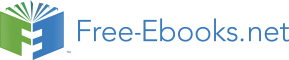

Strategy Analytics
Prof. Simon Watson is exploring a set of intelligent strategic moves for the innovation of emerging digital technologies such as scope analysis, requirements engineering, system design, talent management, team management and coordination, resources planning, concept development, concept evaluation, system architecture design, system development plan, roll out, process design, prototyping and testing The technological innovation on digital technology is associated with. Efficient change management ensures that an organization and its workforce are ready, willing and able to embrace the new processes and information systems. The change management is a complex process. The change should occur at various levels such as system, process, people and organization. Communication is the oil that ensures that everything works properly. It is essential to communicate the scope of digital technology to the policy makers, state and central governments, corporate executives, academic and research community.
Strategy can be analyzed from different dimensions such as R&D policy, learning curve, SWOT analysis, technology life-cycle analysis and knowledge management strategy. Technology trajectory is the path that the technology takes through its time and life-cycle from the perspectives of rate of performance improvement, rate of diffusion or rate of adoption in the market. The emerging digital technologies are now passing through the growth phase of S-curve. Initially, it may be difficult and costly to improve the performance of the technology. The performance is expected to improve with better understanding of the fundamental principles and system architecture. The dominant design should consider an optimal set of most advanced technological features which can meet the demand of the customer, supply and design chain in the best possible way. It is really interesting to analyze the impact of various factors on the trajectory of digital technology
Figure 10.15: Technology life-cycle analysis
SWOT Analysis on Cloud Computing : Let us exercise SWOT analysis on cloud computing. The strength of cloud computing is associated with several issues such as IT cost reduction, improved system performance and productivity, easier maintenance, agility, device and location independent access, scalability and elasticity via dynamic provisioning of resources in near real-time, security, multitenancy, availability, business continuity, disaster recovery, on demand self service (e.g. computing capabilities, server time, network storage capacity), broad network access by various client platforms (e.g., mobile phones, tablets, laptops, and workstations), resource pooling, rapid elasticity and measured services. Multitenancy enables sharing of resources and costs across a large pool of service consumers; the computing resources of the service provider can be pooled to serve multiple consumers using a multi-tenant model with different physical and virtual resources dynamically assigned and reassigned as per the demand of the service consumers. System capabilities can be elastically provisioned and released maintaining scalability automatically. Resource usage (e.g. storage, processing, bandwidth, active user accounts) can be monitored, controlled, optimized and reported with transparency. It is possible to ensure agility of an enterprise with re- provisioning, adding or expanding technological infrastructure resources. Cost reduction is possible by converting capital expenditure to operational expenditure. This strategy may lower barriers to entry as IT infrastructure is provided by a third party and need not be purchased for one time or infrequent intensive computing tasks.
But, there are several limitations of cloud computing such as privacy of critical strategic data, loss of control over critical strategic data, access control problems, confidentiality, data integrity, reliability, consistency, resiliency, network traffic congestion, delay in data communication, threats of various types of malicious attacks (e.g. sybil attack, false data injection attack, denial of service attack) and multi-party corruption. The top three threats in the cloud are insecure interfaces and APIs, data loss, data leakage and hardware failure. Cloud computing poses privacy concerns as the service provider can access the data of service consumer in the cloud at any time. It could accidentally or deliberately alter or delete critical data. The service providers may share or sell strategic data of the consumers to third parties. The consumers can encrypt data to prevent unauthorized access. But, this move may result high cost of computation and communication.
Figure10.16: Technology life–cycle analysis of solar computing
The technological innovation on a smart grid is associated with a set of intelligent strategic moves such as scope analysis, requirements engineering, quality control, system design, concurrent engineering, talent management, team management and coordination, resources planning, concept development, concept evaluation, system architecture design, system development plan, roll out, process design, prototyping and testing. Efficient change management ensures that an organization and its workforce are ready, willing and able to embrace the new processes and systems associated with a smart grid. The change management is a complex process. The change should occur at various levels such as system, process, people and organization. Communication is the oil that ensures everything works properly. It is essential to communicate the scope of solar grid and related solar computing to the public policy makers, state and central governments, corporate executives, academic and research community.
