VH
28
For example:
If temperature is L and gas volume is SS then percentage of net CKD is M.
If temperature is M and gas volume is TS then percentage of net CKD is H; and so on.
Rules of evaluation using the membership functions defined by the equation (2.3.1) and (2.3.2), if temperature is 430oC and gas volume is 13080 m3/min we get the fuzzy inputs as μM(430)
= 0.4, μH(430) = 0.6, μSS(13080) = 0.97 and μTS(3080) = 0.02.
Induced decision table for percentage of net CKD is as follows.
Table 2.3.2
Y
0 μ
X
SS(13080)= 0.97
μTS(13080)= 0.02
0 0 0
0
μM(430)=0.04 0
μM(Z)
μH(Z )
μH(430)=0.06 0
μH(Z)
μVH(Z )
Conflict resolutions of the four rules is as follows :
Rule 1 : If X is M and Y is SS then Z is M
Rule 2 : If X is M and Y is TS then Z is H
Rule 3 : If X is H and Y is SS then Z is H
Rule 4 : If X is H and Y is TS then Z is VH.
Now, using Table 2.3.2 we calculate the strength values of the four rules as 0.4, 0.02, 0.06 and 0.02. Control output for the percentage of net CKD is given in Table 2.3.3.
Table
2.3.3
Y
0
μ
X
SS (13080) = 0.97
μTS(13080) = 0.02
0 0
0
0
μM(430)=0.4 0 min {[0.4, μM(Z)]} min
{[0.02,
μH(Z)]}
μH(430)=0.6 0 min {[0.6, μH(Z)]} min{[0.02,
μVH(Z)]}
29
To find the aggregate of the control outputs, we obtain the maximum of the minimum. This is given by the following
figure 2.3.4, that is μagg (Z) = max{min [0.4, μM(Z)]}, min{[0.6, μM(Z)]}, min {[0.02, μVH (Z)]}. We apply the mean of
maximum method for defuzzification that is the intersection points of the line μ = 0.6 with the triangular fuzzy number μH(Z) in equation (2.3.3) and get the crisp output as 15 to 20%.
VL
L
M
H
VH
1
M
S
G
0
5
10
15
20 Z Percentage of CKD
FIGURE 2.3.4: Terms of the output and defuzzification for the percentage of net CKD
Rules of evaluation using the membership functions defined by the equation (2.3.1) and (2.3.2), if temperature is 350oC and gas volume is 11865 m3/min we get the fuzzy inputs as μL(350) = 1, μH(350) = 0, μFS(11865) = 1 and μSS(11865) = 0. Induced
decision table for percentage of net CKD is as follows.
Table
2.3.4
Y
μ
X
FS (11865) = 1
μSS(11865) = 0 0
μL(350) = 1
μVL(Z)
μM(Z)
0
μM(350) = 0
μL(Z)
μM(Z)
0
0 0 0
0
Conflict resolutions of the four rules is as follows :
Rule 1 : If X is L and Y is FS then Z is VL
30
Rule 2 : If X is L and Y is SS then Z is M
Rule 3 : If X is M and Y is FS then Z is L
Rule 4 : If X is M and Y is SS then Z is M.
Now, using Table 2.3.4 we calculate the strength values of the four rules as 1, 0, 0 and 0. Control output for the percentage of net CKD is given in table 2.3.5.
Table 2.3.5
Y
μ
X
FS (11865) = 1
μSS(11865 ) = 0 0
μL(350)=0.4 min
{[1,
μVL(Z)]} min {[0, μM(Z)]} 0
μM(350)= 0.6 min {[0, μL(Z)]} min
{[0,
μM(Z)]} 0
0 0 0
0
To find the aggregate of the control outputs, we obtain the maximum of the minimum.
This is given by the following figure that is μagg (Z) =
{min{[1, μVL (Z)]}, min{[0, μM (Z)]}, min {[0, μL (Z)]}. We apply the mean of maximum method for defuzzification that is the intersection points of the line μ = 1 with the triangular fuzzy number μVL(Z) in equation (2.3.3) and get the crisp output as 0 to 5%.
