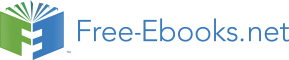

9000
10000
discrete time (n)
Fig. 8. Trajectories of μw of proposed AS-OSNALMS algorithm for TEQ using different
setting of μw and μ for TEQ and TIR with fixed at α
0
b 0
w = 4.45 × 10−4 and αb = 1.75 × 10−4,
when the samples of CSA loop are loop #1.
0.18
0.16
0.14
0.12
0.1
μ b
0.08
0.06
0.04
μ =0.0495, μ =0.015
w0
b0
0.02
μ =0.0750, μ =0.045
w0
b0
μ =0.0335, μ =0.0075
w0
b0
00
1000
2000
3000
4000
5000
6000
7000
8000
9000
10000
discrete time (n)
Fig. 9. Trajectories of μb of proposed AS-OSNALMS algorithm for TIR using different setting
of μw and μ for TEQ and TIR with fixed at α
0
b 0
w = 4.45 × 10−4 and αb = 1.75 × 10−4, when
the samples of CSA loop are loop #1.
402
Discrete Time Systems
0.5
μ =0.0415, α =4.45×10−4
w0
w
μ =0.4150, α =1.25×10−6
w0
w
0.4
0.3
μ w 0.2
0.1
0
−0.10
1000
2000
3000
4000
5000
6000
7000
8000
9000
10000
discrete time (n)
Fig. 10. Trajectories of μw of proposed AS-OSNALMS algorithm for TEQ using different
setting of μw and μ for TEQ and TIR with different at α
0
b 0
w = 4.45 × 10−4 and
αw = 1.25 × 10−6, when the samples of CSA loop are loop #1.
0.2
0.18
0.16
0.14
0.12
0.1
μ b
0.08
0.06
0.04
μ =0.0950, α =1.50x10−6
0.02
b0
b
μ =0.0095, α =1.75x10−4
b0
b
00
1000
2000
3000
4000
5000
6000
7000
8000
9000
10000
discrte time (n)
Fig. 11. Trajectories of μb of proposed AS-OSNALMS algorithm for TIR using different
setting of μw and μ for TEQ and TIR with different at α
0
b 0
b = 1.75 × 10−4 and
αb = 1.5 × 10−6, when the samples of CSA loop are loop #1.
Adaptive Step-size Order Statistic LMS-based
Time-domain Equalisation in Discrete Multitone Systems
403
9. References
Al-Dhahir, N. & Cioffi, J.M. (1996). Optimum Finite-Length Equalization for Multicarrier
Transceivers, IEEE Trans. on Comm. , vol. 44, no. 1, pp. 56-64, Jan. 1996.
Benveniste, A.; M ´ e tivier, M. & Priouret, P. (1990). Adaptive Algorithms and Stochastic
Approximations, Springer-Verlag.
Bladel, M.V. & Moeneclaey, M. (1995). Time-Domain Equalization for Multicarrier
Communication, Proceedings of IEEE Global Comm. Conf. (GLOBECOM), pp.167-171,
Nov. 1995.
Baldemair, R. & Frenger, P. (2001). A Time-domain Equalizer Minimizing Intersymbol and
Intercarrier Interference in DMT Systems, Proceedings of IEEE Global Comm. Conf.
(GLOBECOM), vol.1, pp.381-385, Nov. 2001.
Chambers, J.A. (1993). Normalization of Order Statistics LMS Adaptive Filters Sequential
Parameter Estimation, Schlumberger Cambridge Research, U.K., 1993.
Diniz, P.S.R. (2008) Adaptive Filtering Algorithms and Practical Implementation, Springer.
F-Boroujeny, B. & Ding, M. (2001). Design Methods for Time-Domain Equalizers in DMT
Transceivers, IEEE Trans. on Comm. , vol. 49, no. 3, pp. 554-562, Mar. 2001.
Golden, P.; Dedieu H. & Jacobsen, K.S. (2006). Fundamentals of DSL Technology, Auerbach
Publications, Taylor & Francis Group, New York.
Hayes, M.H. (1996). Statistical Digital Signal Processing and Modeling, John Wiley & Sons, 1996.
Haykin, S. (2002). Adaptive Filter Theory, Prentice Hall, Upper Saddle River, New Jersey.