The fifth element of the deep analytics is strategy. This element can be analyzed from different dimensions such as R&D policy, learning curve, SWOT analysis, technology life-cycle analysis and knowledge management strategy. Technology trajectory is the path that the technology takes through its time and life-cycle from the perspectives of rate of performance improvement, rate of diffusion or rate of adoption in the market. The technology of solar computing is now passing through the emergence phase of S-curve. Initially, it may be difficult and costly to improve the performance of the technology. The performance is expected to improve with better understanding of the fundamental principles and system architecture. The dominant design should consider an optimal set of most advanced technological features which can meet the demand of the consumers in the best possible way. It is really interesting to analyze the impact of various factors on the trajectory of smart solar grid technology.
Figure 10.17 : Technology life-cycle analysis of SCADA & ICS
The fifth element of deep analytics is strategy. This element can be analyzed from different dimensions such as R&D policy, learning curve, SWOT analysis, technology life-cycle analysis and knowledge management strategy. Technology trajectory is the path that IIoT enabled ICS and SCADA technology takes through its time and life-cycle from the perspectives of rate of performance improvement, rate of diffusion or rate of adoption in the market. This technology is now passing through the growth phase of S-curve. Initially, it is difficult and costly to improve the performance of the technology. The performance is expected to improve with better understanding of the fundamental principles and system architecture. The dominant design should consider an optimal set of most advanced technological features which can meet the demand of the customer, supply and design chain in the best possible way. It is really interesting to analyze the impact of various factors on the trajectory IIOT enabled SCADA and ICS technology.
Strategy 1 : SIVM verifies innate and adaptive system immunity in terms of collective, machine, security, collaborative and business intelligence through multi-dimensional view on intelligent reasoning.
SIVM is defined by a set of elements : system, a group of agents, a finite set of inputs, a finite set of outcomes as defined by output function, a set of objective functions and constraints, payment function, an optimal set of moves, revelation principle and model checking or system verification protocol. The proposed mechanism evaluates the innate and adaptive immunity of a system which is defined by a set of states (e.g. initial, goal, local and global) and state transition relations.
The mechanism follows a set of strategic moves. The basic building block of the mechanism is an analytics having multidimensional view of intelligent reasoning. Reasoning has multiple dimensions like common sense, automated theorem proving, planning, understanding, hypothetical, simulation dynamics and envisioning i.e. imagination or anticipation of alternatives. The inference engine selects appropriate reasoning techniques from a list of options such as logical, analytical, case based, forward and backward chaining, sequential, parallel, uncertainty, probabilistic, approximation, predictive, imaginative and perception based reasoning depending on the demand of an application. Another important move is the collection of evidence through private search which may require a balance between breadth and depth optimally.
The critical challenge is how to detect the danger signal from a system? The mechanism evaluates system immunity (i) combinatorially in terms of collective, machine intelligence, security, collaborative and business intelligence. The collective intelligence (a) is defined in terms of scope, input, output, process, agents and system dynamics. For a complex application, it verifies coordination and integration among system, strategy, structure, staff, style, skill and shared vision. What is being done by the various components of a system? Who is doing? Why? How? Where? When? The machine intelligence (b) checks the system in terms of safety, liveness, concurrency, reachability, deadlock freeness, scalability and accuracy. For example, it should check preconditions, post conditions, triggering events, main flow, sub flow, alternate flow, exception flow, computational intelligence, communication cost, traffic congestion, time and space complexity, resources, capacity utilization, load, initial and goal states, local and global states and state transition plans of the information system associated with ICS/SCADA.
The security intelligence (c) verifies the system in terms of authentication, authorization, correct identification, non-repudiation, integrity, audit and privacy; rationality, fairness, correctness, resiliency, adaptation, transparency, accountability, trust, commitment, reliability and consistency. The collaborative intelligence (d) evaluates the feasibility and effectiveness of human-computer interaction to achieve single or multiple set of goals, information sharing principle and negotiation protocol. The business intelligence (e) is associated with business rules such as HR policy, payment function, cost sharing, bonus, contractual clauses, quality, performance, productivity, incentive policy and competitive intelligence.
