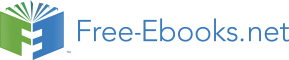

System Analytics
Agents: Earth scientists, environmental engineers;
Moves : requirements engineering, system design, prototype testing, erection, installation, testing, commissioning;
Emerging technologies: innovate a set of emerging technologies based on threats.
Drought and heatwave : Earth science – artificial rainfall, cloud seeding using laser technology and cloud physics; civil – water storage system, irrigation system ; mechanical – air conditioining system, air coolers, water pipeline; electrical – solar water pumps; logistics engineering – rail, ship, truck;
Flood / heavy rainfall : Earth science – cloud diversion; civil – dams, water conservation system, storage, drainage and irrigation system; mechanical – boats, helicopters, drones, planes; electrical – motors and pumps;
Snowfall : mechanical – excavators, ice breakers; electrical – room heaters;
Storm / cyclone / sandstorm : Earth science - predictive analytics; civil – robust housing structure; mechanical - excavator, electrical - fault analytics, power cut, standalone roof top solar panel;
Earthquake : Earth science – predictive analytics, geographical information system ; civil - earthquake proof light weight, flexible housing structure; mechanical and metallurgical – steel, aluminium, glass; electrical – protection from open circuit, short circuit, transients and power cut;
Volcano : Earth science – predictive analytics; logistics system – evacuation system, rail cars, bus, trucks, planes, helicopters; civil : safety chambers;
Bushfire : mechanical – water piepine, electrical – solar water pumps,lightning arrester; chemical – smoke, fire extinguishing system;
Epidemic / pandemic: Pharmaceutical engineering – vaccines, precision medicines, healthcare engineering, biotechnology engineering;
Astronomical hazards : Earth science and digital technology - predictive analytics, real-time moving target search, satellite communication; civil – safety shelter, cave, robust housing structure; mechanical – strong, reliable materials; electrical - fault analytics; chemical – fire extinguishing system;
Environmental pollution : environmental engineering (air, water, soil, sound, sunlight); civil – soil erosion control, restriction on soil digging, drainage system, nano housing architecture; mechanical – smoke emission control from vehicles, waste treatment plants;
Attacks of wild beasts and pastes : conservation of forests; civil - wall; mechsanical, rubber bullet, electrical - fence; chemical - gas bombs; digital / electronics – music system;
Prof. Bob Roy and Dr. Hariharan are discussing on the system associated with emerging technologies against various types of natural disaster, Natural rainfall requires conservation of forests and green plantation in smart cities and villages and along riverside. It is essential to save the rivers. Massive cut of trees and green plants in urban zone occurs due to construction of residential flats and other civil infrastructure (e.g. flyovers, bridges and transportation infrastructure). It may lead to droughts. The basic objectives of rainmaking or artificial precipitation or artificial rainfall is to artificially induce or increase precipitation using airplanes or rockets to sow to the clouds with catalysts. This method makes rain or increase precipitation and drought like situation.
Figure 2.1: Cloud Seeding
Cloud seeding is a weather modification method by dispersing substances into the air ; results condensation of cloud and alter the microphysical processes within the cloud [6-10]. The most common chemicals used for cloud seeding are salt powder (e.g. silver iodide, potassium iodide), dry ice and liquid propane. Cloud seeding chemicals may be dispersed by aircraft or drones or by dispersion devices located on the ground (e.g. firing from anti-aircraft guns or rockets) (Figure 2.1).
Glaciogenic cloud seeding use glaciogenic materials such as Silver Iodide which increase the ice crystal concentration in clouds by freezing cloud droplets. Static cloud seeding is applicable to cold cloud. Dynamic seeding results increased rainfall as compared to the static approach. The seeding of super cooled cloud with large amount of ice nuclei cause glaciation of the cloud. The super cooled liquid water is converted into ice flakes releasing latent heat, increasing buoyancy, growing larger and increased precipitation. Hygroscopic cloud seeding is a form of warm cloud seeding which enhances rainfall through coalescence process using fine spray of hygroscopic salt.
The other emerging techniques for artificial rainfall are laser induced cloud generation and ion generation method. Lasers may help causing rain; rainclouds form when airborne pockets of tiny particles condense water vapor around them (Figure 2.2). It is possible to control over moisture using laser. Weather control may get their next rainmaking tool in the form of an infrared laser. Precipitation is formed after lightning and heavy rain follows due to dissociation, ionization and natural seeding process in the atmosphere. Plasma laser pulse can be used for artificial rain making; for example 2.2 x 1019 gm of water drops are formed in the atmosphere by laser pulse of energy 500 mJ. Plasma laser pulse creates high temperature (up to 3000oC) which breaks bonds N2 and O2 into excited and unstable N* and O* and form NO and O3. These endothermic reactions absorb heat from the clouds and condensation creates water drops. Simultaneously N2 and O2 will be ionized and become big clustered ions through several
Figure 2.2 : Laser induced artificial rain
reaction. The big ions act as seed and results precipitation and rain.