Haweel, T.I. & Clarkson, P.M. (1992). A Class of Order Statistics LMS Algorithms, IEEE Trans.
on Signal Processing, vol.40, no.1, pp.44-53, 1992.
Henkel, W., Taub öck, G., Ödling, P.; B örjesson, P.O. & Petersson, N. (2002). The Cyclic Prefix of
OFDM/DMT-An Analysis, Proceedings of IEEE Int.Zurich Seminar on Broadband Comm.
Access-Transmission-Networking, pp. 22.1-22.3, Feb. 2002.
International Telecommunications Union (ITU) (2001). Recommendation G.996.1, Test
Procedures for Asymmetric Digital Subscriber Line (ADSL) Transceivers, February 2001.
International Telecommunications Union (ITU) (2002). Recommendation G.992.3, Asymmetric
Digital Subscriber Line (ADSL) Transceivers-2 (ADSL), July 2002.
International Telecommunications Union (ITU) (2003). Recommendation G.992.5, Asymmetric
Digital Subscriber Line (ADSL) Transceivers-Extened Bandwidth ADSL2 (ADSL2+), May
2003.
Kushner, H.J. & Yang, J. (1995). Analysis of Adaptive Step-Size SA Algorithms for Parameter
Tracking, IEEE Trans. on Automatic Control, vol. 40, no. 8, pp. 1403-1410, Aug. 1995.
Lee, I., Chow, J.S. & Cioffi, J.M. (1995). Performance evaluation of a fast computation algorithm
for the DMT in high-speed subscriber loop, IEEE J. on Selected Areas in Comm. , pp.
1564-1570, vol.13, Dec. 1995.
L ópez-Valcarce, R. (2004). Minimum Delay Spread TEQ Design in Multicarrier Systems, IEEE
Signal Processing Letters, vol. 11, no. 8, Aug. 2004.
Melsa, P.J.W., Younce, R.C. & Rohrs, C.E. (1996). Impulse Response Shortening for Discrete
Multitone Transceivers, IEEE Trans. on Communications, vol. 44, no. 12, pp. 1662-1672,
Dec. 1996.
Moon, T.K. & Stirling, W.C. (2000). Mathmatical Methods and Algorithms for Signal Processing,
Prentice Hall, Upper Saddle River, New Jersey.
Nafie, M. & Gather, A. (1997). Time-Domain Equalizer Training for ADSL, Proceedings of IEEE
Int. Conf. on Communications (ICC), pp.1085-1089, June 1997.
404
Discrete Time Systems
Sitjongsataporn, S. & Yuvapoositanon, P. (2007). An Adaptive Step-size Order Statistic Time
Domain Equaliser for Discrete Multitone Systems, Proceedings of IEEE Int. Symp. on
Circuits and Systems (ISCAS), pp. 1333-1336, New Orleans, LA., USA., May 2007.
Starr, T., Cioffi, J.M. & Silvermann, P.J. (1999) Understanding Digital Subscriber Line Technology,
Prentice Hall, New Jersey.
Wang, B. & Adali, T. (2000). Time-Domain Equalizer Design for Discrete Multitone Systems,
Proceedings of IEEE Int. Conf. on Communications (ICC), pp.1080-1084, June 2000.
Yap, K.S. & McCanny, J.V. (2002). Improved time-domain equalizer initialization algorithm for
ADSL modems, Proceedings of Int. Symp. on DSP for communication systems (DSPCS),
pp.253-258, Jan. 2002.
Ysebaert, G., Acker, K.Van, Moonen, M. & De Moor, B. (2003). Constraints in channel
shortening equalizer design for DMT-based systems, Signal Processing, vol. 83, no.
3, pp. 641-648, Mar. 2003.
23
Discrete-Time Dynamic
Image-Segmentation System
Ken’ichi Fujimoto, Mio Kobayashi and Tetsuya Yoshinaga
The University of Tokushima
Japan
1. Introduction
The modeling of oscillators and their dynamics has interested researchers in many fields such
as those in physics, chemistry, engineering, and biology. The Hodgkin-Huxley (Hodgkin
& Huxley, 1952) and Fitzhugh-Nagumo models (FitzHugh, 1961), which corresponds
to the Bonhöffer van der Pol (BvP) equation, are well-known models of biological
neurons. They have been described by differential equations, i.e., they are continuous-time
relaxation oscillators. Discrete-time oscillators, e.g., one consisting of a recurrent neural
network (Haschke & Steil, 2005) and another consisting of a spiking neuron model (Rulkov,
2002), have been proposed.