VL
L
M
H
VH
1
M
S
G
0
5 10 15 20
Z Percentage of CKD
FIGURE 2.3.5: Terms of the output and defuzzification for the percentage of net CKD
31
Rules of evaluation using the membership functions defined by the equation (4) and (5), if temperature is 400oC and gas volume is 13020 m3/min we get the fuzzy inputs as μL(400) = 0, μM(400) = 1, μH(400) = 0, μFS(13020) = 0, μSS(13020) = 1 and μTS(13020) = 0. Induced decision table 2.3.6 for percentage of net CKD is as follows.
Table 2.3.6
Y
μ
X
FS(13020)=0
μSS(13020)=1 μTS(13020)=0
μL(400)=0
μVL(Z)
μM(Z)
μH(Z )
μM(400)=1
μL(Z)
μM(Z)
μH(Z )
μH(400)=0
μM(Z)
μH(Z)
μVH(Z )
Conflict resolutions of the nine rules is as follows:
Rule 1 : If X is L and Y is FS then Z is VL
Rule 2 : If X is L and Y is SS then Z is M
Rule 3 : If X is L and Y is TS then Z is H
Rule 4 : If X is M and Y is FS then Z is L.
Rule 5 : If X is M and Y is SS then Z is M.
Rule 6 : If X is M and Y is TS then Z is H.
Rule 7 : If X is H and Y is FS then Z is M.
Rule 8 : If X is H and Y is SS then Z is H.
Rule 9 : If X is H and Y is TS then Z is VH.
Now, using Table 2.3.6 we calculate the strength values of the nine rules as 0, 0, 0, 0, 1, 0, 0, 0, 0. Control output for the percentage of net CKD is given in Table 2.3.7.
Table 2.3.7
Y μ
X
FS(13020) = 0
μSS(13020) = 1 μTS(13020) = 0
μL(400) =0 min{[1,μVL(Z)]} min{[0,μM(Z)]} min{[0,μH(Z)]}
μM(400)=1 min{[0, μL(Z)]} min{[1,μM(Z)]} min{[0,μH(Z)]}
μH(400)=0 min{[0, μM(Z)]} min{[0,μH(Z)]} min{[0,μvH(Z)]}
32
To find the aggregate(agg) of the control outputs, we obtain the maximum of the minimum.
This is given by the following figure 2.3.6, that is μagg (Z) =
max {min {[0, μVL(Z)]}, min {[1, μM(Z)],)], min {[0, μL(Z)]}, min {[0, μH(Z)]}, min {[0, μVH(Z)]}. We apply the mean of maximum method for defuzzification that is the intersection points of the line μ = 1 with the triangular fuzzy number μM(Z) in equation (2.3.3) we get the crisp output to be 10 % to 15 %.
VL
L
M
H
VH
1
M
S
G
0
5 10 15 20
Z Percentage of net
CKD
FIGURE 2.3.6: Aggregated output and defuzzification for
the percentage of net CKD
Rules of evaluation using the membership functions defined by the equation (2.3.1) and (2.3.2), if temperature is 450oC and gas volume is 15174 m3/min we get the fuzzy inputs as μM(450)
= 0, μH(450) = 1, μSS(15174) = 0 and μTS(15174) = 1. Induced decision table for percentage of net CKD is as follows.
Table
2.3.8
Y
0
μ
X
SS (15174) = 0
μTS(15174) = 1
0 0
0
0
μM(450) = 0 0
μM(Z)
μH(Z)
μH(450)= 1 0
μH(Z)
μVH(Z)
Conflict resolutions of the four rules is as follows:
33
Rule 1 : If X is M and Y is SS then Z is M
Rule 2 : If X is M and Y is TS then Z is H
Rule 3 : If X is H and Y is SS then Z is H
Rule 4 : If X is H and Y is TS then Z is VH.
