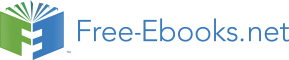

0
− 1
⎢0
⎥
⎢
⎥
−1
−1
−1 ⎢
q
− 10 ⎥
⎢1 − 10 − 19 q
1 + 3 9
. q ⎥
⎣
⎦⎢0
0
⎥
(9)
⎣
⎦
⎡ 1
0⎤~−1
• ⎢
F ( −1
q
[
~
) y ( t +
⎥
d) − H( −1
q ) y( t)
ref
]
− 1 1
⎣
⎦
−1
1
−
with specific forms of ~
F ( 1
−
q ) and ~
H ( 1
−
q ) not presented here due to their mathematical
complexity. Now, for y 1
= 1
ref
, y 2
= 5
.
1
ref
the outputs remain at the setpoint for t ≥ d = 2
under the stabilizing perfect control, whose plots u 1( t) , u 2( t) and u 3( t) according to equation (9) are shown in Fig. 1. For clarity, we have chosen to show the performance of
(noise-free) perfect control rather than MVC.
3
2.5
2
1.5
u (t)
3
1
0.5
u (t)
1
0
u (t)
2
-0.50
2
4
6
8
10
12
14
16
18
20
t
Fig. 1. Perfect control plots for the specific example
Remark. However, the Smith factorization approach undeniably contributes to the robust
MVC design, in the majority cases the application of the control zeros can give much better
results (Hunek, under review), giving rise to the extension of the Davison’s theory of
minimum phase systems (Davison, 1983). Unfortunately, there exists no formal proof of the
above statement and it has been left for future research.
6. Conclusions
The Smith factorization approach to the robust minimum variance control has been
presented in this paper. The new method appears much better than others, designed by
A Smith Factorization Approach to Robust Minimum Variance Control of
Nonsquare LTI MIMO Systems
379
authors, namely those based on the extreme points and extreme directions method and
second one called minimum-energy. Firstly, it is computationally much simpler and
secondly, it works also for the case when transmission zeros are (nongenerically) present in
the nonsquare system. Strange enough, the presented method should operate on the T -
inverse exclusively and any other inverse applied gives rise to the appearance of control
zeros. What is also strange, applying the T -inverse directly to the polynomial (.)
B (rather
than to its Smith-factorized form) inevitably ends up with control zeros. Finally, the new
approach confirms the Davison’s theory and indicates the need of the introduction of the
complementary control zeros theory.
7. References
Davison, E. J. (1983). Some properties of minimum phase systems and 'squared-down'
systems. IEEE Trans. Auto. Control, Vol. AC-28, No. 2, 1983, pp. 221-222.
Desoer, C. A. & Schulman, J. D. (1974). Zeros and poles of matrix transfer functions and
their dynamical interpretation. IEEE Trans. Circuits & Systems, Vol. CAS-21, No. 1,
1974, pp. 3-8.
Hunek, W. P. (2003). Control zeros for continuous-time LTI MIMO systems and their application
in theory of circuits and systems (in Polish), Ph.D. thesis, Opole University of
Technology, Department of Electrical, Control and Computer Engineering, Opole.
Hunek, W. P. (2007). A robust approach to the minimum variance control of LTI MIMO
systems. Emerging Technologies, Robotics and Control Systems, Vol. 2, 2007, pp. 133-
138, ISBN: 978-88-901928-9-5; also in International Journal of Factory Automation,
Robotics and Soft Computing, Vol. 2, 2007, pp. 191-196, ISSN: 1828-6984.
Hunek, W. P. (to be published). Towards robust minimum variance control of nonsquare
LTI MIMO systems. Archives of Control Sciences.
Hunek, W. P. & Latawiec, K. J. (2006). An inverse-free approach to minimum variance
control of LTI MIMO systems, Proceedings of 12th IEEE International Conference on
Methods and Models in Automation and Robotics (MMAR’2006), pp. 373-378,
Międzyzdroje, Poland, August 2006.
Hunek, W. P. & Latawiec, K. J. (under review). Minimum variance control of discrete-time
and continuous-time LTI MIMO systems - a new unified framework. Control and
Cybernetics.
Kaczorek, T. (1998). Vectors and Matrices in Automatic Control and Electrical Engineering (in
Polish), WNT, Warszawa.
Latawiec, K. J. (1998). Contributions to Advanced Control and Estimation for Linear Discrete-Time
MIMO Systems, Opole University of Technology Press, ISSN: 1429-6063, Opole.
Latawiec, K. J. (2004). The Power of Inverse Systems in Linear and Nonlinear Modeling and
Control, Opole University of Technology Press, ISSN: 1429-6063, Opole.
Latawiec, K. J.; Bańka, S. & Tokarzewski, J. (2000). Control zeros and nonminimum phase
LTI MIMO systems. Annual Reviews in Control, Vol. 24, 2000, pp. 105-112; also in
Proceedings of the IFAC World Congress, Vol. D, pp. 397-404, Beijing, P.R. China, 1999.