Synchronization observed in coupled oscillators has been established to be an important
topic (Pikovsky et al., 2003; Waller & Kapral, 1984).
Research on coupled oscillators
has involved studies on pattern formation (Kapral, 1985; Oppo & Kapral, 1986), image
segmentation (Shareef et al., 1999; Terman & Wang, 1995; Wang & Terman, 1995; 1997),
and scene analysis (Wang, 2005). Of these, a locally excitatory globally inhibitory oscillator
network (LEGION) (Wang & Terman, 1995), which is a continuous-time dynamical system,
has been spotlighted as an ingenious image-segmentation system. A LEGION can segment
an image and exhibit segmented images in a time series, i.e., it can spatially and temporally
segment an image. We call such processing dynamic image segmentation. A LEGION consists
of relaxation oscillators arranged in a two-dimensional (2D) grid and an inhibitor globally
connected to all oscillators and it can segment images according to the synchronization of
locally coupled oscillators. Image segmentation is the task of segmenting a given image so
that homogeneous image blocks are disjoined; it is a fundamental technique in computer
vision, e.g., object recognition for a computer-aided diagnosis system (Doi, 2007) in medical
imaging. The problem with image segmentation is still serious, and various frameworks have
been proposed (Pal & Pal, 1993; Suri et al., 2005) to solve this.
We proposed a discrete-time oscillator model consisting of a neuron (Fujimoto et al., 2008),
which was modified from a chaotic neuron model (Aihara, 1990; Aihara et al., 1990),
coupled with an inhibitor. Despite discrete-time dynamics as well as the recurrent neural
network (Haschke & Steil, 2005), a neuron in our oscillator can generate a similar oscillatory
response formed by a periodic point to an oscillation as observed in a continuous-time
relaxation oscillator model, e.g., the BvP equation. This is a key attribute in our idea.
Moreover, we proposed a neuronal network system consisting of our neurons (discrete-time
oscillators) arranged in a 2D grid and an inhibitor globally coupled to all neurons. As well as
406
Discrete Time Systems
a LEGION, our neuronal network system can work as a dynamic image-segmentation system
according to the oscillatory responses of neurons. Our system provides much faster dynamic
image segmentation than a LEGION on a digital computer because numerical integration is
not required (Fujimoto et al., 2008). Another advantage of our system is that it simplifies
the investigation of bifurcations of fixed points and periodic points due to the discrete-time
dynamical system. A fixed point and a periodic point correspond to non-oscillatory and
periodic oscillatory responses. Knowledge on the bifurcations of responses allows us to
directly design appropriate system parameters to dynamically segment images. The assigned
system parameters are made available by implementing our dynamic image-segmentation
system into hardware such as field-programmable gate array devices (Fujimoto et al., 2011b).
This article describes the derivation of a model reduced from our dynamic
image-segmentation system that can simplify bifurcation analysis. We also explain our
method of bifurcation analysis based on dynamical systems theory. Through analysis in
reduced models with two or three neurons using our method of analysis, we find parameter
regions where a fixed point or a periodic point exists.
We also demonstrate that our
dynamic image-segmentation system, whose system parameters were appropriately assigned
according to the analyzed results, can work for images with two or three image regions.
To demonstrate that segmentation is not limited to three in the system, we also present a
successive algorithm for segmenting an image with an arbitrary number of image regions
using our dynamic image-segmentation system.
2. Discrete-time dynamic image-segmentation system
2.1 Single neuronal system
Figure 1(a) illustrates the architecture of a system consisting of a neuron (Fujimoto et al., 2008)
and an inhibitor. Here, let us call it a single neuronal system. Our neuron model modified from
a chaotic neuron model (Aihara, 1990; Aihara et al., 1990) has two internal state variables, x
and y; z corresponds to the internal state variable of an inhibitor, in which x, y, z ∈ R with R
denoting the set of real numbers. Let the sum of internal state values in a neuron, i.e. x + y,
be the activity level of a neuron. The dynamics of the single neuronal system is described by
difference equations:
x( t + 1) = k f x( t) + d + Wx · g( x( t) + y( t), θc) − Wz · g( z( t), θz) (1a)
y( t + 1) = kry( t) − α · g( x( t) + y( t), θc) + a
(1b)
z( t + 1) = φ g g( x( t) + y( t), θ f ), θd − z( t) .
