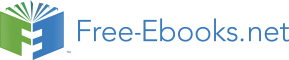

In the algorithm described above
we encountered expressions
of the form A1⊗A2⊗⋯⊗An
which we denote by
To calculate the product (⊗iAi)x
it is computationally advantageous to factor
⊗iAi into terms of the form
I⊗Ai⊗I1.
Then each term represents a set of copies of Ai.
First, recall the following property of Kronecker products
This property can be used to factor ⊗iAi in the following way. Let the number of rows and columns of Ai be denoted by ri and ci respectively. Then
But we can also write
Note that in factorization Equation 5.2, copies of A2 are applied to the data vector x first, followed by copies of A1. On the other hand, in factorization Equation 5.3, copies of A1 are applied to the data vector x first, followed by copies of A2. These two factorizations can be distinguished by the sequence in which A1 and A2 are ordered.
Lets compare the computational complexity of factorizations Equation 5.2 and Equation 5.3. Notice that Equation 5.2 consists of r2 copies of A1 and c1 copies of A2, therefore Equation 5.2 has a computational cost of r2Q1+c1Q2 where Qi is the computational cost of Ai. On the other hand, the computational cost of Equation 5.3 is c2Q1+r1Q2. That is, the factorizations Equation 5.2 and Equation 5.3 have in general different computational costs when Ai are not square. Further, observe that Equation 5.2 is the more efficient factorization exactly when
or equivalently, when
Consequently,
in the more efficient factorization,
the operation Ai applied to the data vector x first is the one
for which the ratio is the
more negative.
If r1>c1 and r2<c2
then Equation 5.4 is always true (Qi is always positive).
Therefore, in the most computationally efficient
factorization of A1⊗A2, matrices with fewer rows than
columns are always applied to the data vector x before
matrices with more rows than columns.
If both matrices are square, then their ordering does not
affect the computational efficiency, because
in that case
each ordering has the same computation cost.
We now consider the Kronecker product of more than two matrices. For the Kronecker product ⊗ni=1Ai there are n! possible different ways in which to order the operations Ai. For example
Each factorization of ⊗iAi can be described by a permutation g(·) of {1,...,n} which gives the order in which Ai is applied to the data vector x. Ag(1) is the first operation applied to the data vector x, Ag(2) is the second, and so on. For example, the factorization Equation 5.6 is described by the permutation g(1)=3, g(2)=1, g(3)=2. For n=3, the computational cost of each factorization can be written as
In general
Therefore, the most efficient factorization of ⊗iAi is described by the permutation g(·) that minimizes C.
It turns out that for the Kronecker product of more than two
matrices, the ordering of operations that describes
the most efficient factorization of ⊗iAi
also depends only on the ratios .
To show that this is the case, suppose
u(·) is the permutation that
minimizes C,
then u(·) has the property that
for k=1,⋯,n–1. To support this, note that since u(·) is the permutation that minimizes C, we have in particular
where v(·) is the permutation defined by the following:
Because only two terms in Equation 5.8 are different, we have from Equation 5.10
which, after canceling common terms from each side, gives
Since v(k)=u(k+1) and v(k+1)=u(k) this becomes
which is equivalent to Equation 5.9.
Therefore, to find the best factorization of ⊗iAi
it is necessary only to compute the ratios
and to order them in an non-decreasing order.
The operation Ai whose index appears first in this list
is applied to the data vector x first, and so on
As above, if ru(k+1)>cu(k+1) and ru(k)<cu(k) then Equation 5.14 is always true. Therefore, in the most computationally efficient factorization of ⊗iAi, all matrices with fewer rows than columns are always applied to the data vector x before any matrices with more rows than columns. If some matrices are square, then their ordering does not affect the computational efficiency as long as they are applied after all matrices with fewer rows than columns and before all matrices with more rows than columns.
Once the permutation g(·) that minimizes C
is determined by ordering the ratios
,
⊗iAi can be written as
where
and where γ(·) is defined by
A Matlab program that computes the permutation
that describes the computationally most efficient
factorization of ⊗ni=1Ai is cgc()
.
It also gives the resulting computational cost.
It requires the computational cost of each of the
matrices Ai and the number of rows and columns
of each.
function [g,C] = cgc(Q,r,c,n) % [g,C] = cgc(Q,r,c,n); % Compute g and C % g : permutation that minimizes C % C : computational cost of Kronecker product of A(1),...,A(n) % Q : computation cost of A(i) % r : rows of A(i) % c : columns of A(i) % n : number of terms f = find(Q==0); Q(f) = eps * ones(size(Q(f))); Q = Q(:); r = r(:); c = c(:); [s,g] = sort((r-c)./Q); C = 0; for i = 1:n C = C + prod(r(g(1:i-1)))*Q(g(i))*prod(c(g(i+1:n))); end C = round(C);
The Matlab program kpi()
implements the Kronecker
product ⊗ni=1Ai.
function y = kpi(d,g,r,c,n,x) % y = kpi(d,g,r,c,n,x); % Kronecker Product : A(d(1)) kron ... kron A(d(n)) % g : permutation of 1,...,n % r : [r(1),...,r(n)] % c : [c(1),..,c(n)] % r(i) : rows of A(d(i)) % c(i) : columns of A(d(i)) % n : number of terms for i = 1:n a = 1; for k = 1:(g(i)-1) if i > find(g==k) a = a * r(k); else a = a * c(k); end end b = 1; for k = (g(i)+1):n if i > find(g==k) b = b * r(k); else b = b * c(k); end end % y = (I(a) kron A(d(g(i))) kron I(b)) * x; y = IAI(d(g(i)),a,b,x); end
where the last line of code
calls a function that implements .
That is, the program
IAI(i,a,b,x)
implements
.
The Matlab program IAI
implements
function y = IAI(A,r,c,m,n,x) % y = (I(m) kron A kron I(n))x % r : number of rows of A % c : number of columns of A v = 0:n:n*(r-1); u = 0:n:n*(c-1); for i = 0:m-1 for j = 0:n-1 y(v+i*r*n+j+1) = A * x(u+i*c*n+j+1); end end
It simply uses two loops to implement the mn copies of A. Each copy of A is applied to a different subset of the elements of x.