The mechanism verifies system immunity through a set of verification algorithms. It is possible to follow various strategies like model checking, simulation, testing and deductive reasoning for automated verification. Simulation is done on the model while testing is performed on the actual product. It checks the correctness of output for a given input. Deductive reasoning tries to check the correctness of a system using axioms and proof rules. There is risk of state space explosion problem in case of a complex system with many components interacting with one another; it may be hard to evaluate the efficiency of coordination and integration appropriately. Some applications also demand semi-automated and natural verification protocol. The mechanism calls threat analytics and assesses risks of single or multiple attacks on the system under consideration: analyze performance, sensitivity, trends, exception and alerts; checks what is corrupted or compromised: agents, protocol, communication, data, application and computing schema? Performs time series analysis: what occurred? what is occuring? what will occur? assess probability of occurrence and impact; explores insights : how and why did it occur? do cause- effect analysis; recommends : what is the next best action? predicts: what is the best or worst that can happen?
Strategy 2 : SIVM verifies security intelligence collectively through rational threat analytics.
Model checking is an automated technique for verifying a finite state concurrent system such as a hardware or software system. Model checking has three steps: represent a system by automata, represent the property of a system by logic and design model checking algorithm. The security intelligence of SCADA is a multi- dimensional parameter which is defined at five different levels L1,L2,L3,L4 and L5. At level L1, it is essential to verify the security of data schema in terms of authentication, authorization, correct identification, privacy and audit of access control mechanism offered by the system. In this case, the system should ask the identity and authentication of one or more agents involved in SCADA operation and system administration. The agents of the same trust zone may skip authentication but it is essential for all sensitive communication across different trust boundaries. After correct identification and authentication, SIVM should address the issue of authorization. The system should be configured in such a way that an unauthorized agent cannot perform any task out of scope. The system should ask the credentials of the requester; validate the credentials and authorize the agents to perform a specific task as per agreed protocol. Each agent should be assigned an explicit set of access rights according to role. Privacy is another important issue; an agent can view only the information according to authorized access rights. A protocol preserves privacy if no agent learns anything more than its output; the only information that should be disclosed about other agent’s inputs is what can be derived from the output itself. The privacy of data may be preserved in different ways such as adding random noise to data, splitting a message into multiple parts randomly and sending each part to an agent through a number of parties hiding the identity of the source, controlling the sequence of passing selected messages from an agent to others through serial or parallel mode of communication, dynamically modifying the sequence of events and agents through random selection and permuting the sequence of messages randomly. The agents must commit the confidentiality of data exchange associated with private communication.
At level L2, it is essential to verify the computing schema in terms of fairness, correctness, transparency, accountability and trust. A protocol ensures correctness if the sending agent broadcasts correct data free from any false data injection attack and each recipient receives the same correct data in time without any change and modification done by any malicious agent. Fairness is associated with proper resource allocation, trust, commitment, honesty and rational reasoning of the agents involved in SCADA system administration. Fairness ensures that something will or will not occur infinitely often under certain conditions. The mechanism must ensure the accountability and responsibility of the agents in access control, data integrity and non-repudiation. The transparency of SCADA system administration is associated with communication protocols and revelation principle.
At level L3, it is essential to verify the application schema in terms of system performance. The performance of the system and quality of service is expected to be consistent and reliable. Reachability ensures that some particular state or situation can be reached. Safety indicates that under certain conditions, an event never occurs. Liveness ensures that under certain conditions an event will ultimately occur. Deadlock freeness indicates that a system can never be in a state in which no progress is possible; this indicates the correctness of a real-time dynamic system. SCADA is expected to be a resilient system. The resiliency measures the ability to and the speed at which the system can return to normal performance level following a disruption.
At level L4, SIVM verifies networking schema and assesses the threats of internal and external malicious attacks on SCADA & ICS such as cyber attack, rubber hose, sybil, node replication, wormhole, coremelt, forward, blackhole, neighbor, jellyfish and crypto jacking attack. At level L5, SIVM verifies security schema and assesses the risk of multi-party corruption (e.g. sender, receiver, data, communication channel, mechanism and protocol) and business intelligence of payment function.
Strategy 3 : The security intelligence of an industrial control system is verified comprehensively in terms of correctness of computing schema, stability and robustness in system performance of the plant, data, networking, security and application schema. Please refer to second test case in the appendix where SIVM is applied for a plant having supervisory adaptive fuzzy control. Let us first explain various types of circuits for intelligent process control of the plant. Next, it is essential to call threat analytics and verify the security intelligence of data, computing, application, networking and security schema at levels 1,2,3,4 and 5. At level L1, it is crucial to verify the data schema in terms of authentication, authorization, correct identification, privacy, audit, confidentiality, integrity, non-repudiation, locking of passwords, false data injection attack and intrusion for proper access control of the plant. Any flaws in access control may affect the stable performance of the plant negatively. A malicious agent can take over the control of the plant completely or partially by hacking system control passwords or compromising system administration.