(1c)
The t ∈ Z denotes the discrete time where Z expresses the set of integers. g( ·, ·) is the output
function of a neuron or an inhibitor and is described as
g( u( t), θ) =
1
1 + exp( −( u( t) − θ)/ ε) .
(2)
Note that g( ·, θd) where g( x( t) + y( t), θ f ) is nested in Eq. (1c) is neither output function, but a function to find the firing of a neuron that corresponds to a high level of activity. Therefore, an
inhibitor plays roles in detecting a fired neuron and suppressing the activity level of a neuron
at the next discrete time. The k f , kr, and φ are coefficients corresponding to the gradient of x,
y, and z. The d denotes an external direct-current (DC) input. The Wx and α are self-feedback
gains in a neuron, and Wz is the coupling coefficient from an inhibitor to a neuron. The a is a
Discrete-Time Dynamic Image-Segmentation System
407
bias term in a neuron. The θc and θz are threshold parameters in output functions of a neuron
and an inhibitor, respectively. Also, θ f and θd are threshold parameters to define the firing of
a neuron and to detect a fired neuron, respectively. The ε is a parameter that determines the
gradient of the sigmoid function (2) at u( t) = θ.
When we set all the parameters to certain values, our neuron can generate a similar oscillatory
response formed by a periodic point to an oscillation as observed in a continuous-time
relaxation oscillator model. For instance, the time evolution of a generated response, in
which this is a waveform, is shown in Fig. 1(b) for initial values, ( x(0), y(0), z(0)) =
(32.108, − 31.626, 0.222), at k f = 0.5, d = 2, Wx = 15, θc = 0, Wz = 15, θz = 0.5, kr = 0.89, α = 4, a = 0.5, φ = 0.8, θf = 15, θd = 0, and ε = 0.1. To clarify the effect of the inhibitor,
we have shown the activity level of the neuron and the internal state of the inhibitor on the
vertical axis in this figure. The points marked with open circles “◦” indicate the values of
x + y and z at discrete time t. Although the response of a neuron or an inhibitor is formed
by a series of points because of its discrete-time dynamics, we drew lines between temporally
adjacent points as a visual aid. Therefore, our neuron coupled with an inhibitor is available as
a discrete-time oscillator.
20
10
−→
Neuron
0
y+ -10
x
(
-20
x, y)
0
20
40
60
80
100
1
0.8
0.6
−→ 0.4
z
z 0.2
00
20
40
60
80
100
Inhibitor
t −→
(a) Architecture
(b) Oscillatory response
Fig. 1. Architecture of single neuronal system and generated oscillatory response
2.2 Neuronal network system
We have proposed a neuronal network system for dynamic image segmentation (Fujimoto
et al., 2008). Figure 2(a) outlines the architecture of our system for a 2D image with P
pixels. It is composed of our neurons that have as many pixels as in a given image and
an inhibitor that is called a global inhibitor because it is connected with all neurons. All
neurons are arranged in a 2D grid so that one corresponds to a pixel, and a neuron can have
excitatory connections to its neighboring neurons. Here, we assumed that a neuron could
connect to its four-neighboring ones. The formation of local connections between neighboring
neurons is determined according to the value of DC input to each neuron. Note that, we
can use our neuronal network system, in which neurons are arranged in a 3D grid so that
one neuron corresponds to a voxel, which means a volumetric picture element, as a dynamic
image-segmentation system for a 3D image.
The architecture for the i th neuron in a neuronal network system is illustrated in Fig. 2(b).
The open and closed circles at the ends of the arrows correspond to excitatory and inhibitory
408
Discrete Time Systems
N1
N +1
N P− +1
N2
N +2
N P− +2
N
N2
N P
N : modified chaotic neuron
GI
GI: global inhibitor
(a) Neuronal network system
External input
g( xk + yk, θc)
Wx/ Mi
g( z, θz)
Wz
di
Output
N i
g( xi + yi, θc)
Wx/ Mi
Self-feedback
α
xi, yi : Internal States
(b) The i th neuron
Fig. 2. Architecture of neuronal network system and a neuron
couplings. A neuron can receive external inputs from neighboring ones connected to it. An
external input from another neuron can induce in-phase synchron