Latawiec, K. J. & Hunek, W. P. (2002). Control zeros for continuous-time LTI MIMO
systems, Proceedings of 8th IEEE International Conference on Methods and Models in
Automation and Robotics (MMAR’2002), pp. 411-416, Szczecin, Poland, September
2002.
380
New Approaches in Automation and Robotics
Latawiec, K. J.; Hunek, W. P. & Adamek, B. (2005a). A new uniform solution of the
minimum variance control problem for discrete-time and continuous-time LTI
MIMO systems, Proceedings of 11th IEEE International Conference on Methods and
Models in Automation and Robotics (MMAR’2005), pp. 339-344, Międzyzdroje,
Poland, August-September 2005.
Latawiec, K. J.; Hunek, W. P. & Łukaniszyn, M. (2004). A new type of control zeros for LTI
MIMO systems, Proceedings of 10th IEEE International Conference on Methods and
Models in Automation and Robotics (MMAR’2004), pp. 251-256, Międzyzdroje,
Poland, August-September 2004.
Latawiec, K. J.; Hunek, W. P. & Łukaniszyn, M. (2005b). New optimal solvers of MVC-
related linear matrix polynomial equations, Proceedings of 11th IEEE International
Conference on Methods and Models in Automation and Robotics (MMAR’2005), pp. 333-
338, Międzyzdroje, Poland, August-September 2005.
Latawiec, K. J.; Hunek, W. P.; Stanisławski, R. & Łukaniszyn, M. (2003). Control zeros versus
transmission zeros intriguingly revisited, Proceedings of 9th IEEE International
Conference on Methods and Models in Automation and Robotics (MMAR’2003), pp. 449-
454, Międzyzdroje, Poland, August 2003.
Rosenbrock, H. H. (1970). State-space and Multivariable Theory, Nelson-Wiley, New York.
Wolowich, W. A. (1974). Linear Multivariable Systems, Springer-Verlag, New York.
22
The Wafer Alignment Algorithm
Regardless of Rotational Center
HyungTae Kim, HaeJeong Yang and SungChul Kim
Korea Institute of Industrial Technology
South Korea
1. Introduction
Semiconductor manufacturers prefer automatic machines due to quality, productivity and
effectiveness. Compared with other industries, the semiconductor industry has automized
the individual steps of a process to a relatively high level. Most of the operators usually
learn about wafer placement rather than the principle of the process. But the manual
systems do not know the placement of a wafer, so operators should set the initial conditions
for the process. Wafer alignment is an operation for correcting the current wafer position in
the system coordinate until the wafer is located at the target position. The wafer position
varies after loading, so alignment steps are required.
Manual alignment systems need the operators’ help every time the wafer is loaded and is
actually a time-consuming process, that lowers manufacturing productivity and raises costs.
So, automatic alignment can save these time and costs. If the machines have automatic
alignment function, wafer processes can be connected automatically. Operators then would
only have to check and monitor the processing situation, and fix a problem when it arises.
Therefore, one operator can operate more machines, and would not be required to have high
process skills. Fig. 1 shows the concept of wafer alignment in the dicing process.
Passive alignment is related with mechanical structures which persist into external forces
without any actuators. A self-constrained mechanism is developed by Choi. When axial
force is exerted on a stage, grooves beneath the stage generate internal stress which prevents
from moving the stage (Choi et al., 1999). Pyramid and groove mechanism make stacking
force under external stress (Slocum & Weber, 2003).
Active alignment skill using sensors and actuators is applied widely in semiconductor
manufacturing process. Anderson’s method (Anderson et al, 2004) is based on cross-relation
between a defined template and an inspected image. He segmented the pixels around a
peak and interpolated under sub-pixel level. Misalignment can be detected by Moire effect
and laser beam. PZT actuators are applied to remove misalignment in lithography (Fan et
al., 2006). Machine vision is a common device to detect misalignment in wafer aligment
(Hong & Fang, 2002).
We have developed an algorithm for wafer alignment. This alignment algorithm was
derived from rigid body transformation or object transformation. The algorithm was based
on the simultaneous motion of x-y-θ axes in 2D space. A 2-step algorithm has a simple
form which can be written by 2×2 matrix operations. The magnitude of misalignment was
382
Desktop\New Approaches in Automation and Robotics
reduced in base of macro and micro inspection data(Kim et al., 2004). The matrix was
expanded with 4×4, and θ was included in the equation. So, the alignment variables of x-y-
θcan be calculated within one equation(Kim et al, 2004), (Kim et al, 2006). The
manufacturing condition which was varied to be ideal conditions, affected the quality of
alignment. Sometimes the misalignment did not become zero after only macro-micro
alignment. In this case, the problem can be solved by iterative alignment equation, which is
similar to numerical algorithms(Kim et al, 2007). The convergence speed of the iteration can
be controlled by the convergence constant. This constant can prevent numerical vibration.
The convergence analysis method, similar to a numerical method, is proposed(Kim et al,
2006). We tried to obtain the exact solution in these studies, but they found that the solutions
can be obtained from an estimated equation. The idea in this study came from the estimated
method. The equation could be simplified by making a few assumptions of the wafer
alignment condition. The derived formula did not have the terms for rotational center,
which was impossible to measure exactly. The alignment results showed that the
performance of the proposed algorithm was similar to the exact solution, and that the error
convergence speed can be controlled.