Now, using Table 2.3.8 we calculate the strength values of the four rules as 0, 0, 0 and 1. Control output for the percentage of net CKD is given in Table 2.3.9.
Table 2.3.9
Y
0
μ
X
SS(15174) = 0
μTS(15174) = 0
0 0
0
0
μM(450)=0 0 min[0, μM(Z)] min[0,μH(Z)]
μH(400)= 1 0 min[0, μH(Z)] min[1,μVH(Z)]
To find the aggregate(agg) of the control outputs, we obtain the maximum of the minimum.
This is given by the following figure 2.3.7, that is μagg (Z) =
max{min{[0, μM(Z)]}, min{[0, μH(Z)],)], min{[1, μVH(Z)]}. We apply the mean of maximum method for defuzzification that is the intersection points of the line μ = 1 with the triangular fuzzy number μM(Z) in equation (2.3.3) we get the crisp output to be 20 %.
VL
L
M
H
VH
1
M
S
G
0
5 10 15 20
Z Percentage of net
CKD
FIGURE 2.3.7: Aggregated output and defuzzification for
the percentage of CKD
34
2.4 Finding the MIX of raw materials in proper proportion and minimize the waste dust using fuzzy neural network The study of proper proportions of material mix during the clinkerization process is very difficult due to inconsistency in the chemical and mineralogical composition and the variation of these characteristic affects kiln operation, fuel consumption, clinker quality and above all the amount of CKD vent into the atmosphere. Further the raw mix should maintain a fixed range for a specific quality of cement. The problem of satisfying this range involves lot of randomness and uncertainty, which in turn speaks about the desired quality of the clinker. Chemical and mineralogical composition contains SiO2, Al2O3, Fe2O3, CaO, MgO, K2O and Na2O. Since all terms used to determine the proper proportions of material mix is very ambiguous, we felt it would be proper to use fuzzy theory approach to study the problem. We adopt fuzzy relational neural network method to find the correct proportion of raw mix so that the desired quality of the clinker is achieved. This is done by taking experts opinion about the proportions and then by giving fuzzy weights.
This membership grades are varied a finite number of times till the error function reaches zero, which is equivalent to studying the set point values. The clinker of desired chemical
composition is expected to satisfy the following modulus related to the chemical composition of the raw mix.
Lime saturation factor (LSF),
CaO
× 100
LSF =
(2.4.1)
2.8 SiO + 1.2 Al O + 0.65Fe O
2
2
3
2
3
A high LSF requires high heat consumption for clinker
burning inside the kiln and this gives more strength to the cement.
Silica Modulus (SM)
SiO2
SM =
(2.4.2)
Al O + Fe O
2
3
2
3
35
A higher SM decreases the liquid phase content, which
impairs the burnability of the clinker and reduces the cement setting time.
Alumina Modulus (AM)
Al O
2
3
AM =
(2.4.3)
Fe O
2
3
The value of AM determines the composition of liquid phase in the clinker.
Here we describe the working of the block schematic of raw mill processing. The raw mill grinder receives raw materials such as limestone, silica, iron and bauxite for the production of cement in separate feeders, called weigh feeders. All the raw materials are ground in a raw mill grinder to a powder form. A sample of this ground raw mix is collected at the output of the raw mill grinder by an auto sampler, and a sample is prepared after being finely ground by vibration mill and pressed by hydraulic press and then is analysed in the laboratory by an X-ray sequential spectrometer. The results of X-ray analysis, which are obtained through sampling and analysing the
equipment, are fed to the computer through a data
communication line, for the required control action. The entire process is illustrated in figure 2.4.1.
Weigh
feeders
Auto
Sampler Storage &
Raw mill
Plant
Blending
Sequential
X-Ray
Sample
Spectrometer
Preparation
Computer
System
FIGURE 2.4.1 Block schematic of raw mill processing steps 36
The past researchers developed a control algorithm for raw mixing proportion based of singular value decomposition(SVD) methods. The purpose of this algorithm is to calculate the change in raw materials in each of the weigh feeders to achieve the raw mixing that is LSF, SM and AM.