Self-guided filaments generated by ultra short laser pulses is an emerging technology to assist water condensation, in an under saturated free atmosphere based on photo-oxidative chemistry and electrostatic effects. The phenomenon is used for remote characterization of humid atmosphere, triggering of water precipitation and cloud formation. Laser based condensation is a remote sensing nucleation processes in clouds influencing or triggering water precipitation. Self- guided laser filaments result from a nonlinear propagation regime of ultra-short laser pulses. Beyond a critical power (Pcr = 3 GW in air at a wavelength of 800 nm), the beam self-focuses due to the optical Kerr effect until its intensity is sufficient to allow multi-photon ionization of air molecules generating a cold plasma. At this point, the released free electrons (typically 1015–1016 cm) and the negative higher- order Kerr terms tend to defocus the beam and dynamically balance Kerr self- focusing. one or several self-guided filaments with a diameter of 100 m are generated over distances much longer than the Rayleigh length, up to hundreds of meters. Filaments can be initiated at predefined remote distances and propagate through adverse conditions including fog and clouds, turbulence or reduced pressures.
Test case : Private real-time moving target search for astronomical hazards Real-Time Probabilistic Search Mechanism (RPSM)
Input: search space, goal state, target distribution, detection function;
Output: Identify objects (e.g. moving targets);
Critical parameters : Target goal state, search space, detection function
Moves:
Procedure (Probabilistic Search):
Divide the search space into a set of private blocks;
Assign resources to each private block;
Project light beam on private search space move forward and backward;
Search discrete or continuous search space sense data stream
filter data stream;
Detect target verify correctness
give alert.
Security measures : (a) Proactive (b) Reactive.
The aforesaid mechanism (RPSM) is defined by a set of elements: system, searching agents, a finite set of inputs, a finite set of outcomes as defined by output function, a set of objective functions and constraints, an optimal set of moves, revelation principle, security measures and search procedure. It evaluates a system which is defined by a set of states (e.g. initial, goal, local and global) and state transition relations. The mechanism seeks the support of an intelligent reasoning system i.e. threat analytics.
Private Light Beam Search Algorithm : Let us analyze the aforesaid private search algorithm which is basically an interactive search. The cost of computation of probabilistic search depends on light beam projection on private search space. Let us show an illustration of private search. The basic steps of an interactive search algorithm which operates between a decision making agent (DMA) and the mediator agent (MA) are as follows: (a) MA computes an initial feasible solution. (b) MA interacts with the DMA and (c) MA obtains a (or a set of) new solution. If the new solution or one of the previous solutions is acceptable to the DMA, stop. Otherwise, go to step 2. The design of interactive search methods depends on various issues: (a) The form through which DMA gives information, (b) The approach by which a multi-objective problem is transformed into a single objective problem, (c) The type of data used for interaction with DMA, (d) Number of non- dominated points to be presented to the DMA (a single point or a sample of points) and (e) How the DMA evaluates a set of alternatives?
Agents : A decision-making agent (DMA) and the mediator agent (MA).
Input : The mediator holds the deterministic problem; The DMA holds its aspiration point, reservation point, indifferent threshold, strong and weak preference threshold and veto threshold.
Output: DMA knows a set of solutions; MA can not know the output.
1. MA requests the DMA to specify its preferential parameters ( PA,PR,Ith, Pth, Sth, Wth, Vth ).
2. The DMA generates (n-1) random set of preferential parameters and appends its desired set of preferential parameters at a random position. The DMA sends to MA the list H = (H1,…,Hn) where for a secret index 1j
n, Hj = ( PA, PR, Ith, Pth, Sth, Wth, Vth).
3. Repeat until the DMA is satisfied with a solution or concludes that no compromise point exists for the present constraints
a. MA computes a middle point (MP) alongwith characteristic neighbors for each set of preferential parameters.
b. The DMA gets back the results of k middle points alongwith characteristic neighbors using k-out-of-n oblivious transfer protocol where kn.; DMA scans the inner area of the current neighborhood and stores its preferred solutions in a private list L1; it stores the invalid middle points in a private list L2.
c. Case
1. The DMA wants to define a new aspiration and/or reservation point and/or updates preferential thresholds:
with characteristic neighborhood.