Fig. 1. Concept of wafer alignment in dicing process
2. Review of 2D alignment model
2.1 Coordinate transformation for alignment(Kim et al, 2004)
The machine coordinate in the alignment system has three variables - x, y and θ. Let be the
P(x,y) original coordinate, and P’(x’,y’) be the transformed coordinate. The each coordinate
can be shown as follows
T
T
T
P = ( x y θ )
1
P′ = ( x′ y′ θ ′ )
1
P = C
(
C 0 0)
x
y
(1)
The Wafer Alignment Algorithm Regardless of Rotational Center
383
The alignment space is on 2D, the alignment procedure carries out translation and rotational
motion. The movement can be described by rigid body transformation and the coordinate
after the motion can be calculated simply by multiplying matrices. The notation of the
translational matrix usually has a ‘T’, the rotational matrix has an ‘R’ and the center of
rotation has a ‘C’. Then the transformation is formulated by equation (2).
P′ = T{ R( P − C) + C}
(2)
TR matrices in the wafer alignment system can be derived as equations (3) and (4), which
are 4 × 4 matrices.
⎡1 0 0 Δ x⎤
⎢
⎥
0 1 0 Δ y
⎢
⎥
T = ⎢
(3)
0 0 1 0 ⎥
⎢
⎥
⎣0 0 0 1 ⎦
⎡cos Δθ − sin Δθ 0 0 ⎤
⎢
⎥
sin Δθ cos Δθ 0 0
⎢
⎥
R = ⎢
(4)
0
0
1 Δθ ⎥
⎢
⎥
⎣ 0
0
0 1 ⎦
2.2 Basic alignment algorithm(Kim et al, 2006)
The wafer has marks for alignment. The ideal mark position Pt is stored when the wafer is
perfectly aligned. Misalignment is calculated from the current position Pc. The vision system
inspects the location of the mark on the screen. These mark positions can be defined as
follows.
T
T
P = ( x y θ
)
1
P = ( x y θ
)
1
t
t
t
t
c
c
c
c
(5)
When the current position of the mark is deviated from the ideal one, the resulting
displacement can be defined as 4. The mark position in the machine can be obtained from
the target position and the displacement by equation (6).
P ≈ P + Δ = (x + Δx, y + Δy, θ + Δθ, 1 T
)
(6)
c
t
c
c
c
If the current position is compensated with an arbitrary value α = (α x, α y, α , 0), the mark
θ
will be located at the target position. So, an alignment algorithm f(x) can be written by
equation (7).
f(P ,α) − P = 0
c
t
(7)
f(Pc,α) can be replaced with the equation from the rigid body transformation. The result is
shown as (8). The T and R have the unknown compensation variable for the current
position.
384
Desktop\New Approaches in Automation and Robotics
T{R(P − C) + C} − P = 0
c
t
(8)
The plus direction between the mathematical coordinate and vision can be reverse, and the
relation can be written by vision direction matrix Dv whose diagonal terms have a value of
either +1 or -1 and the other terms are zero.
T{R(P + D Δ − C) + C} − P = 0
t
v
t
(9)
The direction problem can occur between the math coordinate and the machine, and the
machine direction matrix Dm has the similar characteristics as Dv.
T
β = D α β = (β β β
)
1
m
x
y
θ
(10)
The unknown α can be calculated from the equation, and (11) and (12) are the exact solution
in the case when two points are inspected to align a line.
α = x -C -(x -C ) cos θ + (y -C ) sin θ
xn
tn
x
cn
x
n
cn
y
n
α
(11)
= y -C -(x -C ) sin θ -(y -C ) cos θ
yn
tn
y
cn
x
n
cn
y
n
(x − x )(y − y ) − (y − y )(x − x )
tan α
c 1
c 2
t 1
t 2
c 1
c 2
t 1
t 2
=
θ
(12)
(x − x )(x − x ) + (y − y )(y − y )
t 1
t 2
c 1
c 2
t 1
t 2
c 1
c 2
3. Centerless model
3.1 Simplification
The equations (11) and (12) are the exact solution, but estimated solutions have been used
for many numerical problems. Some variables can be erased by geometric relations and
alignment conditions. Fig. 2 shows the general condition for wafer alignment in the dicing
process. First, angular misalignment in the process is within ±2o, which means sin α ≈ α and
cos α ≈ 1. the equation (11) can be written as follows
α = x -x + (y -C ) sin α = − Δ + (y -C )α
xn
tn
cn
cn
y
θn
xn
cn
y
θn
(13)
α = y -y -(x -C ) sin α = − Δ − (x -C )α
yn
tn
cn
cn
x
θn
yn
cn
x
θn
Second, inspection is carried out at two points, and the compensation value is the average,
α=(α1+α2)/2. And the inspection points are axis-symmetric at the rotational center,
2Cx−(xc1+xc2)=0. Another assumption is that the mark position is defined near the rotational
center, 2Cy−(yc1+yc2) = 0. Therefore, the equation (13) can be expressed as (14).
α = (
− Δ +