Next, let us consider level L2 to verify the computing schema. Fuzzy logic is a multi- valued logic used for approximate reasoning. Fuzzy logic based algorithms are used for the control of complex plants through fuzzy controllers. The fuzzy controllers (FC) operate based on IF-THEN fuzzy rules. Industrial process control systems use various types of controllers such as P/PI/PID through adaptive gain scheduling, supervisory control architectures and algorithms. The fuzzy controllers use IF- THEN rules, can learn universal approximation property and can deal with fuzzy values. If there are flaws in design of these basic features of fuzzy controllers, a plant may face various types of problems in terms of system performance, stability and robustness.
A plant’s behavior can be defined by a set of IF-THEN fuzzy rules based on knowledge acquisition from knowledge based expert system, ANN and GA based algorithms. ANN and GA are used to learn fuzzy rules through structural identification which requires structural apriori knowledge such as linear or nonlinear control of a system. Another approach is parameter identification, scaling and normalization of physical signals. Another important feature is universal approximation: a fuzzy system with IF-THEN rules firing, defuzzification and membership function can approximate any real continuous function with certain approximation error due to the overlap of membership function from IF parts of a set of fuzzy rules. The inputs to the fuzzy controller are fuzzy values; they are quantitative numbers being obtained from different sources. For example, the intensity of a signal with respect to time interval is expressed by a membership function. FCs are able to deal with fuzzy value through heuristics or model based approaches.
The objective of fuzzy control is to design a feedback control law in the form of a set of fuzzy rules such that the closed loop system exhibits the desired behavior of a given model of the system and its desired behavior. The quality of nonlinear control is measured in terms of system performance, stability, robustness, accuracy and response speed In case of stabilization, the state vector of a closed loop system should be stabilized around a set point of the state space. Here, the challenge is to define a set of fuzzy rules based control law. In case of tracking, a FC is to be designed so that the output of closed loop system follows a time varying trajectory. The basic objective is to find a control law in terms of a set of fuzzy rules such that the tracking error [x(t) – xd(t)] tends to zero. In fact, stabilization is a special type of tracking where the desired trajectory is constant.
Next, let us consider system performance of a plant in terms of computing and application schema at level L3. In case of linear control, the behavior of a closed loop system can be specified in exact quantitative terms such as rise time, setting time, overshoot and undershoot. The desired behavior of a non-linear closed system can be specified in qualitative terms such as stability, accuracy, response speed and robustness. In case of linear control, stability implies the ability to withstand bounded disturbances in linear range of operation of the system. In case of nonlinear control, the behavior of the system is analyzed in terms of effects of positive disturbances and robustness. Robustness is the sensitivity of the closed loop system to various types of effects such as measurement noise, disturbances and unmodelled dynamics. The closed loop system must be insensitive to these effects. Accuracy and response speed must be considered for desired trajectories in the region of operation. Moreover, it is important to verify the plant’s performance in terms of reliability, consistency, resiliency, liveness, denial of service (DoS) attack, deadlock freeness, synchronization and application integration. It is expected to minimize human error in plant’s operation from the perspectives of correct decision making and adjustment of the setting of plant’s parameters.
The verification of correctness of computing schema of a fuzzy controller is a critical issue. A fuzzy logic controller defines a control law in terms of a transfer element due to non-linear nature of computation. The fuzzy control law is expressed by a set of ‘IF THEN’ fuzzy rules. ‘IF’ part of a fuzzy rule describes a fuzzy region in the state space. ‘THEN’ part specifies a control law applicable within fuzzy region from IF part of the same rule. FC yields a smooth non-linear control law through the operation of aggregation and defuzzification. The correctness of computing schema is associated with a set of computational steps such as input scaling or normalization, fuzzification of controller input variables, inference rule firing, defuzzification of controller output variables and output scaling or denormalization.