Singular value decomposition(SVD) is one of the most
basic and important tools in the analysis and solution of the problems in numerical linear algebra, and are finding increasing applications in control and digital signal processing. The potential of SVD technique is first exploited in the domain of linear algebra, where it provides a reliable determination of the rank of the matrix, thereby leading to accurate solutions of linear equations.
Here we adopt raw mix proportion control algorithm to our problem. The purpose of this algorithm is to calculate the change in raw materials in each of the weigh feeders to achieve the target value of the chemical composition ratio (or) module of LSF, SM and AM.
Suppose at any instant the action of the control system gives rise to the composition change as dLSF', dSM' and dAM' in response to the required composition change as dLSF, dSM and dAM respectively then the total mean square error at that instant will be
E = (dLSF – dLSF')2+(dSM – dSM')2 + (dAM – dAM')2 (2.4.4) The problem now is to minimize E with respect to the change in the feeder content(dw;: i = 1, 2, …, n). Differentiating equation (2.4.4) with respect to dw and equating to zero, we will have dLSF' = dLSF, dSM' = dSM and dAM' = dAM. As mentioned
earlier, the values of LSF, SM and AM of the raw material, change constantly.
Our objective is to keep the values of LSF, SM and AM of the raw mix at the raw mill outlet fixed by changing the quantity of the raw material in the weigh feeders. So the module LSF, SM and AM are functions of the change in the raw material in different feeders. This can be represented as
37
n
LS
∂ F
dSLF′ = dLSF = ∑
dw
(2.4.5)
i
=
w
∂
i 1
i
n
SM
∂
dSM′ = dSM = ∑
dw
(2.4.6)
i
=
w
∂
i 1
i
n
AM
∂
dAM′ = dAM = ∑
dw
(2.4.7)
i
=
w
∂
i 1
i
n
∑dw = 0 (2.4.8)
i
i 1
=
LLi ≤ dwi ≤ HLi
(2.4.9)
where wi is the mix ratio of raw material in the feeder, LLi and HLi are the lower limit and the higher limit respectively of the raw material change possible for the ith feeder(i = 1, 2, …, n).
The composition change, for example in LSF is given by
dLSF'
=
LSFsp - LSFmeas
(2.4.10)
Here ‘sp’ stands for set point that is the desired value and ‘meas’ stands for the measured value that is the value achieved.
Now consider the solution of equation (2.3.5) to (2.3.8). The number of unknowns is the same as the number of weigh
feeders. If there are four unknowns then there are four weigh feeders, we have the following set of equations with four unknowns.
LS
∂ F
L
∂ SF
LS
∂ F
L
∂ SF
dw +
dw +
dw +
dw = dLSF′
1
2
3
4
w
∂
w
∂
w
∂
w
∂
1
2
3
4
(2.4.11)
38
SM
∂
SM
∂
SM
∂
SM
∂
dw +
dw +
dw +
dw = dSM′
1
2
3
4
w
∂
w
∂
w
∂
w
∂
1
2
3
4
(2.4.12)
A
∂ M
AM
∂
AM
∂
AM
∂
dw +
dw +
dw +
dw = dAM′
1
2
3
4
w
∂
w
∂
w
∂
w
∂
1
2
3
4
(2.4.13)
dw + dw + dw + dw = 0
(2.4.14)
1
2
3
4
Rearranging equations (2.4.11) to (2.4.14) in matrix form yields
⎡ LS
∂ F
L
∂ SF
LS
∂ F
L
∂ SF⎤
⎢
⎥
w
∂
w
∂
w
∂
w
∂
1
2
3
4
⎢
⎥ ⎡dw ⎤ ⎡dLS F′⎤
1
⎢ S
∂ M
SM
∂
SM
∂
SM
∂
⎥ ⎢
⎥ ⎢
⎥
dw
dS M′
⎢
⎥
2
w