2. The DMA wants a point from the current neighborhood to be the new middle point or wants to return to one of the stored points of L1:
Let us consider a specific interactive search procedure called Light Beam Search (LBS) method The idea of light beam search is analogous to projecting a focused beam of light from the aspiration point onto the search space [Figure 2.3]. The lighted part of the frontier changes if the aspiration point or the point of interest in the non-dominated set is changed. This interactive search occurs between a DMA and the MA. The mediator asks the DMA to specify its preference in the form of aspiration and reservation point and various types of preferential thresholds. At each iteration of LBS procedure, MA generates a sample of non-dominated points using this preferential information. The sample is composed of a middle point and a set of non-dominated points from its neighborhood. MA shows these points to the decision-making agent.
Figure 2.3 : Light Beam Projection
Private light beam search preserves the privacy of individual preferential parameters of the decision making agents about the target goal in terms of aspiration point (PA), reservation point (PR), indifferent threshold (Ith), strong preference threshold (Sth), weak preference threshold (Wth), veto threshold (Vth), middle point (MP) and preferred solutions resulted from the search process. The mediator agent preserves the privacy of the search problem. The value of an objective function which is desirable or satisfactory to the decision maker is called aspiration point. The value of an objective function that the decision maker wants to avoid is called reservation point. A decision vector x*S is pareto optimal if there does not exist another decision vector x
S such that fi(x)
fi(x*) for all i =1,…,k and fj(x) <fj(x*) for at least one index j; fi is objective function and S is feasible space. An objective vector z*
Z is pareto optimal if there does not exist another objective vector z
Z such that zi
zi* for all i =1,…,k and zj < zj* for at least one index j.
The decision maker should inform the mediator various preference thresholds in order to compare alternatives and to define outranking relations. There is an interval of preference wherein it is not possible for the decision-making agent to distinguish between different alternatives due to imprecision and uncertainty of measurements and this corresponds to indifference threshold. Strict preference threshold is defined as minimal increase/decrease of any objective that makes the new alternative strictly preferred with respect to this objective. There exists an intermediate region between indifference and strict preference threshold where the decision-making agent hesitates to compare alternatives. This corresponds to weak preference threshold. Veto threshold It indicates that what is the minimal increase/decrease of any objective that makes the new alternative unacceptable regardless of the value of other objectives. In each computation phase of search, a finite sample of non-dominated points is generated by the mediator agent. The sample is composed of a middle point and a set of points within its neighborhood. The starting middle point is obtained by projecting aspiration point on the non- dominated set in the direction of reservation point. For a middle point, the neighborhood is defined as a set of non-dominated points that are not worse than the middle point. The neighborhood points from the sample indicate to what extent the values of particular objectives can be improved in relation to the middle point.
Test case : Private real-time moving target search algorithm for robotic navigation
The goal state of private search algorithm may be fixed or may change during the course of search. The goal may be a target which actively avoids the searching agent or object. In case of robot navigation In a moving target search algorithm, if the average speed of target robot is slower than that of the problem solving search agent, the later is guaranteed to eventually reach the target in a connected problem space. The algorithm is expected to be efficient in terms of minimum operations necessary to guarantee its completeness, commitment to reach the target goal and deliberation for selecting plan as per the principle of resource based planning. The target robot may be reaching the problem solving robot cooperatively or avoiding the same. The target robot may not stop eventually. The goal is achieved when the position of the problem solving robot and target robot coincide. If the problem solving robot moves faster than the target robot, the goal may be achieved. Otherwise, the target robot could evade the problem solving robot infinitely even in a finite problem solving space by avoiding in a dead end path.
Private Real-time Moving Target Search Mechanism
Agents / Objects : Problem solving agent(s), Target agent(s);
Scope :
System :
Structure : The search space consists of a set of nodes connected through edges. Security : Verify security intelligence of searching mechanism,
Access control : A or a set of authorized problem solving search agent(s) should be able to communicate and exchange data through authenticated channel and correctly identify target agent(s) in time.
Privacy in revelation principle: The problem solving agent(s) hide own speed and position from the target(s).
Audit rationality, commitment of trust of searching agents.
Verify fairness and correctness of heuristics estimate of distance, speed and position of the targets).
Asses the risks of corruption of searching agent(s) (e.g. problem solving robot).
Verify reliability, consistency, deadlock-freeness, reachability and resiliency of the search process.
Assess the risks of malicious attacks: Denial of service, false data injection and Sybil attack.
Strategy : Adaptive real-time private moving target search – depth first search, breadth first search or a combination of the two;
Resources : Allocate adequate resources for search and private communication.
Skill-style-support: Ensure transparency, accountability, collaboration, coordination and and resource based planning in private search.
Analysis of Private Search Algorithm : The efficiency of private moving target search is evaluated n terms of space complexity and time complexity. The upper bound on the space complexity of private moving target search is n2 where n is the number of states in the problem space. The overall space complexity is the minimum of n2 and the total number of moves of the problem solving search agent and target. The worst-case time complexity of private search is n3 where