In case of supervisory control, one or more controllers are supervised by a control law on a higher level. The low level controllers perform a specific task under certain conditions keeping a predefined error between desired state and current state, performing a specific control task and being at a specific location of the state space. Supervision is required only if some of the predefined conditions fail : change the set of control parameters or switches from one control strategy to another. Supervisory algorithms are formulated in terms of IF-THEN rules. Fuzzy IF-THEN rules support soft supervision and avoid hard switching between set of parameters or between control structures.
In case of adaptive control, a dynamic system may have a known structure, but uncertain or slowly varying non-linear parameters. Direct adaptive approaches start with sufficient knowledge about the system structure and its parameters. Direct change of controller parameters optimize the system's behavior with respect to a given criterion. Indirect adaptive control methods estimate the uncertain parameters of the system under control on-line and use the estimated parameters in the computation of the control law. Adaptive control audits system performance in terms of stability, robustness, tracking convergence, tuning and optimization of various parameters like scaling factors for input and output signals, input and output membership functions and fuzzy IF-THEN rules.
It is also essential to audit security intelligence of the networking schema of the plant at level L4: detect threats of internal and external attacks such as cyber, rubber hose attack, sybil, node replication, wormhole, coremelt, forward, blackhole, neighbor, jellyfish and Crypto jacking attack. A real-time monitoring system must audit multi-party corruption at level L5 [e.g. sender, receiver, data, communication channel, mechanism, protocol, process, procedure]. The system administrator should mitigate the risks of various threats through proactive, reactive approaches and sense-and-response against bad luck like the occurrence of natural disaster.
Strategy 4 : SCADA / ICS requires both automated and semi-automated verification mechanisms for intrusion detection.
SCADA / ICS calls threat analytics and a set of model checking algorithms for various phases : exploratory phase for locating errors, fault finding phase through cause effect analysis, diagnostics tool for program model checking and real-time system verification. Model checking is basically the process of automated verification of the properties of the system under consideration. Given a formal model of a system and property specification in some form of computational logic, the task is to validate whether or not the specification is satisfied in the model. If not, the model checker returns a counter example for the system’s flawed behavior to support the debugging of the system. Another important aspect is to check whether or not a knowledge based system is consistent or contains anomalies through a set of diagnostics tools.
There are two different phases : explanatory phase to locate errors and fault finding phase to look for short error trails. Model checking is an efficient verification technique for communication protocol validation, embedded system, software programmers’, workflow analysis and schedule check. The basic objective of the model checking algorithm is to locate errors in a system efficiently. If an error is found, the model checker produces a counter example how the errors occur for debugging of the system. A counter example may be the execution of the system i.e. a path or tree. A model checker is expected to find out error states efficiently and produce a simple counterexample. There are two primary approaches of model checking: symbolic and explicit state. Symbolic model checking applies a symbolic representation of the state set for property validation. Explicit state approach searches the global state of a system by a transition function. The efficiency of model checking algorithms is measured in terms of automation and error reporting capabilities. The computational intelligence is also associated with the complexity of threat analytics equipped with the features of data visualization and performance measurement.
The threat analytics analyze system performance, sensitivity, trends, exception and alerts along two dimensions: time and insights. The analysis on time dimension may be as follows: what is corrupted or compromised in the system: agents, communication schema, data schema, application schema, computing schema and protocol? what occurred? what is occuring? what will occur? Assess probability of occurrence and impact. The analysis on insights may be as follows : how and why did the threat occur? What is the output of cause-effect analysis? The analytics also recommends what is the next best action? It predicts what is the best or worst that can happen?
How can you assess the immunity of ICS against intrusion in the form of sybil, cloning, wormhole or node capture attacks? Traditional intrusion detection techniques may not be able to sense danger signal or perform negative or clonal selection due to non-availability of intelligent threat analytics and ill-defined system immunity. The verification algorithm is expected to detect, assess and mitigate the risks of intrusion attacks more efficiently as compared to traditional approaches since it has a broad vision and adopts a set of AI moves including multi-dimensional view of intelligent reasoning, private search for evidence by balancing breadth and depth optimally and exploring system immunity combinatorially. Another interesting strategy is the use of a rational threat analytics.
Possible functionalities, constraints like computational and communication complexities and systematic features influence the perception of security and trust of a distributed network. For example, the computational power, memory capacity and energy li