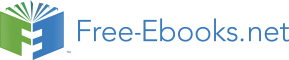

System Analytics
Agents: system analysts, business analysts, scientists;
Moves : requirements engineering, system design, coding, prototype testing, installation, testing, commissioning
Emerging technologies: Innovate a set of emerging digital technologies to ensure financial security such as anti-money laundering, fraud detection, proof of works, secure multi-party computation and financial analytics;
Prof. G. Nissim and Prof. Goldwasser are outlining the system associated with the technology of financial security. Financial analytics is an intelligent, complex, hybrid, multi-phased and multi-dimensional data analysis system. The basic steps of computation are data sourcing, data filtering / preprocessing, data ensembling, data analysis and knowledge discovery from data. The authorized data analysts select an optimal set of input variables, features and dimensions (e.g. scope, system, structure, security, strategy, staff-resources, skill-style-support) correctly being free from malicious attacks (e.g. false data injection, shilling); input data is sourced through authenticated channels accordingly. The sourced data is filtered, preprocessed (e.g. bagging, boosting, cross validation) and ensembled. It is rational to adopt an optimal mix of quantitative (e.g. regression, prediction, sequence, association, classification and clustering algorithms) and qualitative methods for multi-dimensional analysis. The analysts define intelligent training and testing strategies in terms of selection of correct soft computing tools, network architecture – no. of layers and nodes; training algorithm, learning rate, no. of training rounds, cross validation and stopping criteria. The hidden knowledge is discovered from data in terms of business intelligence. The analysts audit fairness and correctness of computation and also reliability, consistency, rationality, transparency and accountability of the analytics.
Financial analytics can process precisely targeted, complex and fast queries on large data sets of real-time and near real-time systems. Business analytics follows a systematic, streamlined and structured process that can extract, organize and analyze large amounts of data in a form being acceptable, useful and beneficial for an entity. It is basically a specific type of distributed computing across a number of server or nodes to speed up the analysis process. Generally, shallow analytics use the concept of means, standard deviation, variance, probability, proportions, pie charts, bar charts and tabs to analyze small data set. Business analytics analyze large data sets based on the concepts of data visualization, descriptive and prescriptive statistics, predictive modeling, machine learning, multilevel modeling, data reduction, multivariate analysis, regression analysis, logistic regression analysis, text analysis and data wrangling. Deep analytics is often coupled with business intelligence applications which perform query based search on large data, analyze, extract information from data sets hosted on a complex and distributed architecture and convert that information into specialized data visualization outcome such as reports, charts and graphs. Big data refers to large data being generated continuously in the form of unstructured, semi-structured and structured data produced by social network to scientific computing applications. The dataset may range from a few hundred gigabytes to terabytes beyond the capacity of existing data management tools that can capture, store, manage and analyze. Big data is characterized by volume, velocity, variety, variability, complexity and low value density. Capital market firms use big data technologies to mitigate risks for fraud mitigation, ondemand enterprise management, regulation, trading analysis and data tagging.